What Is Generative AI? Understanding Its Capabilities, Applications, and Impact
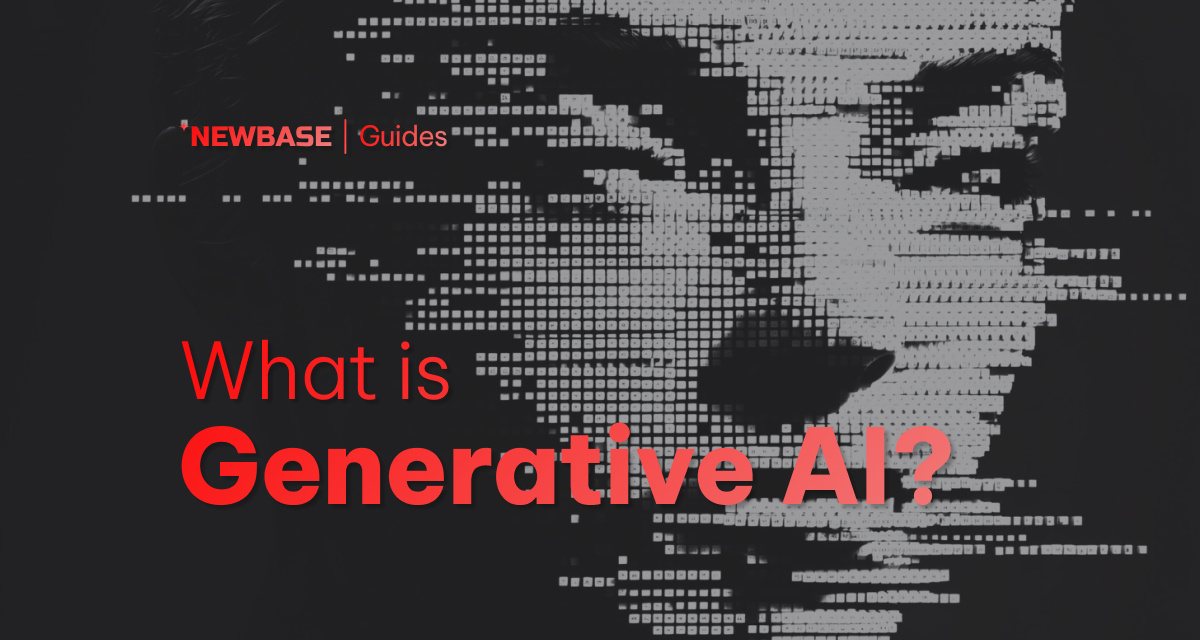
Generative AI, often referred to as Gen AI, represents a revolutionary branch of artificial intelligence capable of creating original content, such as text, images, videos, audio, or even software code, based on a user’s prompt or request. This technology leverages cutting-edge machine learning techniques, particularly deep learning models, to simulate human-like creativity and problem-solving. It serves as a bridge between computation and creativity, transforming industries and redefining the way we interact with technology.
The Mechanics of Generative AI
At the core of generative AI are deep learning models—algorithms inspired by the neural networks of the human brain. These models analyze vast datasets to identify patterns, relationships, and structures. By encoding this information, they can interpret user inputs in natural language and generate meaningful, contextually relevant outputs.
Training the Foundation Model
Generative AI systems rely on foundation models as their base. These models are developed using massive datasets, often spanning terabytes of text, images, or other media. The training process involves predicting the next element in a sequence—whether it’s the next word in a sentence, pixel in an image, or note in a melody. Through countless iterations, the model refines its predictions, ultimately achieving a level of autonomy in content creation.
Training these models is resource-intensive, requiring clusters of GPUs, weeks of computation, and significant financial investment. This scale of training not only underscores the technological sophistication of generative AI but also highlights the barriers to entry for new developers. However, open-source initiatives like Meta’s Llama-2 and OpenAI’s GPT family provide opportunities for smaller organizations and researchers to build upon existing models without incurring prohibitive costs.
Fine-Tuning and Optimization
Once a foundation model is trained, it often undergoes tuning to specialize in specific tasks. Fine-tuning involves feeding the model curated datasets tailored to a particular application, such as customer service or medical diagnostics. Another method, Reinforcement Learning with Human Feedback (RLHF), uses human evaluators to assess and improve the model’s outputs. This method ensures outputs align with user expectations and values, enhancing usability and trustworthiness.
Another enhancement is retrieval-augmented generation (RAG), which integrates real-time external data sources to supplement the model’s knowledge. By combining pre-trained generative capabilities with live data, RAG systems can provide more accurate and contextually updated responses.
Output Generation and Iterative Refinement
Generative AI continually evolves through iterative feedback loops. Users and developers assess the quality of the AI’s outputs, providing feedback that informs further tuning. This iterative process ensures that generative AI systems remain accurate, relevant, and aligned with user expectations. These systems adapt dynamically, becoming more effective and versatile with continued use.
