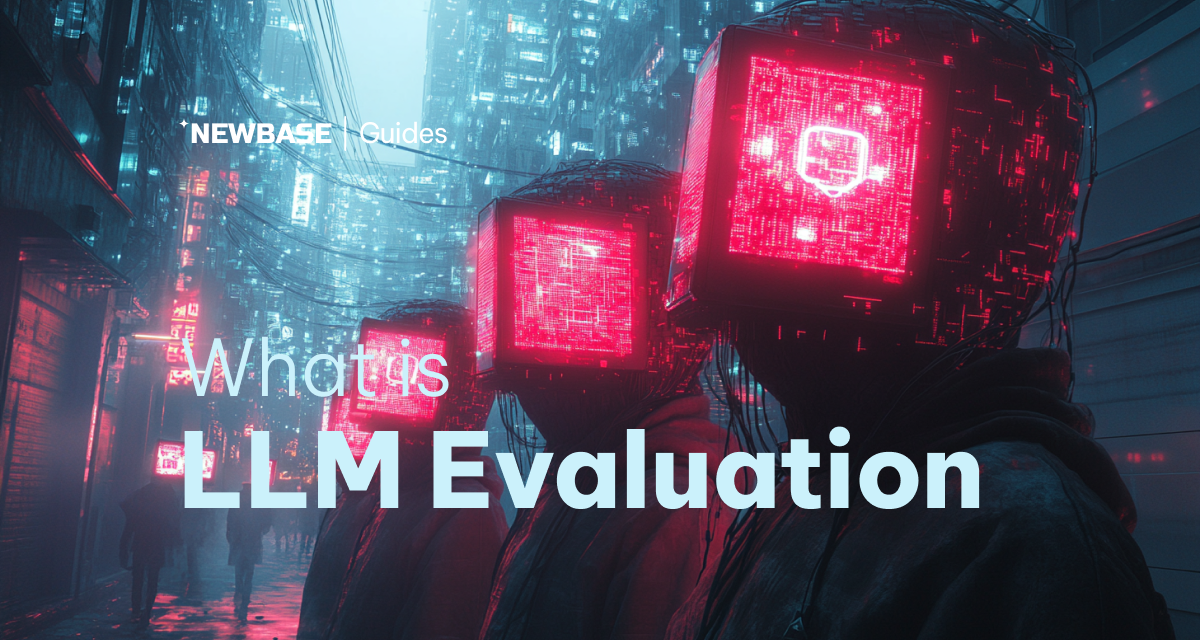
Large language models (LLMs) are revolutionizing AI, but ensuring their effectiveness requires rigorous testing. Enter LLM evaluation, the process of assessing an LLM’s performance through tasks, data, and metrics.
Imagine hiring a new employee. Their qualifications are stellar, but do they deliver high-quality work? Similarly, LLM evaluation goes beyond functionality to assess accuracy, coherence, and reliability.
Why is LLM Evaluation Important?
LLM evaluation serves several crucial purposes:
- Model Performance: It verifies if the LLM performs as intended, generating high-quality outputs across various domains and tasks.
- Ethical Considerations: It helps identify and mitigate potential biases or inaccuracies in model responses.
- Comparative Benchmarking: It facilitates comparing different models to choose the best one for specific use cases.
- New Model Development: Insights from evaluation guide the development of new models and training techniques.
- User and Stakeholder Trust: Transparency in evaluation builds trust in LLM outputs and fosters confidence in AI tools.
LLM Evaluation vs. LLM System Evaluation
While closely related, these evaluations have distinct focuses:
- LLM Evaluation (Model Evaluation): Assesses the core language model’s ability to understand and generate text across tasks and domains. It focuses on raw capabilities like language understanding, output quality, and task-specific performance.
- LLM System Evaluation: Provides a more comprehensive view of the LLM-powered application’s end-to-end performance. It looks at scalability, security, and integration with other components (APIs, databases).
Think of LLM evaluation as ensuring the LLM works for specific tasks, while system evaluation offers a broader view of its overall effectiveness. Both are essential for robust LLM applications.
