What Is LoRA? Examining Stable Diffusion’s Low-Rank Adaptation
LoRA models are small fine-tuned Stable Diffusion models that apply minimal parameter changes to standard model checkpoints. They are typically 10 to 100 times smaller in size than checkpoint models. This compact size makes LoRA models very appealing for users seeking to maintain extensive model collections.
This tutorial provides an introduction to LoRA models for beginners without prior experience using them. Users will learn about the nature of LoRA models, where to find them, and how to utilize them within the AUTOMATIC1111 graphical user interface (GUI). The tutorial concludes with demonstrations of several LoRA models.
What are LoRA models?
LoRA (Low-Rank Adaptation) is a fine-tuning technique for Stable Diffusion models. While training methods such as Dreambooth and textual inversion exist, LoRA offers an advantageous balance between file size and fine-tuning capability. Dreambooth results in substantially large model files (2-7 GB), while textual inversions are extremely small (approximately 100 KB) but allow for limited customization.
LoRA sits between these two approaches. File sizes range from 2-200 MB, and fine-tuning power remains considerable. Stable Diffusion users maintaining extensive model collections can attest to how quickly local storage fills. LoRA effectively solves storage constraints.
Like textual inversions, a LoRA model cannot be used independently and must be utilized alongside a model checkpoint. LoRA customizes styles by applying minimal parameter changes to the accompanying checkpoint. LoRA enables style customization without exhausting local storage.
How does LoRA work?
LoRA applies minor modifications to the most critical component of Stable Diffusion models: the cross-attention layers. This component is where the image and prompt information interact. Researchers found it sufficient to fine-tune only this component to achieve effective training results. The cross-attention layers represent the core architecture of the Stable Diffusion model, as shown below.
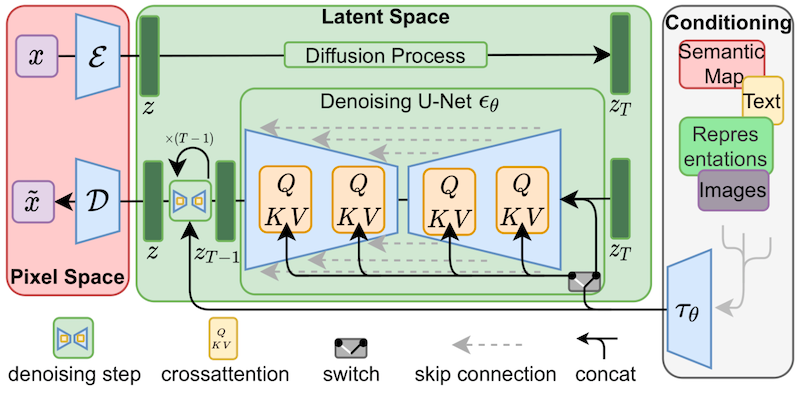
LoRA fine-tunes the cross-attention layers (the question-key-value or “QKV” components of the U-Net noise predictor).
The weights in a cross-attention layer are organized in matrix form. Matrices simply represent numbers arranged in rows and columns, similar to a spreadsheet. A LoRA model fine-tunes an existing model by incorporating its weights into these matrices.
Although LoRA models require storing the same number of weights, their file sizes are smaller due to a decomposition technique. The weights matrix is decomposed into two smaller “low-rank” matrices, represented by fewer total values. For example, a 1,000×2,000 matrix containing 2,000,000 values can be decomposed into a 1,000×2 matrix and a 2×2,000 matrix containing just 6,000 values, representing a storage reduction of over 333 times. This decomposition enables significantly smaller file sizes for LoRA models.
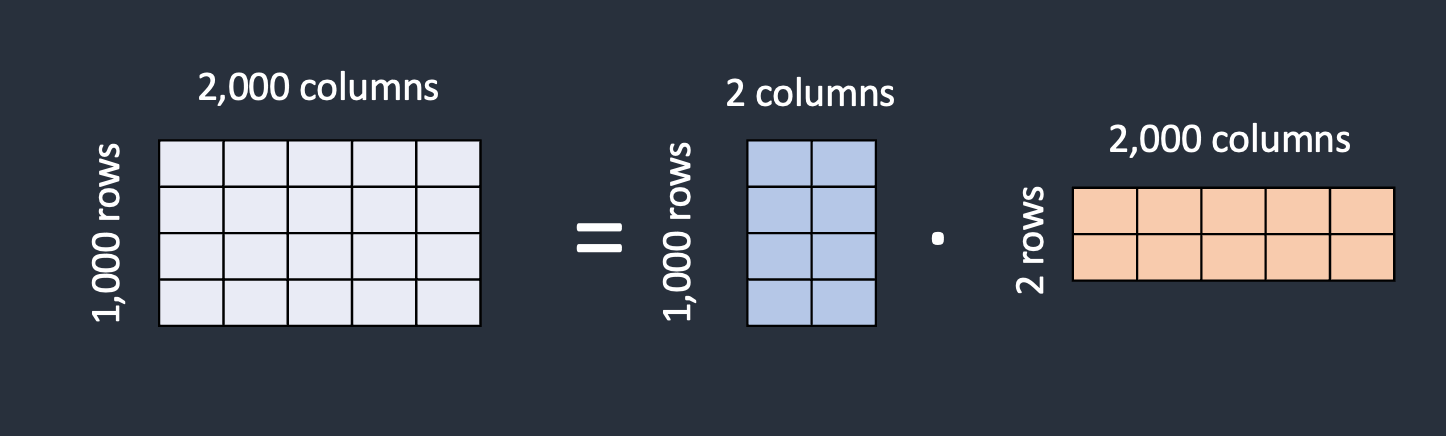
LoRA decomposes large matrices into two smaller low-rank matrices. In the example, the rank of the decomposed matrices is two, which is much lower than the original dimensions – hence the term “low-rank”. The rank can be as low as one.
