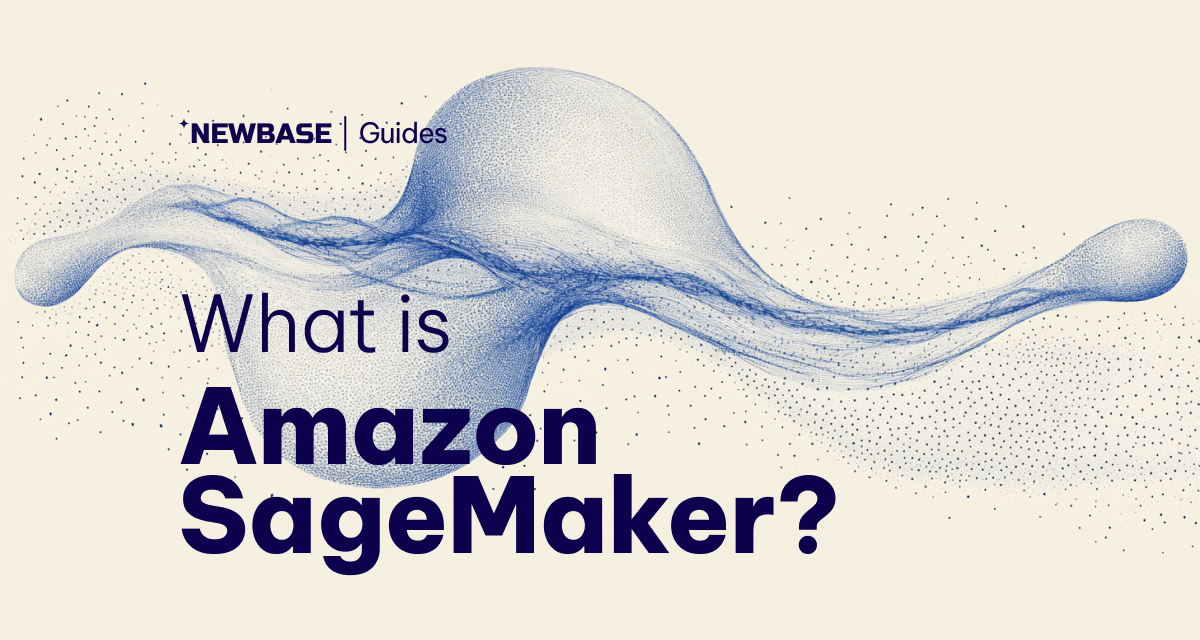
Empowering Your Machine Learning Journey
Amazon SageMaker is a comprehensive, fully-managed service designed to streamline the entire machine learning (ML) lifecycle. Offered by Amazon Web Services (AWS), SageMaker simplifies the process of building, training, and deploying ML models, accelerating innovation and maximizing the value derived from your data.
This article delves into the core functionalities of SageMaker, explores its benefits, and highlights use cases across various industries. We’ll also explore how SageMaker integrates with broader AI governance strategies, ensuring responsible and compliant development of your machine learning applications.
Understanding the Machine Learning Process
Before diving into SageMaker’s capabilities, let’s revisit the fundamental stages of machine learning:
- Decision Process: ML algorithms analyze data, labeled or unlabeled, to identify patterns and make predictions.
- Error Function: This function evaluates the model’s accuracy by comparing its predictions to known examples, pinpointing areas for improvement.
- Model Optimization: The algorithm iteratively adjusts its internal parameters based on the error function, striving for ever-increasing accuracy.
SageMaker simplifies these processes, empowering data scientists to efficiently develop and deploy machine learning models.
How Does AWS SageMaker Work?
SageMaker offers a structured approach to the ML lifecycle, encompassing three critical phases:
Data Preparation:
- Gather and clean real-world datasets.
- Utilize Amazon Ground Truth for labeled synthetic image data (optional).
- Upload prepared data to Amazon Simple Storage Service (S3) for easy access.
Training:
- Leverage pre-built SageMaker notebook instances for efficient data processing.