Evolution of Generative AI Architectures
Generative AI has witnessed significant advancements since its inception, with several groundbreaking model architectures shaping its trajectory:
- Variational Autoencoders (VAEs):
Introduced in 2013, VAEs compress data into encoded representations and generate variations of the content. These models found early applications in anomaly detection, image generation, and natural language generation, providing a foundation for subsequent advancements. - Generative Adversarial Networks (GANs):
Debuting in 2014, GANs consist of two competing neural networks—a generator and a discriminator. This adversarial setup fosters the creation of high-quality, realistic outputs, particularly in image and video generation. GANs have revolutionized industries like entertainment, fashion, and media. - Diffusion Models:
Emerging in 2014, these models excel in generating detailed, high-fidelity outputs by iteratively removing noise from data. Tools like OpenAI’s DALL-E leverage diffusion models for image generation, enabling intricate and creative visual outputs. These models are also gaining traction in scientific simulations and complex system designs. - Transformers:
First documented in 2017, transformers revolutionized AI with their attention mechanisms, allowing models to focus on the most relevant aspects of input data. This innovation underpins modern generative AI solutions like GPT-4, Bard, and MidJourney, enabling applications ranging from language understanding to multimedia generation.
Applications of Generative AI
Generative AI has transformative potential across various domains:
- Text Generation:
Models can produce coherent and contextually relevant text, ranging from articles and essays to emails and scripts. They also assist in summarizing, translating, and generating metadata, streamlining workflows across industries. - Image and Video Creation:
Tools like DALL-E and MidJourney can create original artwork, realistic images, and animations. Beyond creative uses, these tools aid in architectural design, medical imaging, and augmented reality applications. - Audio and Music Production:
Generative AI synthesizes lifelike speech for virtual assistants and generates original music compositions, mirroring professional standards. These capabilities are expanding into podcasting, game development, and personalized audio experiences. - Software Development:
AI models automate coding tasks, such as generating code snippets, translating between programming languages, and debugging. This dramatically enhances developer productivity and accelerates software innovation. - Scientific Research and Design:
Generative AI aids in drug discovery by simulating molecular structures, advancing healthcare and material sciences. It also supports the development of renewable energy technologies and innovative material designs.
Benefits of Generative AI
The overarching benefit of generative AI lies in its efficiency. By automating complex and repetitive tasks, it accelerates workflows, reduces costs, and frees up human creativity for higher-value pursuits. Additional advantages include:
- Enhanced Creativity: AI’s ability to generate multiple iterations of content can inspire novel ideas and overcome creative blocks. This democratizes access to creative tools, empowering individuals and organizations alike.
- Improved Decision-Making: Generative AI excels in analyzing large datasets, identifying patterns, and presenting actionable insights. This has profound implications for business intelligence, financial modeling, and strategic planning.
- Personalization: By analyzing user preferences, generative AI can create tailored content for enhanced user experiences. Applications span personalized marketing, adaptive learning platforms, and customized entertainment.
- 24/7 Availability: Unlike human counterparts, AI operates without fatigue, offering round-the-clock support. This is particularly valuable in customer service, healthcare triage, and crisis management.
Challenges and Risks
Despite its promise, generative AI poses significant challenges:
- Hallucinations: AI sometimes generates outputs that are nonsensical or factually incorrect. Implementing guardrails, such as external verification sources and stricter model evaluation protocols, can mitigate this issue.
- Bias: Generative models may inherit societal biases from their training data, leading to unfair or offensive outputs. Addressing this requires diverse datasets, vigilant monitoring, and proactive interventions to ensure fairness.
- Security Threats: AI’s capabilities can be misused to create convincing phishing schemes, fake identities, or deepfakes. This necessitates robust ethical guidelines, detection mechanisms, and legal frameworks to combat misuse.
- Intellectual Property Concerns: Content generated by AI may inadvertently infringe on copyrights, raising legal and ethical questions. Clear regulatory policies and licensing agreements are essential to address these issues.
A Glimpse into the Future
Generative AI is rapidly evolving, with its influence permeating industries and everyday life. From its origins in early machine learning to the transformative models of today, generative AI continues to push the boundaries of creativity and innovation. As organizations and researchers address its challenges, the potential for generative AI to reshape our world remains boundless.
Future developments may include:
- Enhanced collaboration between humans and AI, fostering hybrid creative processes.
- Greater emphasis on ethical AI, ensuring technologies align with societal values.
- Integration of multimodal systems that seamlessly blend text, visuals, and audio.
- Advances in personalization, allowing hyper-tailored user experiences.
Generative AI represents not just a technological leap but a profound shift in how we conceive and realize creativity, problem-solving, and innovation.