Research has found this decomposition technique, when applied only to cross-attention layers, does not meaningfully impact the effectiveness of fine-tuning. Therefore, LoRA achieves model compression without functional compromise.
Where to find LoRA models?
Civitai
Civitai hosts one of the largest collections of LoRA models. Users can apply a LoRA filter to view only models generated by this technique. While the site contains a variety of styles such as female portraits, anime, and realistic illustrations, the models do tend to share certain commonalities. It should be noted that Civitai also hosts some not safe for work (NSFW) content, so appropriate filters should be applied depending on individual preferences.
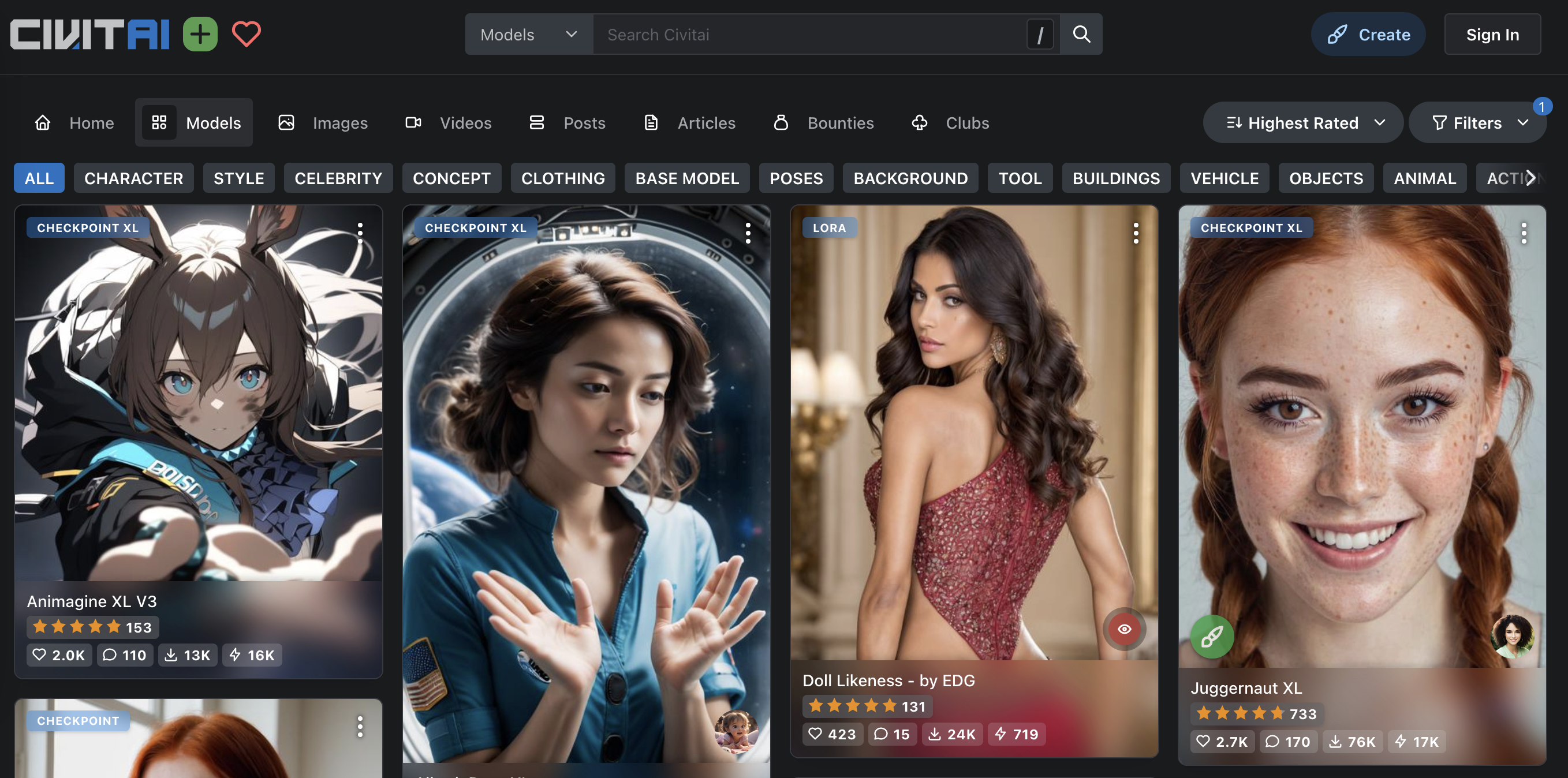
Hugging Face
Hugging Face also provides access to LoRA models through its library. While the selection is more limited in size compared to other sources, Hugging Face offers more diversity in the types of LoRA models available. Users interested in LoRA models hosted on Hugging Face can search the library or browse pre-trained models directly.
