What is Multimodal AI? The Future of Integrated Intelligence Across Multiple Data Sources
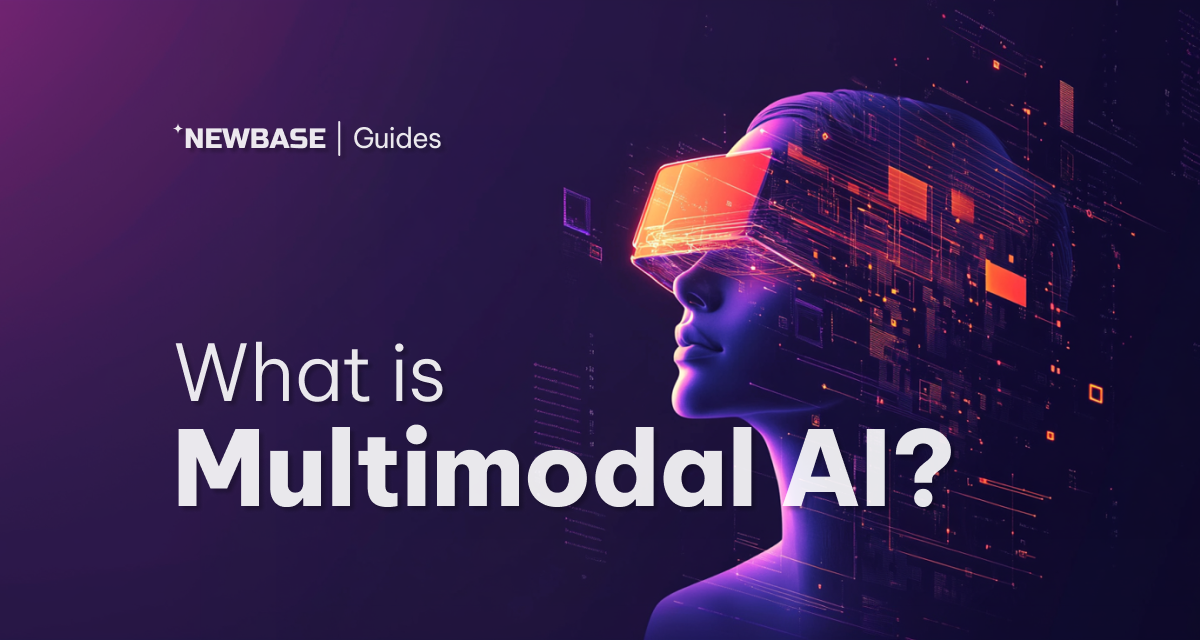
Multimodal AI refers to advanced machine learning models capable of processing, understanding, and integrating information from multiple data modalities, such as text, images, audio, video, and other sensory inputs. These systems go beyond traditional unimodal AI, which is typically designed to handle a single type of data, by synthesizing diverse data sources to deliver a richer, more holistic understanding of complex scenarios and generate more robust and insightful outputs.
Key Features of Multimodal AI
Multimodal AI stands out due to its ability to bridge and combine multiple forms of data, enabling richer interaction and analysis. Examples include:
- A model analyzing a photograph of a landscape and generating a detailed, written summary of its visual characteristics.
- Another model interpreting a textual description of a landscape to create a realistic image based on that description.
This seamless integration of data modalities significantly enhances the functionality and versatility of AI systems. OpenAI’s ChatGPT, launched in November 2022, initially operated as a unimodal AI model, capable of processing only text inputs and outputs via natural language processing (NLP). The introduction of multimodal capabilities in subsequent models, such as DALL-E and GPT-4, highlighted the immense potential of this technology.
Advantages of Multimodal AI
1. Comprehensive Understanding
By integrating multiple data modalities, multimodal AI captures a more nuanced and complete perspective of a given context. For instance, combining visual data with textual descriptions enhances interpretative accuracy and reduces ambiguities.
2. Improved Robustness and Reliability
Multimodal systems exhibit resilience in handling missing or noisy data. If one modality is unreliable or unavailable, the system can leverage information from other modalities to maintain performance and accuracy.
3. Enhanced User Interactions
Multimodal AI systems enable more intuitive and engaging human-computer interactions. For instance, virtual assistants that understand voice commands while processing visual cues deliver smoother and more natural user experiences.
4. Expanded Applications
From personalized product recommendations based on shared photos to bird identification systems that combine image recognition and audio analysis, multimodal AI opens doors to innovative applications across industries.
