What is an AI Model? The Building Blocks of AI: A Guide to AI Models
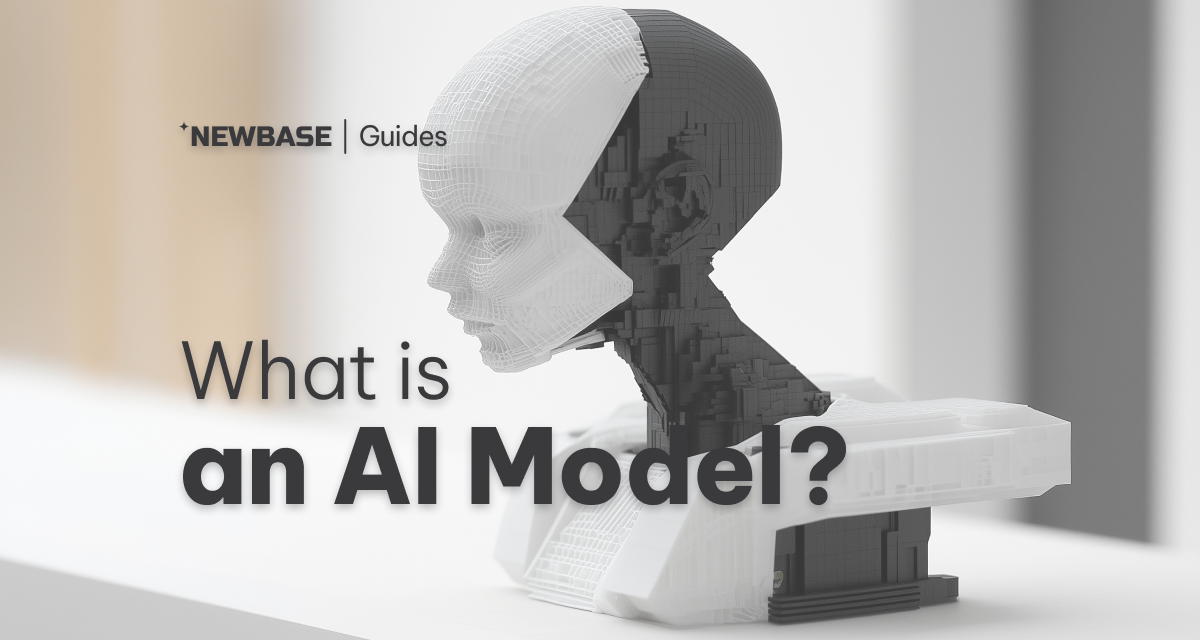
An AI model is a computer program that has been trained on a set of data to recognize patterns, make predictions, or perform specific tasks without further human intervention. These models are the backbone of modern artificial intelligence systems, utilizing algorithms and vast datasets to perform functions like image recognition, language processing, and decision-making.
At its core, an AI model autonomously makes decisions or predictions rather than merely simulating human logic. Early examples include the checkers- and chess-playing programs from the 1950s, which could react to an opponent’s moves instead of following a fixed series of pre-programmed steps.
Types of AI Models
Different types of AI models are optimized for specific tasks or domains, depending on their logic and design. Complex AI systems often integrate multiple models using techniques like ensemble learning (e.g., bagging, boosting, and stacking) to improve overall performance.
- Rule-based AI models: These models operate using explicit “if-then-else” rules programmed by data scientists. Examples include expert systems and symbolic AI.
- Machine Learning (ML) models: These models can learn from data and improve over time without being explicitly programmed. ML models use statistical methods to analyze and make predictions.
- Deep Learning models: A subset of machine learning, deep learning models use neural networks with multiple layers to process data in a way that mimics the human brain. These models power advanced AI systems like large language models (LLMs).
Algorithms vs. Models
The terms “algorithm” and “model” are often used interchangeably, but they have distinct meanings:
- Algorithms: These are step-by-step instructions or procedures, often in mathematical form, that process data to achieve a certain goal.
- Models: These are the outcomes of applying algorithms to datasets. A model is essentially the functional product that can make predictions or decisions based on input data.
In essence, the algorithm defines the process, while the model is the result of that process applied to training data.
Machine Learning Model Categories
Machine learning (ML) models can be classified based on the learning approach they use:
- Supervised Learning: The model is trained on labeled data. Examples include image classification and spam detection. The training data contains input-output pairs, enabling the model to learn relationships between inputs and outputs.