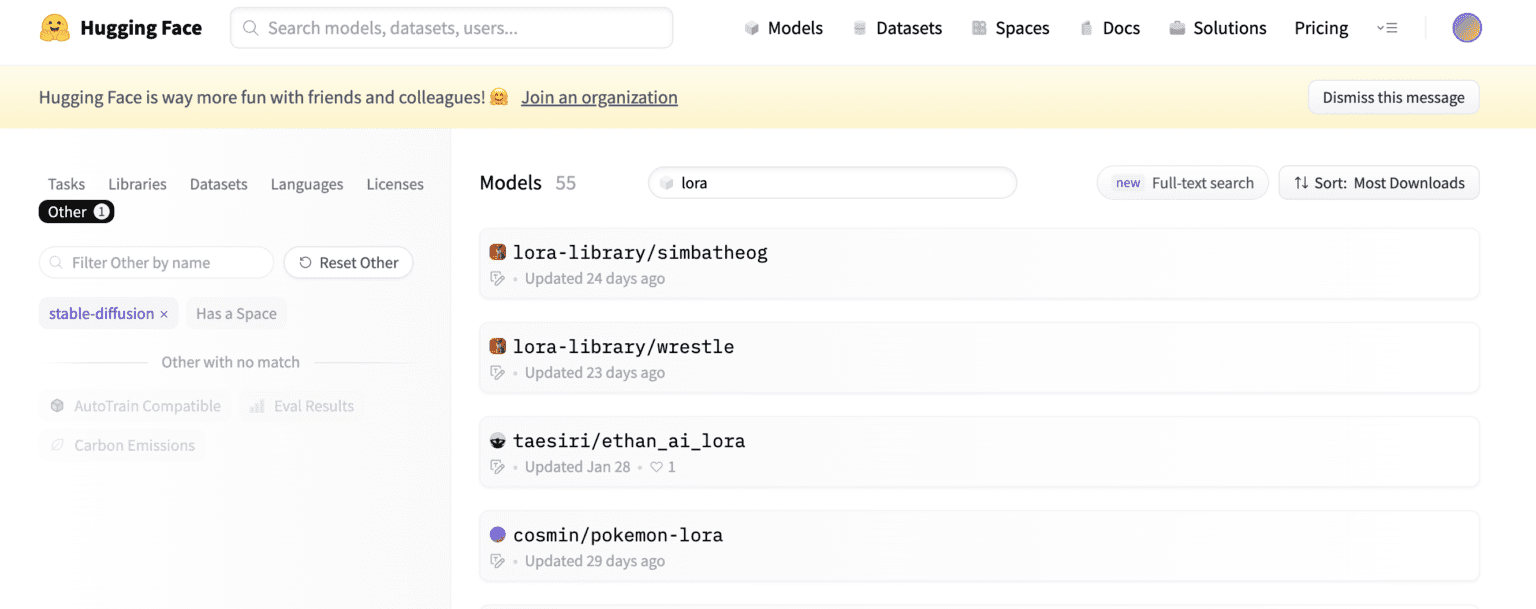
Search LoRA models in Hugging Face
LoRA Library in Hugging Face
How to use LoRA with AUTOMATIC1111?
The AUTOMATIC1111 Stable Diffusion GUI supports LoRA models natively, requiring no additional extensions.
Step 1: Install the LoRA Model
To install LoRA models in the AUTOMATIC1111 Stable Diffusion GUI, place the model files in the following folder:
stable-diffusion-webui/models/Lora
Step 2: Utilize LoRA in Prompts
To incorporate a LoRA model with a specified weight in the AUTOMATIC1111 Stable Diffusion GUI prompt or negative prompt, use the following syntax:
<lora: name: weight>
The “name” denotes the LoRA model name, which may differ from the filename.
Weight signifies the importance applied to the LoRA model. It functions similar to a keyword weighting.The default value is 1. Assigning it to 0 deactivates the model.
To verify the name is accurate, click the LoRA tab instead of typing this phrase.