LLM Evaluation Metrics
The first step is defining evaluation criteria based on the LLM’s intended use. Common metrics include:
- Accuracy: Measures correct responses in tasks like classification or question answering.
- Recall: Measures the number of true positives in LLM responses.
- F1 Score: Combines accuracy and recall into a single metric.
- Coherence: Assesses the logical flow and consistency of generated text.
- Perplexity: Measures how well the model predicts a sequence of words.
- BLEU (Bilingual Evaluation Understudy): Assesses machine-generated text quality, especially in translation tasks.
- ROUGE (Recall-Oriented Understudy for Gisting Evaluation): Evaluates text summaries by comparing them to human-created ones.
- Latency: Measures the model’s efficiency and speed.
- Toxicity: Measures the presence of harmful or offensive content in model outputs.
Applying LLM Evaluation Frameworks and Benchmarks
Evaluators establish clear criteria and select an evaluation framework that offers a comprehensive methodology. Examples include IBM’s Foundation Model Evaluation framework (FM-eval). These frameworks work alongside LLM benchmarks – standardized datasets or tasks used to analyze results. Some widely used LLM benchmarks include:
- MMLU (Massive Multitask Language Understanding) dataset: Tests on question-answering, machine translation, summarization, and sentiment analysis.
- HumanEval: Assesses LLM performance in code generation, especially functional correctness.
- TruthfulQA: Addresses hallucination problems by measuring an LLM’s ability to generate truthful answers.
- General Language Understanding Evaluation (GLUE) and SuperGLUE: Test NLP models on language-understanding tasks.
- Hugging Face datasets library: Provides open-source access to numerous evaluation datasets.
Zero-shot, few-shot, and fine-tuning tests are used to evaluate how well an LLM operates within these benchmarks. LLM evaluation results are then used to refine and iterate the model.
LLM as a Judge vs. Humans in the Loop
There are two evaluation approaches:
- LLM-as-a-judge: The LLM itself evaluates the quality of its outputs, using metrics or comparing text to ground-truth data.
- Human-in-the-loop: Human evaluators assess the quality of LLM outputs, useful for nuanced assessments like coherence or user experience.
LLM Evaluation Use Cases
- Evaluating question-answering systems: Ensuring the accuracy of answers generated by LLMs.
- Assessing fluency and coherence of generated text: Evaluating chatbots or machine translation systems.
- Detecting bias and toxicity: Identifying and mitigating potential biases and harmful content.
- Comparing LLM performance: Comparing different LLMs across various NLP tasks.
These uses cases highlight how LLM evaluation can lead to a better user experience, fewer risks, and a potential competitive advantage.
Challenges of LLM Evaluation
While LLM evaluation is crucial, it presents several challenges:
- Standardization: The rapid evolution of LLMs makes it difficult to establish standardized benchmarks that remain relevant over time.
- Contextual Understanding: Evaluating an LLM’s ability to understand and respond to complex, nuanced queries remains a challenge.
- Bias and Fairness: Assessing the fairness and lack of bias in LLM outputs is complex, requiring careful consideration of various social and cultural factors.
- Explainability: Understanding the internal workings of LLMs can be difficult, making it challenging to explain their decision-making processes.
- Real-world Applications: Evaluating the performance of LLMs in real-world applications, such as healthcare or finance, requires careful consideration of domain-specific factors.
The Future of LLM Evaluation
As LLMs continue to advance, so too will the methods for evaluating them. Some potential future developments include:
- Automated Evaluation Metrics: Developing more sophisticated metrics that can accurately assess the quality of LLM outputs.
- Human-in-the-Loop Evaluation: Combining human judgment with automated metrics to provide a more comprehensive evaluation.
- Benchmarking for Specific Domains: Creating specialized benchmarks for specific domains, such as healthcare or finance.
- Ethical Evaluation: Developing frameworks to assess the ethical implications of LLM outputs.
By addressing these challenges and embracing new approaches, we can ensure that LLMs are developed and deployed responsibly, leading to more reliable and beneficial AI applications.