How Multimodal AI Works
The functioning of multimodal AI is built on the convergence of foundational AI models, advancements in deep learning, and data integration techniques. Multimodal AI draws upon earlier innovations such as audio-visual speech recognition and multimedia content indexing but extends their capabilities through modern techniques like transformers and attention mechanisms.
Core Characteristics of Multimodal AI
A 2022 Carnegie Mellon paper identified three defining attributes of multimodal AI:
- Heterogeneity: Modalities differ in structure, representation, and quality. For example, a textual description of an event contrasts fundamentally in form and content from a photograph of the same event.
- Connections: Modalities often share complementary information, which may manifest as statistical correlations or semantic similarities. These connections are vital for integrating data effectively.
- Interactions: The interplay between modalities enriches data analysis and output generation when synthesized appropriately.
Technical Challenges
Developing effective multimodal AI systems involves overcoming several challenges:
- Representation: Designing representations that encapsulate both the distinct characteristics and interconnections of various modalities. Practitioners employ specialized neural networks, such as convolutional neural networks (CNNs) for images and transformers for text, alongside attention mechanisms and joint embedding spaces.
- Alignment: Identifying and aligning corresponding elements across modalities. Techniques include spatial alignment (e.g., linking text descriptions with regions in images) and temporal alignment (e.g., synchronizing video and audio streams).
- Reasoning: Combining knowledge from different modalities through multi-step inferential processes to draw meaningful conclusions.
- Generation: Developing systems that can produce coherent and structured outputs across modalities, reflecting cross-modal interactions and maintaining consistency.
- Transference: Transferring knowledge across modalities using techniques like shared embedding spaces and transfer learning to enhance system versatility.
- Quantification: Conducting empirical and theoretical studies to evaluate and refine multimodal learning approaches, ensuring reliable performance.
Fusion Techniques
Data fusion—the process of integrating information from multiple modalities—is central to multimodal AI. Fusion occurs at different stages:
- Early Fusion: Combining modalities into a unified representation early in the processing pipeline.
- Mid Fusion: Merging data at intermediate stages of preprocessing or feature extraction.
- Late Fusion: Independently processing each modality before merging their outputs for a final decision or analysis.
Trends Shaping Multimodal AI
1. Unified Models
Unified architectures, such as OpenAI’s GPT-4 Vision and Google’s Gemini, are designed to seamlessly handle text, images, and other data types within a single framework. These models exemplify the move towards cohesive multimodal systems.
2. Enhanced Cross-Modal Interaction
Sophisticated attention mechanisms and advanced transformers enable better alignment and integration of data from various modalities, producing more coherent and contextually accurate outputs.
3. Real-Time Multimodal Processing
Applications in autonomous vehicles and augmented reality demand real-time integration and analysis of data from multiple sensors, such as cameras, LIDAR, and GPS, to enable instantaneous decision-making.
4. Multimodal Data Augmentation
Synthetic datasets combining multiple modalities (e.g., pairing textual descriptions with corresponding images) are being developed to improve model training and performance.
5. Open Source Collaboration
Initiatives such as Hugging Face and Google AI provide open-source tools and platforms, fostering a collaborative environment for advancing multimodal AI research and applications.
Applications of Multimodal AI
Healthcare
Multimodal AI aids in diagnosing diseases by analyzing medical images, combining them with patient histories, and integrating laboratory results for more accurate assessments.
Autonomous Vehicles
Self-driving cars use multimodal systems to process data from cameras, LIDAR, radar, and GPS, ensuring safe navigation through complex environments.
Education
Interactive learning tools powered by multimodal AI combine textual content, visuals, and audio to create engaging educational experiences.
Entertainment and Media
In creative industries, multimodal AI generates multimedia content by synthesizing text, images, and audio. For example, video game developers use these systems to create dynamic and immersive environments.
Retail and E-commerce
By integrating user-uploaded photos, product specifications, and customer reviews, multimodal AI provides personalized recommendations and enhances the shopping experience.
Future Prospects
As multimodal AI evolves, its potential applications and impact are expected to expand significantly. Areas such as virtual reality, personalized healthcare, and advanced robotics will likely benefit from its integration. Ethical considerations and responsible AI development will also play crucial roles in shaping its trajectory.
Conclusion
Multimodal AI represents a transformative leap in artificial intelligence, allowing systems to synthesize diverse data types for enhanced understanding, decision-making, and functionality. With its ability to bridge gaps across modalities, this technology is poised to revolutionize industries, create more intuitive and human-like systems, and push the boundaries of what AI can achieve.