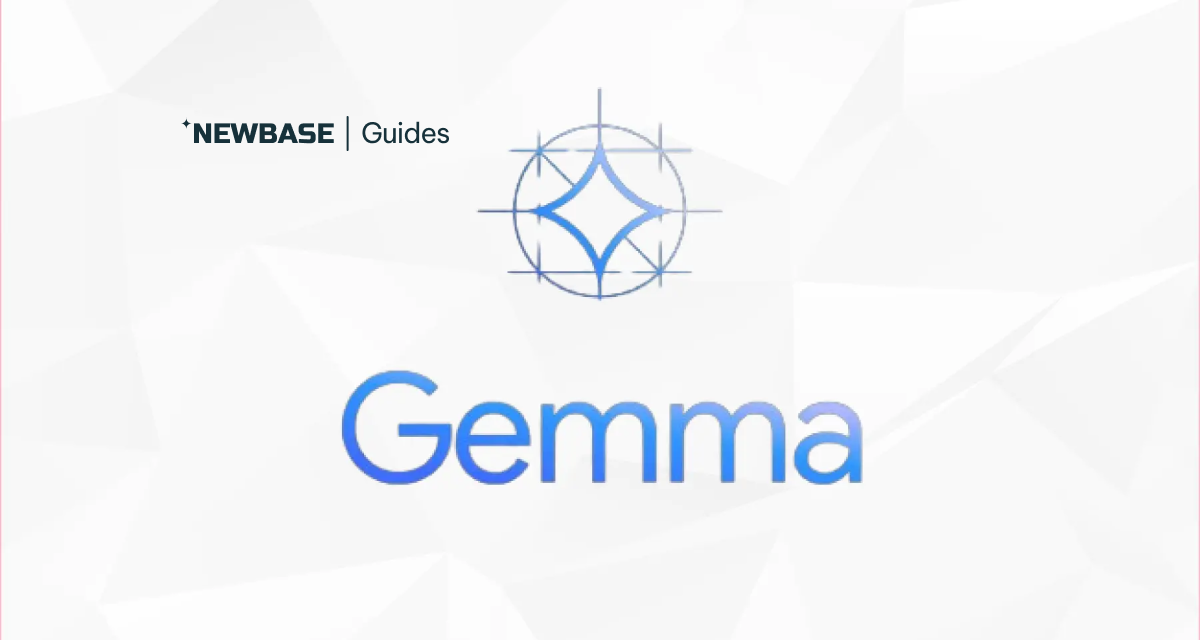
Google Gemma is a family of free and open-source small language models (SLMs) built with the same technology as the larger Google Gemini family. Think of them as lightweight versions of Gemini, designed for easier deployment on various devices.
Key Features of Gemma:
- Open-source and free: Anyone can access and use Gemma models for personal or commercial purposes.
- Lightweight: Optimized for deployment on laptops, mobiles, as well as NVIDIA GPUs and Google Cloud TPUs.
- Text-to-text AI: Primarily focused on tasks involving text generation and manipulation.
- Multiple generations: Includes both first-generation (Gemma) and second-generation (Gemma 2) models with varying functionalities.
- Specialized models: Gemma offers models like CodeGemma and DataGemma for specific tasks like code completion and data retrieval.
- Multilingual (limited): While Gemma 1 primarily focused on English content, Gemma 2 offers some support for other languages.
- Instruction tuning: Gemma models can be fine-tuned to better follow specific instructions.
Gemma Model Breakdown:
- Gemma & Gemma 2: The core models, available in various parameter sizes (2B to 27B) trained on web documents, code, and scientific articles.
- CodeGemma: A specialized model for code-related tasks, supporting multiple programming languages.
- DataGemma: Combines Gemma models with Google’s Data Commons for data-driven responses.
- PaliGemma: A vision-language model that understands and generates text based on images.
- RecurrentGemma: Employs a recurrent neural network architecture for faster inference, particularly for long sequences.
Gemma Use Cases:
- Building chatbots and conversational AI assistants
- Text editing and proofreading
- Answering questions and research tasks
- Text generation (emails, marketing copy, etc.)
- Text summarization, especially for lengthy documents
How Does Gemma Work?
Gemma is based on transformer models, a neural network architecture that analyzes input sequences and generates outputs. It uses a “decoder-only” variant, focusing on generating the output sequence directly from the input.
- Embeddings: Input sequences are converted into numerical representations capturing meaning and position.
- Self-attention: The model focuses on the most important parts of the input sequence.
- Output generation: The decoder uses the processed information to generate the most likely output sequence.
Gemma Performance:
- Gemma models perform competitively with similar-sized SLMs like Llama 3 and Mistral on various benchmarks.
- Larger Gemma 2 models outperform smaller ones, demonstrating improved capabilities.
- Newer SLMs from Meta and Mistral have surpassed some Gemma models in specific benchmarks.
Accessing Gemma:
- Google AI Studio
- Hugging Face
- Kaggle
- Vertex AI Model Garden
- Open-source frameworks (JAX, LangChain, PyTorch, TensorFlow)
- APIs like Keras 3.0
- NVIDIA tools (NeMo, TensorRT-LLM) for fine-tuning and optimization
Deployment Options:
- Google Cloud Vertex AI and Google Kubernetes Engine (GKE) for enterprise deployments.
- Google Colab for free cloud-based access to computing resources (limited power users).
Gemma Risks:
- Bias: Gemma models can inherit biases from their larger counterparts.
- Hallucinations: Outputs require verification to ensure accuracy and factual correctness.
- Privacy violations: Careful data handling is essential to avoid leaks during fine-tuning.
While Google has evaluated Gemma for safety concerns and released a Responsible Generative AI Toolkit, it’s crucial to be aware of potential risks associated with AI models.
Sources and related content
The Future of Gemma and Generative Models
As generative models continue to evolve, we can expect to see even more impressive capabilities from Gemma and other similar models. Here are some potential future directions:
Enhanced Capabilities
- Multimodal Understanding: Gemma could be extended to understand and generate content in multiple modalities, such as text, images, and audio.
- Improved Reasoning and Problem-Solving: Gemma could be trained on more diverse datasets to enhance its reasoning and problem-solving abilities.
- More Efficient and Cost-Effective: Advancements in hardware and software could lead to more efficient and cost-effective deployment of Gemma models.
Ethical Considerations
As with any powerful technology, it’s essential to address the ethical implications of generative models:
- Bias and Fairness: Ensuring that these models are trained on diverse and unbiased data is crucial to mitigate biases in their outputs.
- Misinformation and Disinformation: Safeguards must be put in place to prevent the misuse of generative models to create false or misleading information.
- Intellectual Property: Clear guidelines and regulations are needed to protect intellectual property rights and address issues of copyright infringement.
Google Gemma represents a significant step forward in the field of generative AI. As a powerful and accessible tool, it has the potential to revolutionize various industries and applications. However, it’s crucial to use these models responsibly and ethically, considering the potential risks and biases. By addressing these challenges and continuing to innovate, we can harness the power of generative AI to create a better future.