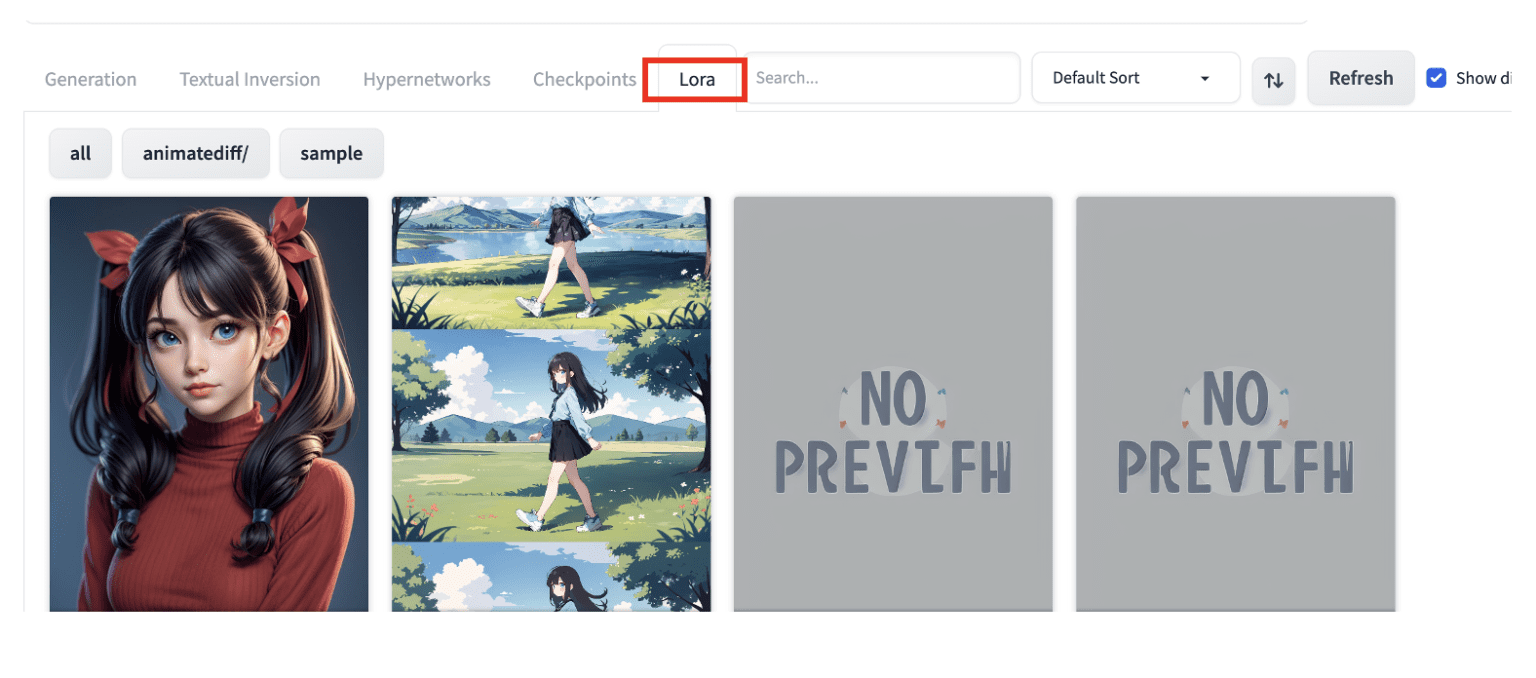
You should see a list of the LoRA devices that are set up. Feel free to select the one you’d like to connect to. Once you’ve made your choice, the LoRA message will be added to the instructions.
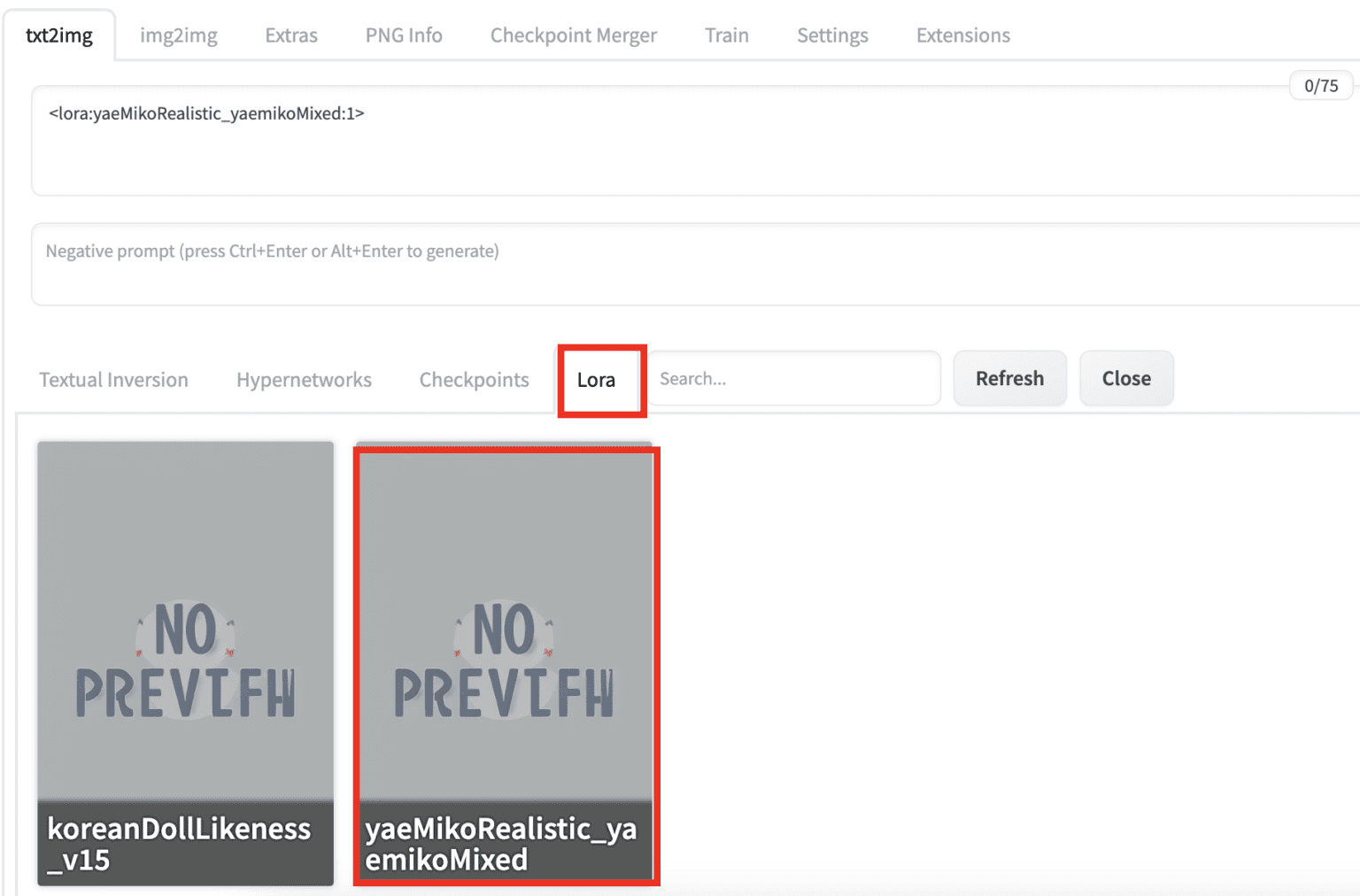
Notes on using LoRA
You can change the multiplier to increase or decrease the effect. Setting the multiplier to zero will turn off the LoRA model. You can adjust the style level between zero and one.
Some LoRA models are trained with Dreambooth technology. You’ll need to include a special trigger keyword to use the LoRA model. You can find the special word on the model’s page.
