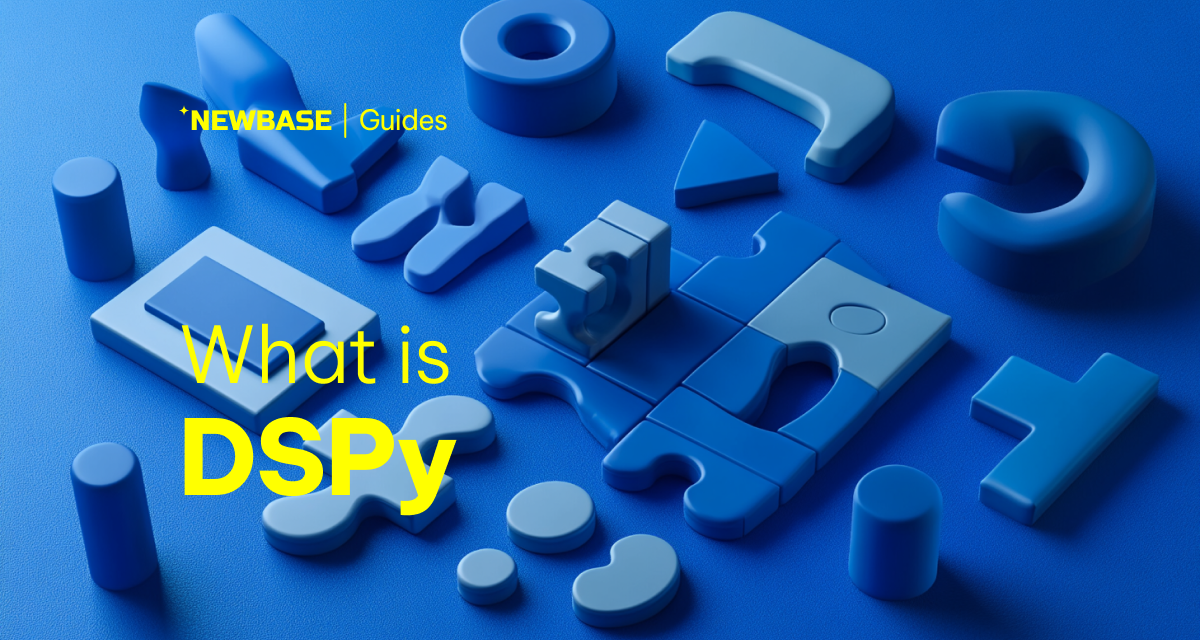
DSPy is a game-changer for large language models (LLMs). It’s a framework that automates prompt optimization, replacing the time-consuming and error-prone process of manual prompt engineering.
Imagine building an LLM application – traditionally, you’d meticulously craft prompts to get the desired output. DSPy streamlines this by:
- Replacing Prompt Engineering: Instead of manually tweaking prompts, DSPy uses general-purpose modules and optimizes them with code.
- Automating Prompt Creation: DSPy harnesses LLMs to generate prompts, then tests them for effectiveness through evaluation metrics.
- General-Purpose Modules: These modules offer flexibility, adapting to various tasks like retrieval-augmented generation or question answering.
Why Use DSPy?
Beyond efficiency, DSPy offers several advantages:
- Reduced Errors: Manual prompt engineering is prone to errors. DSPy’s automated approach minimizes this risk.
- Improved Performance: By optimizing prompts, DSPy can potentially lead to better LLM performance on specific tasks.
- Flexibility: DSPy’s modular design allows for building complex pipelines for various LLM applications.
Working with DSPy
Here’s a glimpse into how DSPy works:
- Concepts: DSPy has its own terminology, including “compiling” (translating Python code into LLM instructions) and “signatures” (defining input/output types of modules).
- Optimizers: These fine-tune DSPy programs for specific LLMs like GPT-3.5 or Llama 3.1, maximizing performance and accuracy.
- Demonstrations: Similar to few-shot examples, demonstrations are used to guide prompt creation and selection.
- Metrics: DSPy offers metrics like Semantic F1 to measure performance. You can also define custom metrics.
Building a DSPy Pipeline
Here’s a simplified view of building a retrieval-augmented generation pipeline with DSPy:
- Define Signatures: These templates specify input/output structures for your language model and retrieval model.
- Compiling: This step creates optimal prompts for your task using an appropriate optimizer. It involves:
- Training data or bootstrapped examples.
- Validation metric (e.g., answer accuracy).
- Specific optimizer (e.g., BootstrapFewShot).
- Evaluation and Iteration: After compilation, you can evaluate the results and iterate by adjusting data, program structure, metrics, or the chosen optimizer.
Getting Started with DSPy
DSPy is open-source and requires no special hardware. You can run it locally, on cloud platforms, or on hosted notebook environments like Google Colab. The StanfordNLP GitHub repository provides comprehensive documentation and tutorials to get you started.
In essence, DSPy empowers developers to create more robust and efficient LLM applications by automating prompt optimization and offering a modular approach to building complex pipelines.