What is Artificial Intelligence (AI)? A Deep Dive into the Essence of Artificial Intelligence
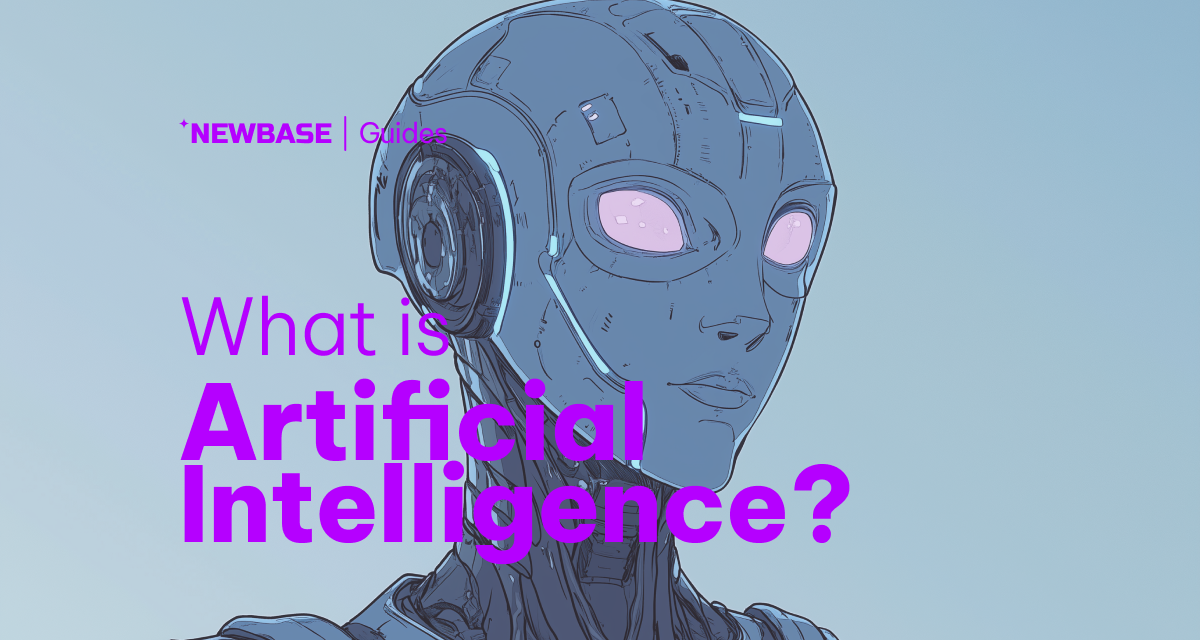
Artificial Intelligence (AI) is a groundbreaking branch of computer science that enables machines to perform tasks typically requiring human intelligence. These tasks range from learning and problem-solving to decision-making, creativity, and autonomy. Over the decades, AI has evolved to become a cornerstone of modern technology, influencing industries and reshaping everyday life.
Core Capabilities of AI
AI systems are designed to replicate human capabilities through advanced algorithms and computational models. These systems can:
- Recognize Objects: Identify and classify images, objects, or patterns with precision, enabling applications like facial recognition and medical imaging analysis.
- Understand Language: Process and respond to human speech or text through natural language processing (NLP), such as in chatbots and virtual assistants.
- Learn from Data: Adapt and improve performance by analyzing large-scale data, making predictions, and generating insights.
- Make Recommendations: Provide personalized suggestions based on analyzed patterns and user behavior, widely used in e-commerce and streaming platforms.
- Act Autonomously: Perform tasks independently, exemplified by technologies like self-driving cars and robotic process automation (RPA).
Generative AI: The 2024 Focus
In 2024, much attention in AI is directed toward generative AI (gen AI). This subset of AI creates original content—text, images, videos, and more—using advanced deep learning models. Generative AI is reshaping creativity, innovation, and problem-solving across industries.
To fully grasp generative AI, it’s essential to understand the foundational technologies it builds upon: machine learning (ML) and deep learning.
Machine Learning (ML)
Machine learning is a subset of AI involving the creation of models trained to make predictions or decisions based on data. ML allows systems to learn and improve without explicit programming for specific tasks.
Types of Machine Learning Algorithms
- Supervised Learning: Models are trained on labeled datasets to predict outcomes or classify data, such as in spam detection or medical diagnosis.
- Unsupervised Learning: Algorithms uncover hidden patterns in unlabeled data, enabling applications like customer segmentation and anomaly detection.
- Reinforcement Learning: Models learn through trial and error, optimizing actions based on rewards, used in robotics and game AI.
- Semi-Supervised Learning: Combines labeled and unlabeled data for training, striking a balance between resource use and accuracy.
