What is Llama 2? Open Approach to Revolutionizing AI for Everyone
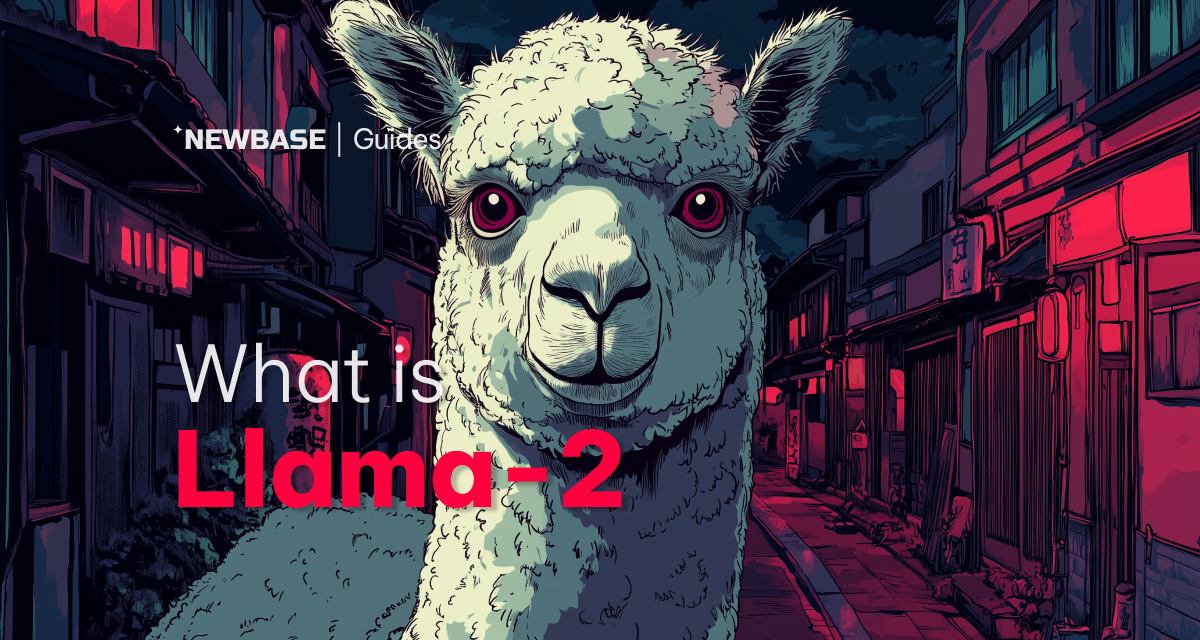
Llama 2 is a groundbreaking family of pre-trained and fine-tuned large language models (LLMs) introduced by Meta AI in 2023, marking a significant advancement in the field when compared to other prominent LLMs like OpenAI’s GPT-4 and Google’s PaLM 2. Designed for both research and commercial applications, these models are available free of charge and excel at a wide range of natural language processing (NLP) tasks, such as text generation and programming code creation. Llama 2 represents a significant leap forward from its predecessor, LLaMa 1, which was released in 2022 with restricted access for noncommercial purposes.
Key Features of Llama 2
The Llama 2 models come in two categories: base foundation models and fine-tuned “chat” models. These offerings are designed to cater to different applications, providing flexibility to researchers and developers. Unlike LLaMa 1, Llama 2 is openly accessible for both academic research and commercial use, fostering a more democratized AI ecosystem. For example, it has enabled smaller enterprises and independent developers to experiment with and deploy advanced AI solutions, such as chatbots for customer service or tools for automating routine tasks, without the need for substantial infrastructure investments.
Advancing AI Accessibility
Meta AI’s Llama 2 aims to lower the barriers to entry in the generative AI landscape by addressing challenges such as high development costs, limited access to advanced models, and the dominance of proprietary systems. By offering free access to its code and model weights, Llama 2 empowers startups, researchers, and smaller organizations to develop AI solutions that were previously out of reach. The high computational costs associated with developing state-of-the-art LLMs have traditionally limited their creation and use to a few dominant players, such as OpenAI, Google, and Anthropic. These companies often deploy proprietary, closed-source models like GPT, Bard, and Claude. In contrast, Llama 2 provides open access to its code and model weights, enabling a broader range of organizations—from startups to academic researchers—to utilize cutting-edge AI technologies without excessive infrastructure investments.
Model Variants and Efficiency
Llama 2 models are available with three parameter configurations: 7 billion (7B), 13 billion (13B), and 70 billion (70B). By focusing on optimizing smaller models rather than merely increasing parameter counts, Llama 2 allows for efficient deployment on local hardware, making advanced AI more accessible to smaller organizations and individual developers. For instance, a startup specializing in personalized education tools leveraged Llama 2 to build a custom tutoring assistant, achieving powerful performance without the need for extensive cloud infrastructure.
