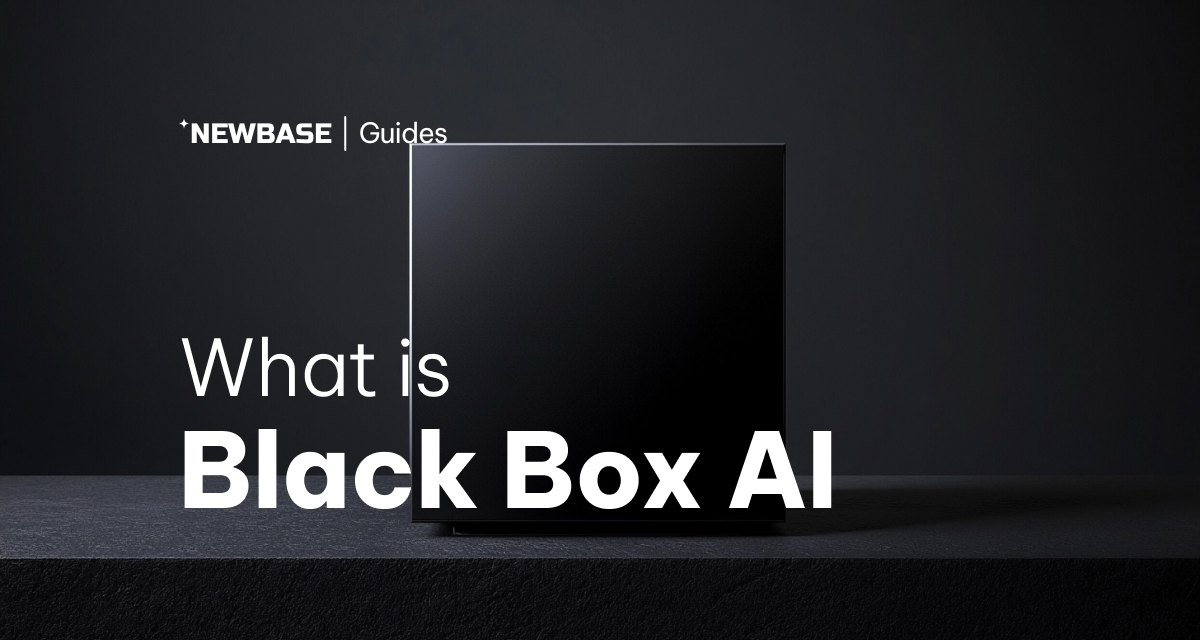
Black box AI refers to artificial intelligence systems whose internal workings remain a mystery to their users. While users can observe the inputs and outputs of the system, the intricate processes behind those outputs are shrouded in obscurity. Imagine a black box that analyzes job applications; you can feed it resumes (inputs) and receive assessments (outputs), but you wouldn’t know the specific criteria the model uses for evaluation.
The Rise of Black Boxes: Intentional or Incidental?
Black box AI can emerge in two ways: intentional design or an unintended consequence of training. Some developers intentionally conceal the inner workings of their AI tools to protect intellectual property. This is often the case with traditional, rule-based algorithms where the source code and decision-making logic remain a secret.
However, the most advanced AI technologies, like generative models, are a different breed. These “organic black boxes” arise from the immense complexity of deep learning. Deep learning algorithms leverage multi-layered neural networks, where each layer contains artificial neurons mimicking the human brain. These networks can process massive amounts of raw data, identify patterns, and generate outputs like images, text, and videos.
The black box nature arises from the “hidden layers” within the deep neural network. While users can see the input and output layers, the intermediate layers remain opaque. Developers have a general understanding of how data flows through these layers, but the exact details – how specific neuron combinations activate or how vector embeddings are used – remain elusive. Even for open-source models sharing their underlying code, interpreting what happens within each layer during operation is a challenge.
The Black Box Problem: Power with a Price
While black box AI models are incredibly powerful, their lack of transparency comes at a cost. These advanced models, particularly generative AI, are difficult to understand due to the complex neural networks involved. Simpler, explainable models exist, but they often lack the power and flexibility of black boxes. Organizations, therefore, face a trade-off: leverage the power of black boxes or prioritize explainability with less powerful alternatives.
The Dark Side of Black Boxes: Challenges and Risks
The lack of transparency in black box AI poses several challenges:
- Reduced Trust in Model Outputs: Without understanding the decision-making process, even seemingly accurate outputs can be difficult to validate. This is akin to the “Clever Hans effect,” where a horse supposedly counted using hoof stomps, but in reality, responded to subtle cues from its owner. In healthcare, an AI model trained to diagnose COVID based on X-rays might learn to identify irrelevant factors like annotations on the image rather than the actual disease.
- Difficulty Adjusting Models: If a black box model makes consistently inaccurate or harmful decisions, it can be hard to pinpoint the root cause and correct its behavior. This poses a significant challenge in autonomous vehicles, where understanding why an AI makes a bad decision is crucial for safety improvements.
- Security Issues: Hidden vulnerabilities can lurk within black box models, leaving them susceptible to attacks like prompt injection (manipulating the input to steer the outcome) or data poisoning (altering training data to subtly change behavior).
- Ethical Concerns: Black box models can perpetuate bias if present in the training data or design. For instance, an AI model screening job candidates might filter out talented women if the training data skews male-dominated. This lack of transparency makes it difficult to identify and address bias.
- Regulatory Noncompliance: Regulations like the EU AI Act or the CCPA govern how sensitive data is used in AI decision-making. Black box models can make it difficult for organizations to demonstrate compliance during audits.
Beyond the Black Box: Towards Explainable AI
Researchers are actively seeking ways to make these advanced models more explainable. Techniques like autoencoders (a type of neural network) are being explored to understand which neuron combinations correspond to specific concepts. OpenAI’s o1 model attempts to explain its reasoning behind outputs, albeit through a model-generated explanation, not a direct peek into its inner workings. Additionally, methods like LIME (local interpretable model-agnostic explanation) analyze the relationships between black box inputs and outputs to identify influential features.
The Road Ahead: Balancing Power and Transparency
Black box AI systems are powerful tools with a crucial role to play in various fields. However, addressing the challenges associated with their opacity is essential. As research into explainable AI progresses, we can hope for a future where powerful AI models are not just effective but also transparent and trustworthy.
The Future of Black Box AI
While the black box nature of many AI systems poses significant challenges, researchers and developers are actively working on solutions. Key areas of focus include:
- Explainable AI (XAI): XAI techniques aim to make AI models more interpretable. These techniques can help to uncover the decision-making processes of models, making them more transparent and accountable.
- Model Interpretability Tools: Tools like LIME (Local Interpretable Model-Agnostic Explanations) and SHAP (SHapley Additive exPlanations) can help visualize the factors that contribute to a model’s predictions.
- Ethical AI Frameworks: Developing ethical guidelines and frameworks can help ensure that AI is used responsibly and ethically. This includes addressing issues like bias, fairness, and transparency.
- Regulation and Governance: Governments and regulatory bodies are increasingly focusing on AI regulation to ensure that AI systems are safe, reliable, and accountable.
As AI continues to evolve, it is essential to strike a balance between innovation and transparency. By understanding the limitations and potential risks of black box AI, we can work towards developing more responsible and accountable AI systems.