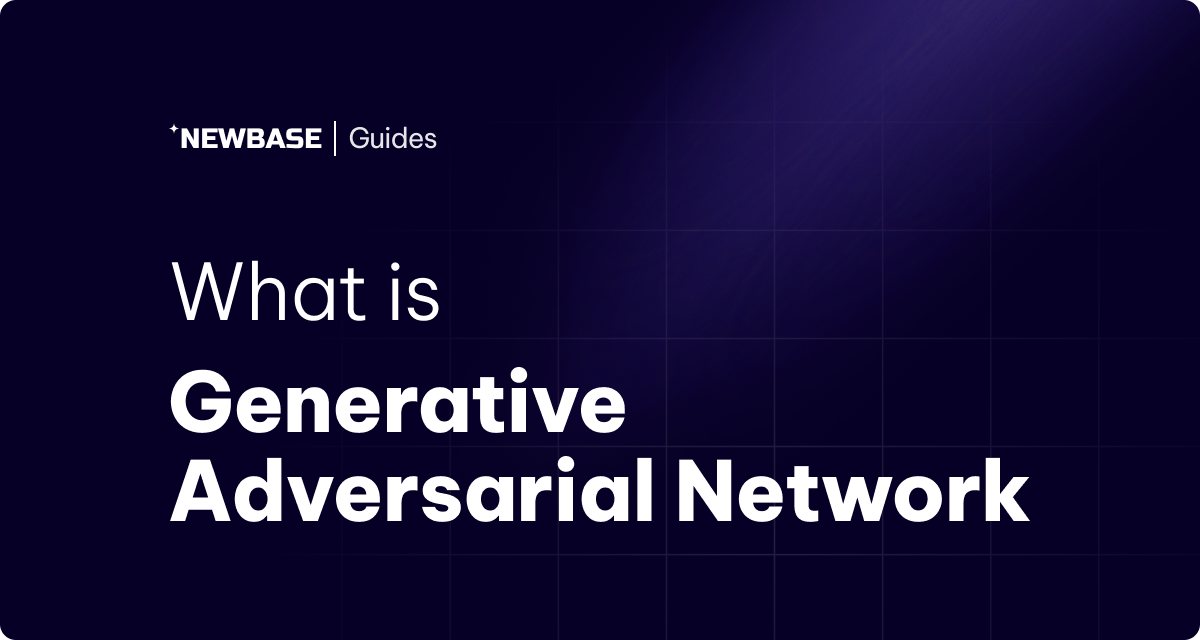
Generative Adversarial Network (GAN, full English name: Generative Adversarial Network) is a deep learning model that has gained great attention in recent years due to its ability to generate high-quality, real-world data. GANs have been used in a wide range of applications, including image synthesis, style transfer, and data augmentation. In this article, we will explore what GANs are, how they work, the advantages of GANs compared to other neural network models, and their main applications and use cases.
What is GAN
Generative adversarial network (GAN) is a type of machine learning model that consists of two neural networks, a generator and a discriminator, which compete with each other in a zero-sum game. The generator creates synthetic data samples, while the discriminator’s job is to distinguish between real and fake data samples. The goal of the generator is to improve its ability to create real data that can “fool” the discriminator, while the goal of the discriminator is to improve its ability to identify fake data.
How GANs work
Generative Adversarial Networks (GAN) consist of two main parts:
Generator
A generator is a neural network that takes random noise as input and generates synthetic data samples (like images, text, etc.) with the goal of creating data that looks identical to the real data distribution.
Discriminator
The discriminator is another neural network that takes as input real data samples and synthetic data samples created by the generator. Its goal is to classify a given sample to determine whether it is real or fake.
The training process of GAN includes the following steps:
- Extract a batch of real data from the data set
- Use a generator to generate a batch of synthetic data
- Train the discriminator on real and synthetic data, updating its weights to improve its ability to distinguish between real and fake samples
- Sample a new batch of random noise and use the generator to generate a new batch of synthetic data
- The generator is trained by backpropagating the gradients of the discriminator, updating the generator’s weights to create more realistic samples that better fool the discriminator.
This training process is repeated until the generator produces real data samples and the discriminator can no longer reliably distinguish between real and fake samples.
Advantages of GAN compared to other neural networks
Compared with traditional neural network models, GANs have some advantages in various tasks:
High-quality data generation
GANs are able to generate high-quality, realistic data samples that are often indistinguishable from real data. This is particularly useful in applications where the availability of real data is limited or costly to acquire.
Unsupervised learning
GANs can learn patterns and features in data without requiring labeled data. This makes them ideal for tasks where annotated data is limited or manual annotation is not feasible.
Flexibility
GANs can be combined with other neural network architectures and techniques, such as convolutional neural networks (CNN) and recurrent neural networks (RNN), to produce a wide variety of data types, including images, text, and audio.
Data augmentation
GANs can generate additional training data for supervised learning tasks, which can help overcome problems related to overfitting and improve the performance of models trained on small data sets.
Main application scenarios of GAN
GAN has been used in various applications in different fields, including but not limited to the following scenarios:
Image synthesis
GANs have been used to generate high-quality synthetic images, such as creating realistic human faces, artwork, and even realistic product scenes.
Style transfer
GANs can be used to transfer the style of one image to another, creating artistic images and novel visual effects.
Data augmentation
GANs can generate additional training data for supervised learning tasks, improving the performance of models trained on small data sets.
Text to image generation
GANs can be used to generate images from text descriptions, which can be useful for generating new visual content or for applications in advertising, entertainment, and design.
Super-resolution and image rendering
GAN can be used to improve the resolution of low-quality images, or to fill in missing areas in the image, improving its overall quality and effectiveness. It is very useful for lossless image enlargement and image repair.
Drug discovery
GANs have been used in the field of drug discovery to generate new chemical structures and identify potential drug candidates.
Anomaly detection
GANs can be used to identify anomalous data points in data sets, which can be helpful in identifying fraud, network intrusions, or other abnormal behavior.
Generative adversarial networks have opened up new possibilities in the field of deep learning and found a wide range of applications. Their ability to generate high-quality, real-world data samples and conduct unsupervised learning makes them incompetent for traditional neural network models. A powerful tool for many tasks