Popular ML Techniques:
- Linear and logistic regression for predictive analytics
- Decision trees and random forests for classification tasks
- Support vector machines (SVMs) for data separation
- Neural networks (basic and advanced) for complex pattern recognition
Deep Learning
Deep learning, a specialized branch of ML, leverages multilayered neural networks called deep neural networks. These networks emulate the human brain’s complexity, enabling intricate data analysis and decision-making.
Characteristics of Deep Learning:
- Multilayered Architecture: Includes input, hidden, and output layers, with the hidden layers often numbering in the hundreds.
- Feature Extraction: Automates identifying significant patterns in large, unstructured data sets.
- Scalable Processing: Handles vast amounts of data with minimal human intervention.
Deep learning excels in areas like NLP, computer vision, and predictive analytics, empowering applications such as real-time language translation and advanced image recognition systems.
Generative AI: Creation and Evolution
Generative AI refers to systems capable of producing original, high-quality content. It has evolved through groundbreaking advancements in deep learning models:
- Variational Autoencoders (VAEs): Generate diverse variations of content, such as creating new product designs or image filters.
- Diffusion Models: Transform noise into coherent images, widely used in art generation and scientific imaging.
- Transformers: Power tools like ChatGPT, MidJourney, and Bard by generating sequential content (e.g., text, video frames).
Workflow of Generative AI
- Training: A foundation model is created by training on massive datasets, ensuring a wide knowledge base.
- Tuning: The model is refined for specific applications using methods like fine-tuning and reinforcement learning with human feedback (RLHF).
- Generation and Evaluation: Outputs are evaluated and iteratively improved for better accuracy and creativity.
Benefits of AI
AI provides transformative advantages across industries, including:
- Automation: Reduces repetitive tasks, freeing humans for creative endeavors and strategic roles.
- Enhanced Decision-Making: Improves accuracy with data-driven insights, essential in finance, healthcare, and logistics.
- 24/7 Availability: Delivers consistent performance around the clock, enhancing customer service and operational efficiency.
- Error Reduction: Minimizes human errors, particularly in critical sectors like healthcare and aviation.
- Increased Safety: Automates dangerous tasks, reducing risks to human workers, such as in mining and hazardous material handling.
Use Cases of AI
AI’s versatility is evident in its applications across numerous fields:
- Customer Support: AI chatbots provide instant, consistent responses, improving user satisfaction.
- Fraud Detection: Identifies anomalies in financial transactions to mitigate risks, safeguarding businesses and consumers.
- Personalized Marketing: Tailors recommendations and campaigns to individual preferences, boosting engagement.
- Human Resources: Streamlines recruitment with AI-driven tools, optimizing candidate matching and onboarding processes.
- Predictive Maintenance: Anticipates equipment failures, optimizing operational efficiency and reducing downtime.
- Healthcare: Enhances diagnostics, patient care, and drug discovery through intelligent analysis.
Challenges and Risks in AI
Despite its benefits, AI adoption comes with challenges:
- Data Vulnerabilities: Risks include data bias, tampering, and breaches, which can undermine trust and effectiveness.
- Model Integrity: Threat actors might manipulate AI models, leading to malicious outcomes.
- Operational Risks: Issues like model drift or governance lapses can disrupt performance and reliability.
- Ethical Concerns: Bias in training data can lead to discriminatory outcomes, posing societal challenges.
AI Ethics and Governance
Ethical AI development ensures alignment with societal values. Key principles include:
- Explainability: Enhances transparency in AI decision-making, fostering trust and accountability.
- Fairness: Reduces bias through diverse, inclusive model design, ensuring equitable outcomes.
- Accountability: Establishes clear governance and responsibilities for developers and organizations.
- Privacy Compliance: Adheres to regulations like GDPR, safeguarding user data and privacy rights.
Weak AI vs. Strong AI
AI is categorized based on complexity:
- Weak AI: Designed for specific tasks (e.g., Siri, Alexa), offering efficiency and reliability in constrained domains.
- Strong AI: Hypothetical systems capable of human-level intelligence and generalization, a goal still under research.
A Brief History of AI
AI’s journey spans decades of innovation:
- 1950: Alan Turing introduces the Turing Test, a foundational concept in AI theory.
- 1956: Term “AI” coined by John McCarthy during the Dartmouth Conference.
- 1997: IBM’s Deep Blue defeats world chess champion Garry Kasparov, marking a milestone in AI capability.
- 2011: IBM Watson wins Jeopardy!, showcasing advanced NLP and data analysis.
- 2024: Advances in multimodal and efficient AI models redefine industry standards, emphasizing generative capabilities.
Artificial Intelligence stands as a transformative force in the modern world. With its continuous evolution, AI holds the promise of solving complex challenges, streamlining processes, and unlocking unparalleled opportunities for innovation.