Llama 2 vs. LLaMa 1
Llama 2 offers several improvements over its predecessor, LLaMa 1:
- Extended Context Length: Llama 2 models support a context length of 4,096 tokens, double that of LLaMa 1. This enhancement allows for more complex and coherent exchanges in natural language tasks.
- Wider Accessibility: While LLaMa 1 was restricted to academic research, Llama 2 is freely available for commercial use by organizations with fewer than 700 million monthly active users.
- Enhanced Training: Llama 2 was trained on 40% more data than its predecessor, resulting in a richer knowledge base. Additionally, Llama 2’s chat models incorporate reinforcement learning from human feedback (RLHF), aligning their outputs more closely with human expectations.
Open Source or “Open Approach”?
While Meta markets Llama 2 as a freely available model, its licensing terms have sparked debate about whether it qualifies as “open source.” According to the Open Source Initiative (OSI), true open-source software must adhere to specific criteria, including non-discrimination and unrestricted use. Llama 2’s licensing conditions—such as requiring approval for organizations with over 700 million users and prohibiting applications like those involving surveillance or harm to individuals—do not meet these standards. As a result, some in the tech community refer to Llama 2’s accessibility as an “open approach” rather than fully open source.
How Does Llama 2 Work?
Llama 2 models are transformer-based autoregressive causal language models, meaning they rely on a deep learning architecture known as the transformer, which excels at processing sequential data. In this context, “autoregressive” indicates that the model generates text one token at a time, predicting each subsequent token based on the preceding ones. “Causal” refers to the unidirectional nature of this process, where only prior context is used to generate future outputs. They use self-supervised learning during pre-training, where they predict the next word in a sequence based on prior input. This process allows the models to learn linguistic and logical patterns from vast datasets, comprising 2 trillion tokens from publicly available sources.
Base Models and Fine-Tuning
The base Llama 2 models serve as foundational frameworks that developers can fine-tune for specific tasks. For instance, prominent derivative models include:
- Alpaca: Fine-tuned for instruction-following by Stanford University.
- Vicuna: A chat assistant optimized for user conversations.
- Orca: Trained by Microsoft for advanced reasoning tasks.
- WizardLM: Fine-tuned using synthetic instruction data to achieve near ChatGPT-level performance.
The fine-tuning process involves techniques like supervised learning and reinforcement learning to adapt the base models for applications such as dialogue generation and creative writing.
Llama 2 Chat Models
Llama 2’s chat models are specifically fine-tuned for dialogue-based applications. Through supervised fine-tuning (SFT) and RLHF, these models are trained to produce responses that align with user expectations. Meta AI’s approach prioritizes quality over quantity, using a smaller but highly curated dataset of 27,540 annotated examples for SFT. RLHF further refines the models’ behavior, incorporating human feedback to optimize helpfulness and safety.
Specialized Variants: Code Llama
Built on Llama 2, Code Llama is a fine-tuned model designed for code generation and related tasks. Supporting major programming languages like Python, C++, and Java, Code Llama is available in parameter sizes of 7B, 13B, and 34B, with a context length of up to 100,000 tokens. Two specialized versions, Code Llama – Python and Code Llama – Instruct, cater to Python-specific tasks and instruction-based interactions, respectively.
Llama 2 vs. Closed-Source Models
Llama 2’s smaller size and open availability make it an attractive alternative to proprietary models like OpenAI’s GPT-4 or Google’s PaLM 2. While these closed-source models boast larger parameter counts and broader capabilities, Llama 2 excels in areas such as efficiency, safety, and deployment flexibility.
- Safety: Llama 2 models exhibit lower safety violation rates compared to competitors like ChatGPT and Google’s PaLM Bison.
- Privacy and Cost-Efficiency: Smaller model sizes enable local deployment, preserving data privacy and reducing reliance on expensive cloud-based infrastructure.
Practical Applications and Access
Llama 2 models can be accessed through several platforms, including Hugging Face, Microsoft Azure, and Amazon SageMaker. Developers can also download model weights and implementation guides directly from Meta’s GitHub repository. These models integrate seamlessly with machine learning frameworks like PyTorch, allowing for diverse use cases ranging from enterprise AI solutions to mobile app development.
Conclusion
Llama 2 represents a pivotal step in making advanced AI technologies more accessible and efficient. By focusing on smaller models with robust capabilities, Meta AI has created a tool that balances performance, safety, and usability. Whether for research, commercial development, or individual exploration, Llama 2 is poised to play a transformative role in the AI landscape.