What is Random Forest? The Powerful Ensemble Learning Algorithm
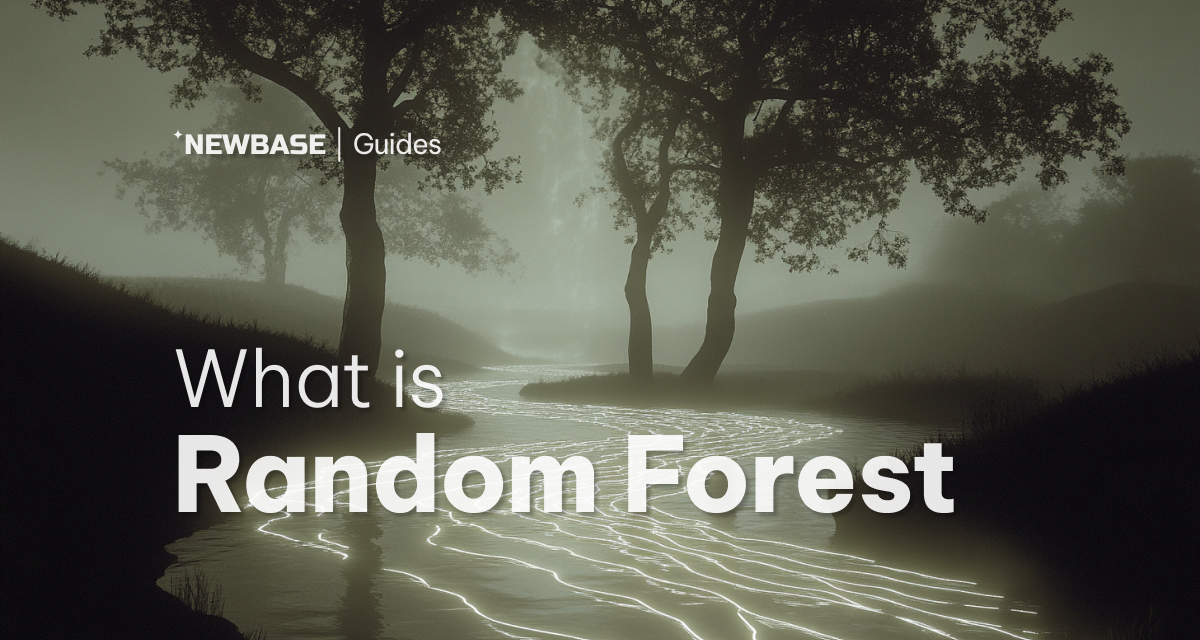
Random forest is a widely-used machine learning algorithm developed by Leo Breiman and Adele Cutler. It operates by combining the predictions of multiple decision trees to achieve a single, robust result. Known for its versatility and ease of use, random forest is adept at handling both classification and regression tasks, making it a cornerstone of modern data science. Its ability to handle large datasets, assess feature importance, and provide accurate predictions has made it a favorite among practitioners in various fields.
Understanding Decision Trees
To fully understand random forest, one must first explore its building blocks: decision trees. A decision tree is a supervised learning model that mimics human decision-making by using a series of conditional questions to classify data or make predictions. For example, to answer the question, “Should I surf?” a decision tree might consider factors like “Is it a long period swell?” or “Is the wind blowing offshore?” Each question forms a decision node, splitting the dataset into subsets, while the final outcomes are represented by leaf nodes. Observations that meet the criteria proceed down the “Yes” branch, while others follow the “No” branch.
Decision trees are typically trained using algorithms like the Classification and Regression Tree (CART) method. Metrics such as Gini impurity, information gain, and mean squared error (MSE) are employed to evaluate the quality of splits. However, while decision trees are intuitive and straightforward, they are prone to significant limitations, including overfitting, high variance, and bias. These limitations make individual decision trees less reliable for complex tasks, necessitating ensemble methods like random forest.
Ensemble Methods: The Foundation of Random Forest
Random forest belongs to a class of algorithms known as ensemble methods, which improve predictive accuracy by aggregating the outputs of multiple models. Ensemble methods rely on the principle that a group of weak learners can combine to form a strong learner. Two primary techniques used in ensemble methods are:
- Bagging (Bootstrap Aggregation): Introduced by Leo Breiman in 1996, bagging involves generating multiple training datasets by sampling with replacement from the original dataset. Each model, trained independently on a different sample, produces predictions that are then averaged (for regression tasks) or combined via majority voting (for classification tasks). This process reduces variance and improves model stability.
