What is Text Generation? How AI Transforms Words into Meaningful Content
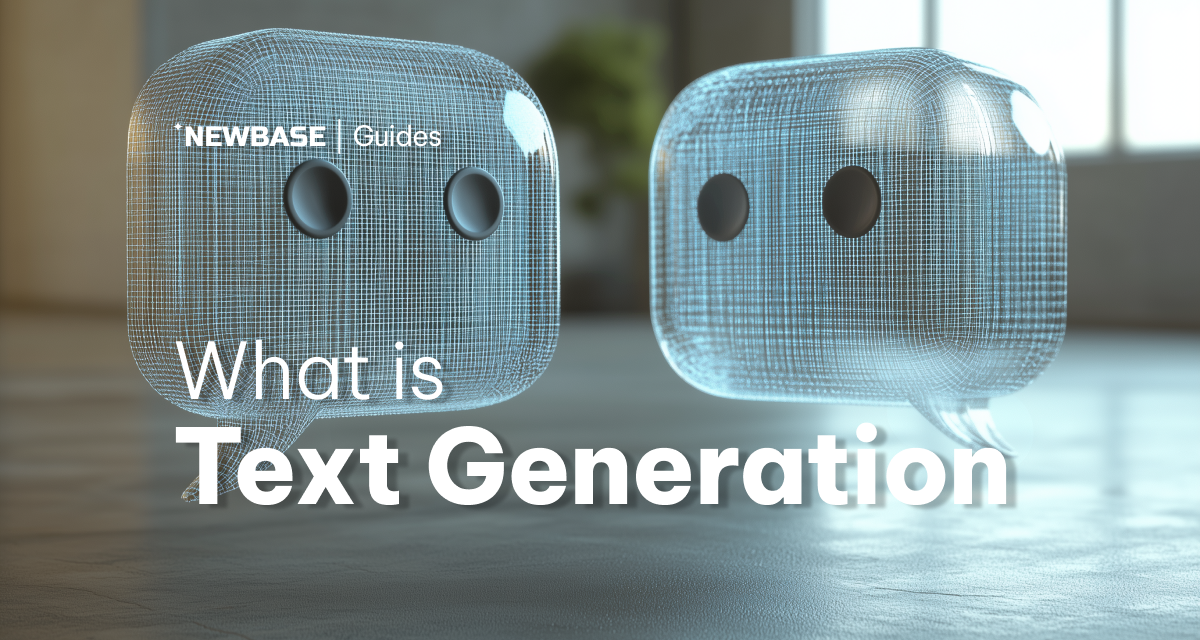
Text generation is the process of automatically creating coherent, meaningful, and contextually appropriate text. This can include anything from single sentences to entire documents. It relies on techniques from fields such as natural language processing (NLP), machine learning (ML), and deep learning to analyze input data and produce human-like text. The primary objective is to ensure that the generated text is not only grammatically accurate but also engaging and relevant to the intended audience.
A Brief History of Text Generation
The concept of text generation has its roots in the early days of computer science, dating back to the 1950s and 1960s. Early approaches were rule-based, relying heavily on pre-defined grammatical structures. The field saw significant advancements in the 1980s and 1990s with the emergence of machine learning techniques. However, it was the advent of deep learning and neural networks in the 21st century that truly revolutionized text generation, enabling more sophisticated and human-like outputs.
Key Concepts in Text Generation
Natural Language Understanding (NLU) vs. Natural Language Generation (NLG)
- Natural Language Understanding (NLU): Focuses on enabling machines to comprehend, interpret, and extract meaningful information from human language. Tasks include sentiment analysis, named entity recognition, and intent detection.
- Natural Language Generation (NLG): Concerned with producing human-like text. Applications include text summarization, storytelling, and dialogue systems.
While NLU aims to understand human language, NLG focuses on generating it. Together, they form the backbone of advanced NLP systems.
Benefits of Text Generation
- Improved Efficiency: Automates content creation, saving time and resources. For example, AI can draft product descriptions or social media posts at scale.
- Enhanced Creativity: AI-generated text can provide unique ideas, overcoming creative blocks for writers and artists.
- Increased Accessibility: Generates text in multiple languages or formats, aiding individuals with disabilities or language barriers.
- Personalized Communication: Tailors content to individual preferences, improving customer engagement.
- Support for Education: Assists language learners by providing constructive feedback and practice material.
Challenges in Text Generation
Despite its advancements, text generation faces several challenges:
- Quality Assurance: Ensuring the generated text is coherent, accurate, and contextually appropriate.
- Diversity: Balancing consistency with the need for varied and creative outputs.
- Ethical Concerns: Addressing issues like bias in training data and the potential misuse of generated content.
- Privacy Risks: Safeguarding sensitive information during the training and deployment of models.
Techniques in Text Generation
Statistical Models
- N-gram Models: Predict the probability of a sequence of words based on fixed-size word groupings.
- Conditional Random Fields (CRFs): Utilize probabilistic graphical models to generate contextually relevant text.
Neural Networks
- Recurrent Neural Networks (RNNs): Specialized for sequential data but struggle with long-term dependencies.
- Long Short-Term Memory Networks (LSTMs): Handle longer sequences by incorporating memory cells.
Transformer-Based Models
- GPT (Generative Pre-trained Transformer): Excels at generating diverse and coherent text by leveraging large-scale pretraining.
- BERT (Bidirectional Encoder Representations from Transformers): Focuses on context understanding, making it ideal for nuanced text generation.
Applications of Text Generation
Text generation has diverse applications across industries:
- Content Creation: Automated generation of blog posts, articles, and reports.
- Social Media Management: Crafting tailored and engaging posts for platforms like Twitter and Instagram.
- Customer Support: Powering chatbots and virtual assistants to deliver personalized responses.
- Language Translation: Providing accurate and fluent translations across languages.
- Creative Writing: Offering prompts or co-writing stories, poems, and scripts.
- Summarization: Condensing lengthy documents into concise summaries for quick comprehension.
Best Practices for Effective Text Generation
- Use High-Quality Training Data: Ensure that datasets are comprehensive, diverse, and free of bias.
- Fine-Tune Models: Customize pre-trained models to suit specific applications or industries.
- Incorporate Ethical Guidelines: Establish safeguards to mitigate misuse and ensure responsible deployment.
- Continuously Monitor Output: Regularly evaluate generated content for quality, relevance, and ethical compliance.
- Leverage Open Source Tools: Platforms like Hugging Face and Python libraries offer resources for building and refining text generation systems.
Conclusion
Text generation has transformed how we approach content creation, communication, and automation. By leveraging advanced techniques such as transformer-based models, it continues to push the boundaries of what machines can achieve in natural language processing. However, addressing challenges like quality, diversity, and ethical considerations is crucial for maximizing its potential and ensuring its responsible use. As this field evolves, text generation will remain a cornerstone of AI’s impact on society, enabling innovative applications across industries and improving accessibility, creativity, and efficiency in countless domains.