Similar to embeddings, you can use multiple LoRA models at the same time. You can also combine them with embeddings.
In AUTOMATIC1111, the prompt for LoRA is removed after the model is applied. So you can’t use prompt syntax like [keyword1:keyword2: 0.8] with these models.
Helpful LoRA models:
Detail Tweaker
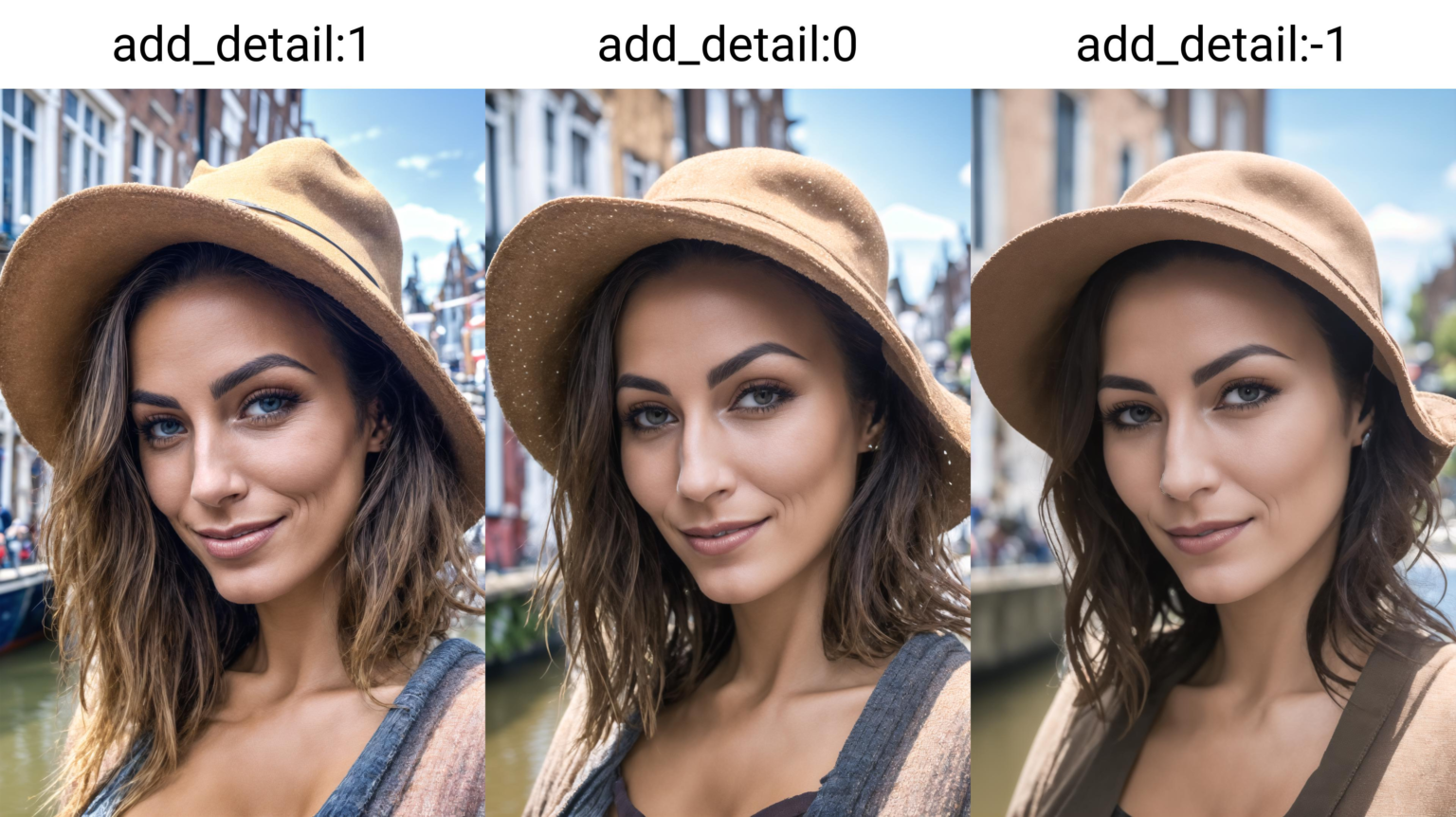
Detail Tweaker provides users with the ability to adjust the level of fine details present within digital images. This application allows for increasing or decreasing the amount of nuanced elements shown through the selection of a LoRA weight value.
