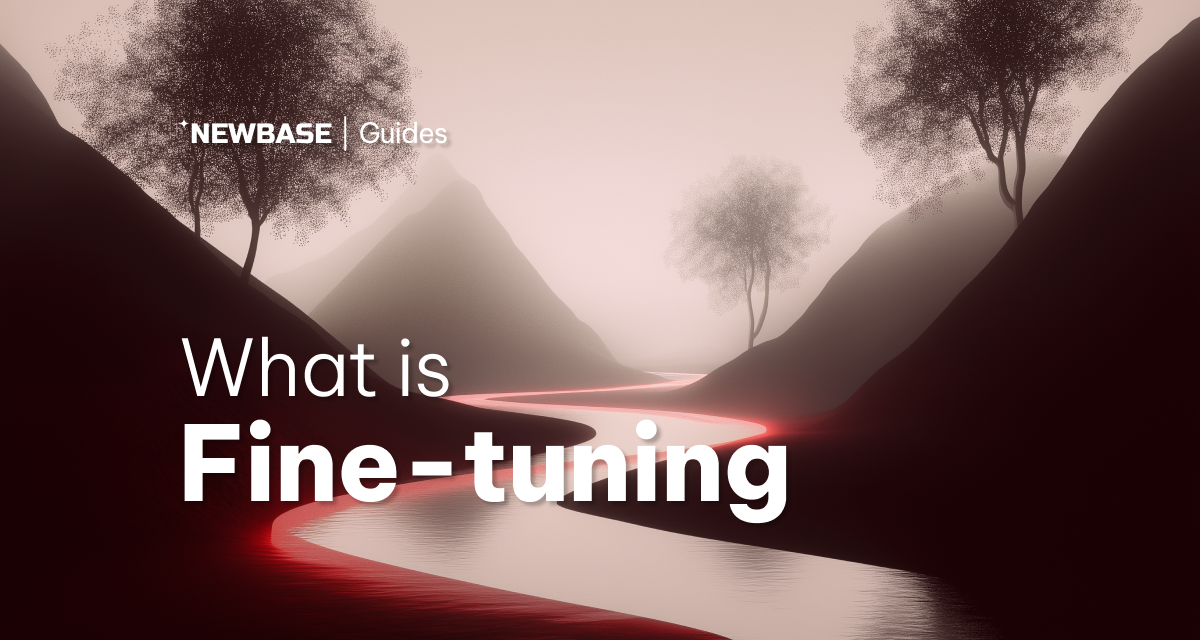
Fine-tuning is a specialized machine learning technique used to adapt pre-trained models for specific tasks or use cases. This approach has become a cornerstone of modern deep learning, particularly in optimizing foundation models that power applications like generative AI, natural language processing (NLP), and computer vision.
By building on the capabilities of existing models, fine-tuning enables organizations to customize and deploy sophisticated AI systems more efficiently, reducing the cost and complexity of model development.
The Concept of Fine-Tuning
Fine-tuning is closely related to transfer learning, a method where knowledge from a pre-trained model is leveraged as a foundation for learning new tasks. Rather than starting from scratch, fine-tuning allows for incremental improvement by adapting a pre-trained model to a specific domain or application.
For instance, large language models (LLMs) like GPT-4 or image-focused models like vision transformers (ViTs) are pre-trained on massive datasets to develop a broad understanding of language or visual patterns. Fine-tuning refines these general capabilities, enabling the model to excel in niche or domain-specific tasks.
Why Fine-Tuning Matters
Developing a machine learning model from scratch, especially one with millions or billions of parameters, is resource-intensive and costly. Fine-tuning offers a more efficient alternative, delivering several key benefits:
- Reduced Computational Costs: Leveraging an existing pre-trained model minimizes the computing power required for training.
- Data Efficiency: Fine-tuning typically requires smaller, more focused datasets compared to the massive datasets used in pre-training.
- Customization: Fine-tuning tailors a model’s outputs to specific business needs, such as adjusting an LLM’s conversational tone or training a vision model to recognize domain-specific objects.
- Access to Advanced Models: Fine-tuning democratizes the use of sophisticated AI systems, allowing organizations without extensive computational resources to benefit from state-of-the-art models.
How Fine-Tuning Works
Fine-tuning involves exposing a pre-trained model to a new dataset that is typically smaller and more focused than the data used during the initial training phase. During this process:
- Pre-Trained Model as a Foundation: The base model retains its general knowledge from pre-training.
- Domain-Specific Data Introduction: The model is trained further using labeled examples from the target domain.
- Parameter Adjustment: The model adjusts its internal parameters to incorporate the new information, refining its predictions and outputs.
For example, a pre-trained LLM might be fine-tuned with a dataset of customer service interactions to better respond to customer queries. Similarly, an image classification model might be fine-tuned with medical imaging data to identify diseases.
Applications of Fine-Tuning
Fine-tuning has a wide range of applications across industries, enabling AI systems to address specific challenges:
- Natural Language Processing (NLP):
- Fine-tuning LLMs for domain-specific text generation, such as legal documents or scientific research.
- Customizing conversational AI to reflect a company’s brand voice or tone.
- Computer Vision:
- Training image recognition models for specialized tasks like medical diagnostics or quality control in manufacturing.
- Adjusting style or characteristics in image generation models for creative industries.
- Generative AI:
- Personalizing content creation tools to align with unique business or artistic styles.
- Enhancing AI models for code generation, music composition, or other creative pursuits.
- Sentiment Analysis and Classification:
- Tuning models for applications like customer feedback analysis, fraud detection, or social media monitoring.
Key Techniques in Fine-Tuning
Fine-tuning methods vary depending on the use case and computational constraints:
- Full Fine-Tuning
- Involves updating all the parameters of the pre-trained model.
- Provides maximum adaptability but requires significant computational resources.
- Parameter-Efficient Fine-Tuning (PEFT)
- Focuses on updating only a subset of the model’s parameters, such as those most relevant to the task.
- Reduces hardware requirements while delivering comparable results.
- Transfer Learning with Specialized Layers
- Adds custom layers on top of the pre-trained model to address domain-specific needs.
- Often used for tasks requiring high precision, like medical or financial applications.
Challenges and Considerations
While fine-tuning offers many benefits, it also presents challenges:
- Overfitting: Training on a small dataset may cause the model to perform well on that data but poorly on new inputs.
- Bias Propagation: If the fine-tuning dataset contains biases, these can be reinforced in the model.
- Resource Requirements: Although less demanding than pre-training, fine-tuning still requires computational resources, particularly for large-scale models.
Mitigating these challenges requires careful dataset selection, rigorous testing, and regular updates to ensure the fine-tuned model remains accurate and unbiased.
Fine-Tuning vs. Pretraining
Fine-tuning complements pretraining but serves a distinct purpose:
- Pretraining: Builds a model’s foundational knowledge using massive, unlabeled datasets.
- Fine-Tuning: Refines the model’s capabilities with smaller, labeled datasets tailored to specific tasks.
For example, an LLM pre-trained on a general corpus of internet text may lack the nuance required for a specialized domain like legal research. Fine-tuning bridges this gap, enabling the model to understand legal terminology and generate accurate legal advice.
Conclusion
Fine-tuning is a powerful tool that unlocks the potential of pre-trained machine learning models, making them more versatile, efficient, and applicable to real-world scenarios. By refining a model’s capabilities with domain-specific data, organizations can deploy advanced AI solutions tailored to their unique needs.
In an era where AI plays a central role in business and innovation, fine-tuning offers a practical and accessible way to harness the power of cutting-edge models while optimizing costs and performance.