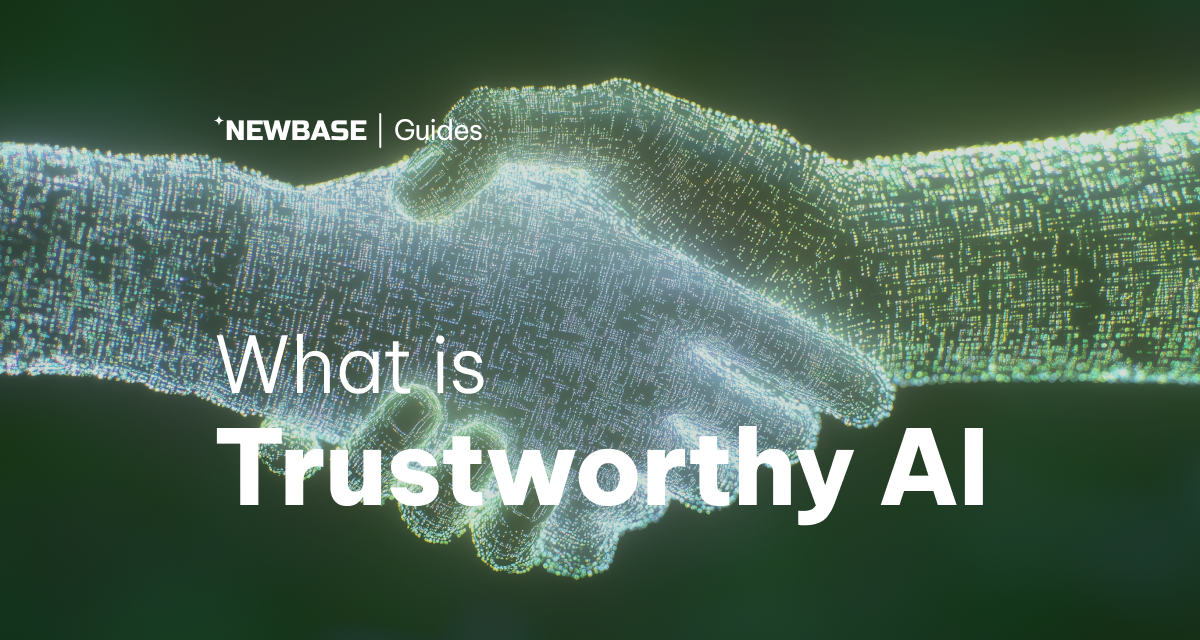
Trust is paramount in any relationship. And as Artificial Intelligence (AI) becomes increasingly integrated into our lives, building trust in these systems is crucial. This is where the concept of Trustworthy AI comes in.
Why Trustworthy AI Matters
Many AI systems operate like black boxes, generating outputs with little transparency into their decision-making processes. This lack of explainability can fuel distrust, hindering both individual and organizational adoption of AI technologies.
Real-world examples highlight the potential pitfalls of untrustworthy AI:
- Biased algorithms: Algorithmic bias can lead to unfair outcomes, such as discriminatory loan approvals or hiring practices.
- Inaccurate outputs: Medical AI models failing to diagnose patients correctly or financial models providing misleading investment advice.
- Security vulnerabilities: Hackers exploiting vulnerabilities in AI models to steal sensitive information.
Trustworthy AI aims to mitigate these risks by ensuring AI systems are:
- Explainable: Users can understand how AI models arrive at their outputs, fostering trust and confidence.
- Fair: Mitigating bias in data and algorithms to ensure equitable treatment of individuals and groups.
- Transparent: Openness about the architecture, features, and data used in AI models.
- Secure: Robust security measures to protect against unauthorized access and cyberattacks.
The Principles of Trustworthy AI
Several key principles guide the development of trustworthy AI:
- Accountability: Holding individuals and organizations responsible for the proper functioning of AI systems throughout their lifecycles.
- Fairness: Ensuring unbiased decision-making, addressing algorithmic and data biases.
- Interpretability: Understanding how AI models arrive at their results for better human oversight.
- Privacy: Protecting personal information collected, used, shared, or stored by AI systems.
- Reliability: Consistent and reliable performance under expected conditions.
- Robustness and Security: Resilience against adversarial attacks and unauthorized access.
- Safety: Minimizing the risk of harm to humans, property, and the environment.
These principles not only mitigate risks but also unlock the full potential of AI. By ensuring reliable, accurate, and ethical applications, trustworthy AI paves the way for responsible development and adoption.
Frameworks for Trustworthy AI
Several frameworks offer guidance for building trustworthy AI systems:
- The National Institute of Standards and Technology (NIST) AI Risk Management Framework (AI RMF): Provides a roadmap for assessing and managing AI risks across the entire AI lifecycle.
- The Organisation for Economic Co-operation and Development (OECD) AI Principles: Focus on human rights and democratic values, emphasizing responsible innovation in AI development.
- The EU’s Ethics Guidelines for Trustworthy Artificial Intelligence: A human-centric approach stressing fairness, transparency, and accountability in AI practices within the European Union.
These frameworks offer valuable tools for organizations to develop and deploy AI in a responsible and trustworthy manner.
Trustworthy AI vs. Ethical AI vs. Responsible AI
These terms often overlap, but subtle distinctions exist:
- Trustworthy AI: Emphasizes earning trust through the characteristics mentioned above.
- Ethical AI: Focuses on designing and developing AI systems with consideration for human values and morals.
- Responsible AI: Encompasses the practical methods of integrating ethical principles into AI applications and workflows.
Ultimately, these concepts work together to build a foundation for trustworthy AI systems.
Achieving Trustworthy AI
Organizations can implement various strategies to achieve trustworthy AI:
- Assessment: Evaluating AI-enabled processes to identify areas for improvement regarding trustworthiness metrics.
- Continuous Monitoring: Proactively identify and address potential biases, model drift, or security vulnerabilities.
- Risk Management: Establish frameworks and tools to minimize security risks and protect user privacy.
- Documentation: Maintain detailed records of data science and AI processes to ensure accountability and transparency.
- AI Governance: Implement frameworks and tools to ensure developers and data scientists adhere to internal standards and regulations.
By adopting these strategies and embracing the principles of trustworthy AI, organizations can minimize risks and maximize the positive impact of AI on society.