A positive weight will serve to heighten subtle image features, while a negative weight reduces finer visual particulars. Detail Tweaker thus furnishes an effective means of customizing the degree of specifics displayed for any given photograph.
Epi Noise Offset
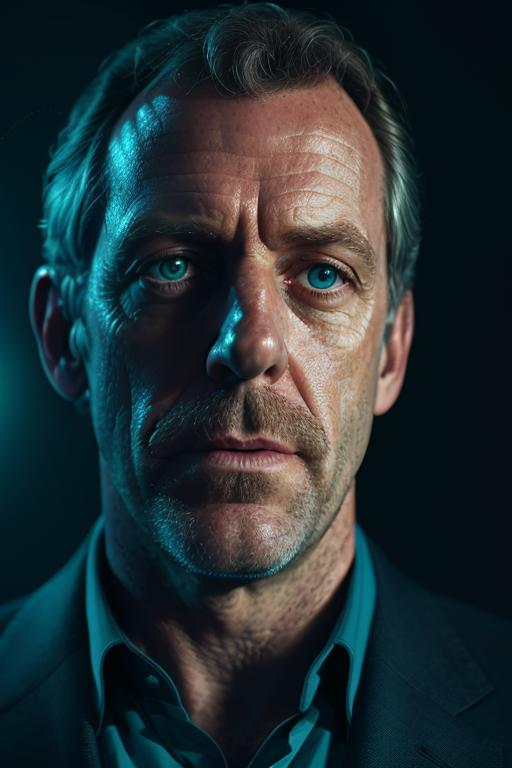
While many Stable Diffusion v1.5 models have difficulty generating images with low light levels, the Epi Noise Offset LoRA modification allows for the creation of dark images using any v1.5 model. Keywords such as “dark studio,” “rim lighting,” “two tone lighting,” “dimly lit,” and
“low key” can be used to induce an effect of lowered brightness and contrast when generating images with this modified model.
Better Portrait lighting
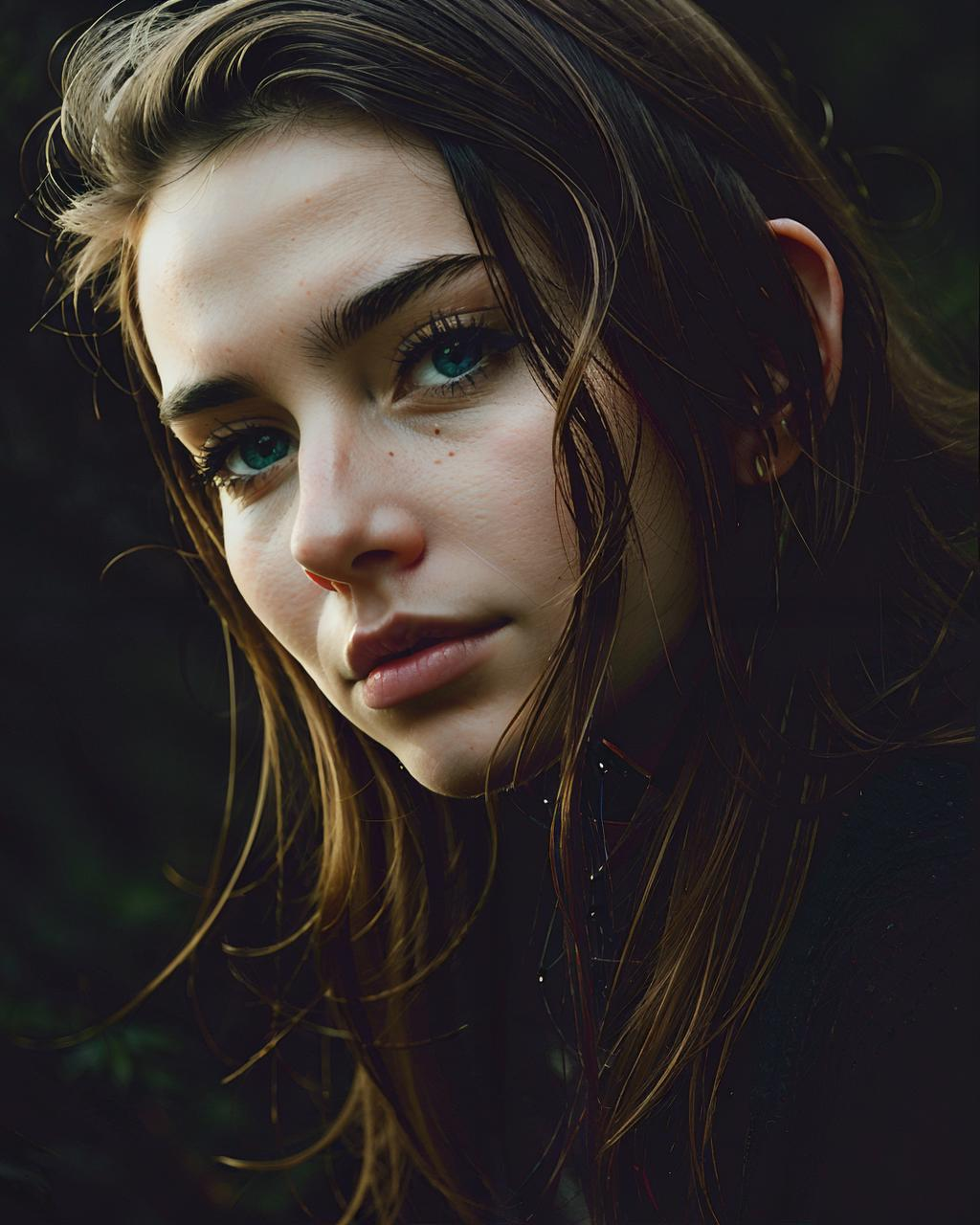