- Import personal tools or use pre-built notebooks with essential libraries for popular deep learning frameworks.
- Train models using your own algorithms or choose from a pre-built selection via the SageMaker console.
- Fine-tune pre-trained models to fit specific datasets and tasks.
- Optimize large language models (LLMs) with SageMaker’s hyperparameter tuning.
- Monitor model performance and resource usage with the Debugger.
Deployment:
- SageMaker automatically manages and scales the underlying cloud infrastructure for seamless deployment.
- Utilize multiple availability zones for enhanced reliability.
- Leverage secure HTTPS endpoints for robust application connectivity.
- Monitor production performance with Amazon CloudWatch metrics and set alerts for deviations.
Benefits of Amazon SageMaker
SageMaker streamlines the ML development process with a multitude of advantages:
- Integrated Development Environment (IDE): SageMaker Studio provides a comprehensive IDE for managing workflows, developing models, and visualizing metrics.
- Model Training and Optimization: Train models with built-in or custom algorithms, leveraging popular frameworks like TensorFlow, PyTorch, and MXNet. Fine-tune pre-trained models for specific tasks.
- Data Preparation and Labeling: Utilize Ground Truth for high-quality labeled datasets and manage features across models with the built-in feature store.
- Real-time and Batch Inference: Deploy models for real-time predictions or batch processing through efficient endpoints.
- Serverless and Cost-Effective Solutions: Leverage serverless capabilities with auto-scaling and AWS Lambda integration for optimized costs and scalability.
- Monitoring and Debugging: Monitor model performance with CloudWatch and utilize debugging features for robust ML lifecycles.
- Flexible Pricing Models: Choose between on-demand and pay-as-you-go pricing based on usage. Explore the free tier for initial exploration.
Amazon SageMaker Use Cases
The versatility of SageMaker empowers various industries:
- Healthcare: Analyze patient data for predicting outcomes, personalized treatments, and operational efficiency enhancements.
- Finance: Develop models for fraud detection, credit scoring, and risk assessment.
- Retail: Leverage predictive analytics for improved inventory management, personalized customer experiences, and optimized pricing strategies.
AI Governance with Amazon SageMaker
SageMaker prioritizes responsible development by providing tools for AI governance and regulatory compliance:
- Identity and Access Management (IAM): Control permissions and roles, ensuring only authorized users access sensitive data and models.
- Version Control: Track model versions and configurations for a clear audit trail.
- Model Registry: Manage model artifacts and metadata for transparency and accountability throughout development.
Integration and Collaboration
SageMaker seamlessly integrates with existing workflows and services through the SageMaker Python SDK. This allows for automated compliance checks and enhanced oversight across ML projects.
Strategic partnerships between IBM and AWS further bolster capabilities. Organizations can leverage IBM’s foundation models alongside SageMaker for advanced analytics, improved data management, and streamlined workflows. Additionally, deploying models within an Amazon VPC ensures secure and controlled access to resources.
The Future of Amazon SageMaker
As the field of machine learning continues to evolve, Amazon SageMaker remains at the forefront of innovation. Here are some potential future developments:
- Enhanced Automation: SageMaker may further automate tasks like feature engineering, model selection, and hyperparameter tuning, making it even easier for data scientists to build and deploy models.
- Advanced AI Capabilities: Integration with cutting-edge AI technologies like generative AI and reinforcement learning could provide new capabilities for model development and deployment.
- Improved Explainability: SageMaker might offer tools to enhance the interpretability of models, making it easier to understand how models arrive at their decisions.
- Enhanced Security and Compliance: As data privacy and security become increasingly important, SageMaker may introduce more robust security features and compliance certifications.
- Expanded Ecosystem: SageMaker could expand its ecosystem of integrations with other AWS services and third-party tools, providing even greater flexibility and customization options.
Conclusion
Amazon SageMaker is a powerful tool that simplifies the machine learning process, from data preparation to model deployment. By leveraging its features, organizations can accelerate their AI initiatives, reduce costs, and drive innovation. As SageMaker continues to evolve, it will likely play an even more significant role in shaping the future of machine learning.