- Boosting: Unlike bagging, boosting focuses on sequentially improving weak learners by giving more weight to incorrectly predicted samples in subsequent iterations. While powerful, boosting can sometimes lead to overfitting, making it less robust than bagging in certain scenarios.
Random forest extends the bagging approach by introducing additional randomness in feature selection, which further enhances its predictive power.
The Random Forest Algorithm
The random forest algorithm takes the concept of bagging a step further by incorporating feature randomness, also known as “feature bagging” or the “random subspace method.” This technique involves selecting a random subset of features at each split, ensuring that individual trees within the forest are decorrelated. This decorrelation is crucial for reducing overfitting and improving generalization.
Key Steps in Random Forest
- Bootstrap Sampling: A collection of decision trees is built, each trained on a bootstrap sample—a subset of the training data drawn with replacement. This ensures diversity among the training datasets.
- Feature Randomness: For each decision tree, a random subset of features is selected for splitting at each node. This prevents dominant features from biasing the model and ensures low correlation among trees.
- Prediction Aggregation:
- For regression tasks, the predictions of individual trees are averaged to yield the final output.
- For classification tasks, a majority vote determines the predicted class.
- Out-of-Bag (OOB) Validation: Approximately one-third of the training data, excluded from the bootstrap sample, serves as the out-of-bag sample. This data is used for cross-validation, providing an unbiased estimate of model performance.
Benefits and Challenges of Random Forest
Benefits
- Reduced Overfitting: Individual decision trees often overfit the training data, but random forest mitigates this by averaging predictions from multiple uncorrelated trees. This reduces variance and enhances model robustness.
- Flexibility and Accuracy: Random forest excels in both classification and regression tasks, achieving high accuracy even with noisy or incomplete data. Its ability to impute missing values further adds to its versatility.
- Feature Importance Evaluation: Random forest simplifies the process of assessing feature importance. Common methods include:
- Gini Importance: Measures the total reduction in Gini impurity across all splits involving a feature.
- Mean Decrease Accuracy (MDA): Assesses the impact on model accuracy when feature values are permuted.
Challenges
- Computational Complexity: Building and aggregating predictions from multiple decision trees can be computationally expensive, especially for large datasets.
- Resource Intensity: Random forest requires substantial memory and processing power, making it less suitable for resource-constrained environments.
- Reduced Interpretability: While individual decision trees are easy to interpret, understanding the collective behavior of a random forest can be challenging, especially for non-technical stakeholders.
Applications of Random Forest
Random forest’s versatility has led to its adoption across numerous industries:
- Finance:
- Evaluating customer credit risk.
- Detecting fraudulent transactions.
- Solving option pricing problems.
- Healthcare:
- Gene expression classification.
- Biomarker discovery for personalized medicine.
- Drug response prediction.
- E-commerce:
- Powering recommendation engines for personalized product suggestions.
- Predicting customer churn and optimizing marketing strategies.
- Environmental Science:
- Analyzing climate patterns and predicting weather anomalies.
- Assessing biodiversity and identifying endangered species.
Advanced Insights into Random Forest
Hyperparameter Tuning
To optimize random forest performance, several hyperparameters can be fine-tuned:
- Number of Trees (n_estimators): Increasing the number of trees enhances accuracy but also raises computational cost. A balance must be struck based on the dataset and available resources.
- Maximum Depth: Restricting tree depth prevents overfitting by limiting how deeply the data can be split.
- Minimum Samples Split and Leaf: Setting thresholds for the minimum number of samples required for a split or leaf node ensures that trees do not become overly complex.
- Number of Features (max_features): Adjusting the number of features considered at each split impacts tree decorrelation and overall model accuracy.
Limitations
Despite its robustness, random forest may struggle with highly imbalanced datasets or datasets with categorical variables that have many levels. Techniques such as SMOTE (Synthetic Minority Over-sampling Technique) or one-hot encoding can help address these challenges.
Conclusion
Random forest is a cornerstone algorithm in machine learning, offering a powerful blend of accuracy, flexibility, and robustness. By aggregating the predictions of multiple decision trees, it effectively reduces overfitting, handles missing data, and identifies important features. Despite its computational demands, its wide-ranging applications in finance, healthcare, e-commerce, and beyond make it an indispensable tool for solving complex problems in data science. As machine learning continues to evolve, random forest remains a shining example of the power of ensemble learning.