Better Portrait Lighting LoRA technology can effectively improve image lighting quality. For photographers working in portrait or other photorealistic styles, applying BEAP may prove a useful technique worth exploring.
Interesting LoRA models
Here are some of the LoRA models we like.
Dissolve Style [SDXL]
A dissolve into particles style, the trigger word is dissolve, works with sand, particles, and dust! you can specify the subject and background too
Prompt:
painting, martius_storm red ominous war [:style of vincent van gogh and leonardo da vinci:0.4] <lora:ral-dissolve:1> ral-dissolve
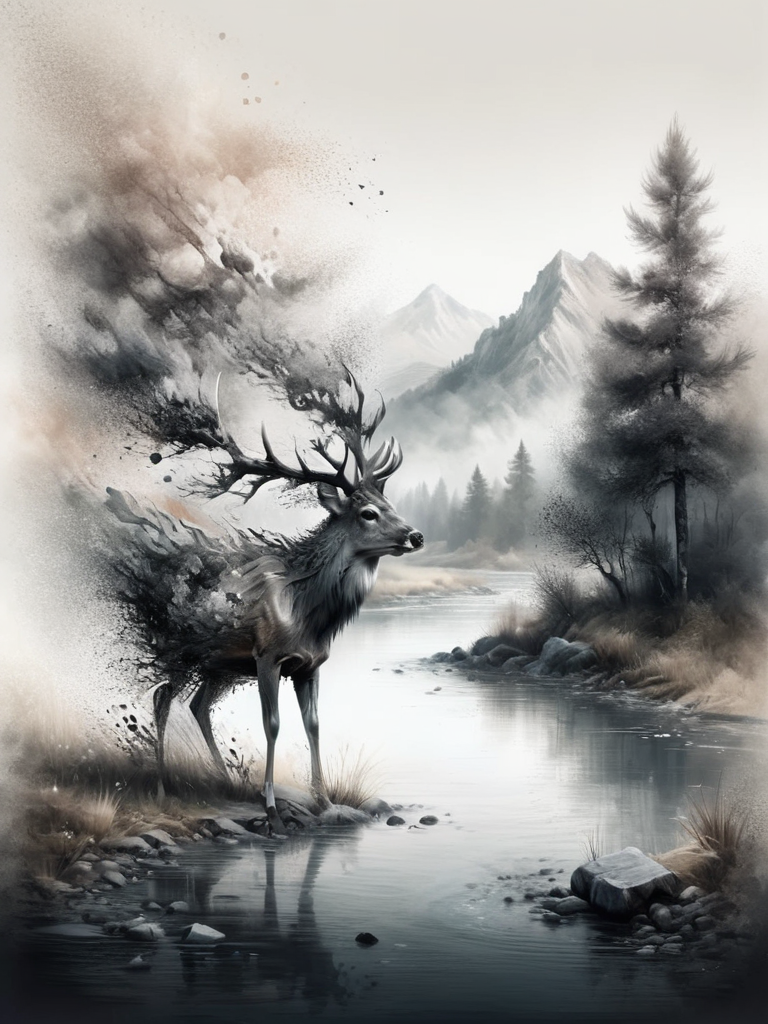
Akira Style XL Style
A 1988 Japanese animated cyberpunk action film
Prompt:
Comic panel illustration of woman prostitute on the street, wearing a fuzzy jacket, red lighting, neon lights, new tokyo, 1girl, solo, akira style <lora:Akira_Style_XL>
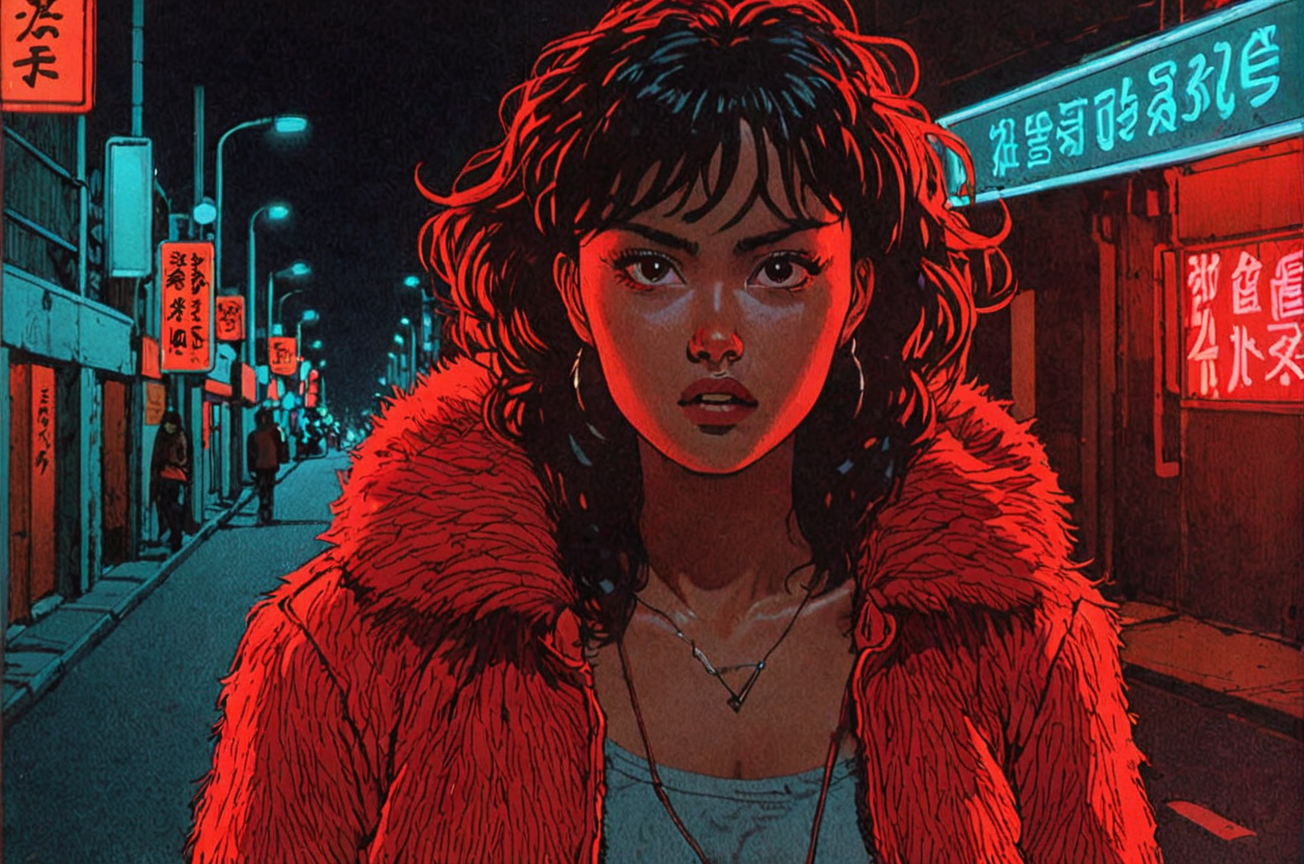
Summary
Low-rank adaptation (LoRA) models can provide efficient fine-tuning of larger checkpoint models. By including specific prompts, LoRA models may be readily utilized within AI assistants to leverage their fine-tuned capabilities.
Further guidance on training LoRA models will be provided in future articles.
A selection of additional resources for interested parties includes:
- “Using LoRA for Efficient Stable Diffusion Fine-Tuning” (Hugging Face blog). This overview examines LoRA’s application to Stable Diffusion fine-tuning.
- “LoRA: Low-Rank Adaptation of Large Language Models” (2021). The seminal research first proposing the LoRA technique, particularly within natural language models.
- “Low-rank Adaptation for Fast Text-to-Image Diffusion Fine-tuning” (Github). Along with demonstrating an initial LoRA application to Stable Diffusion, this repository contains an excellent technical description of the LoRA method.