- Unsupervised Learning: The model identifies patterns and relationships in data without pre-existing labels. This approach is used for clustering and anomaly detection.
- Reinforcement Learning: The model learns by trial and error, receiving rewards for correct actions and penalties for incorrect actions. Applications include robotics, self-driving cars, and game-playing AI.
Types of AI Models by Function
- Generative Models: These models generate new data points similar to the input data. Examples include:
- Diffusion Models: Used to create images by reversing noise.
- Variational Autoencoders (VAEs): Learn to reconstruct input data, often used for anomaly detection.
- Transformer Models: Utilize self-attention to understand and generate text, as seen in language models like GPT.
- Discriminative Models: These models classify data points by predicting labels. They establish decision boundaries between classes and are used for classification and regression tasks.
- Logistic Regression: Predicts binary outcomes like spam/not spam.
- Support Vector Machines (SVMs): Identify decision boundaries between categories.
- Decision Trees and Random Forests: Split data into branches based on feature importance to make classification decisions.
Classification Models vs. Regression Models
- Classification Models: Predict discrete categories (e.g., “spam” or “not spam”). Examples include Naïve Bayes classifiers, decision trees, and support vector machines (SVMs).
- Regression Models: Predict continuous values (e.g., price, temperature). Examples include linear regression, polynomial regression, and autoregressive models.
Training AI Models
Training an AI model involves feeding it sample data to optimize its parameters. The goal is for the model to generalize well to new, unseen data. Training methods vary depending on the type of model and learning technique (supervised, unsupervised, or reinforcement).
- Feature Extraction: Key features are selected from raw data, which the model can then analyze and learn from.
- Data Preparation: Data is cleaned, normalized, and split into training, validation, and testing sets.
- Model Training: The model’s weights and biases are adjusted iteratively to minimize prediction errors.
- Validation and Testing: The model’s performance is tested on unseen data to ensure it generalizes well and avoids overfitting.
Foundation Models
Foundation models, also known as pre-trained models, are large models trained on vast, diverse datasets. These models can be fine-tuned for specific tasks, saving time, cost, and computational resources. Instead of training models from scratch, developers can fine-tune foundation models like OpenAI’s GPT or Google’s BERT for specialized use cases.
Model Testing and Metrics
Testing an AI model’s performance ensures that it meets the intended objectives. The type of model and task determines the testing methods and metrics used.
- Cross-Validation: Splitting the training data into subsets to validate the model’s performance.
- Classification Model Metrics:
- Accuracy: The ratio of correct predictions to total predictions.
- Precision: The ratio of true positives to total predicted positives.
- Recall: The ratio of true positives to the total actual positives.
- F1 Score: A balance between precision and recall.
- Regression Model Metrics:
- Mean Absolute Error (MAE): Average of absolute errors.
- Mean Squared Error (MSE): Average of squared errors, penalizing large errors.
- Root Mean Square Error (RMSE): Standard deviation of prediction errors.
- Mean Absolute Percentage Error (MAPE): Average error expressed as a percentage.
Model Deployment
Deploying an AI model involves making it available for real-world use. Deployment requires sufficient computing power, typically provided by CPUs, GPUs, or cloud platforms. The complexity of modern AI models, particularly large language models, often necessitates the use of high-performance GPUs.
- Machine Learning Frameworks: Tools like TensorFlow, PyTorch, and Scikit-learn make it easier to train, deploy, and run models.
- Deployment Tools: Platforms like AWS SageMaker, Google Cloud AI, and Microsoft Azure AI enable scalable deployment.
Challenges in AI Modeling
- Data Bias: Models trained on biased data will produce biased outcomes. Fairness-aware algorithms like FairIJ help mitigate this issue.
- Overfitting: When a model learns too well from its training data, it fails to generalize to new data.
- Underfitting: When a model is too simple, it performs poorly even on training data.
Conclusion
AI models are essential for automating decision-making, prediction, and analysis tasks. By understanding the different types of models, training methods, and performance metrics, developers can create and deploy AI systems that drive innovation in fields like healthcare, finance, and autonomous systems. The rise of foundation models further accelerates AI development by allowing pre-trained models to be fine-tuned for specialized applications, reducing training time and costs.