What is Algorithmic Bias? Understanding Causes, Risks, and Mitigation Strategies
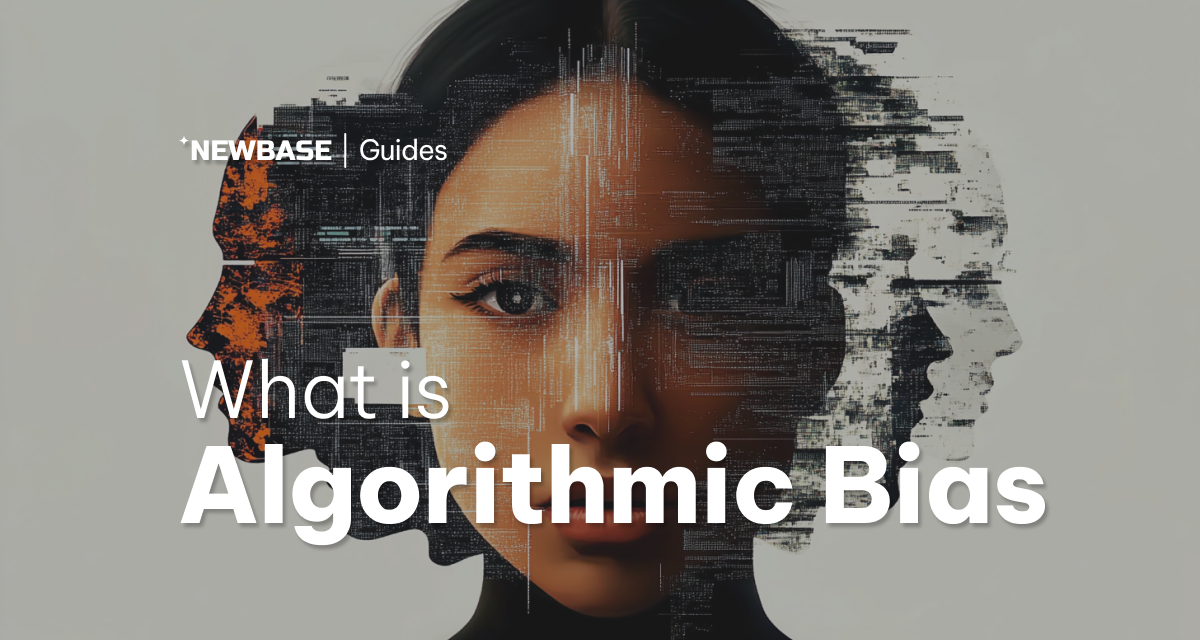
Algorithmic bias occurs when systematic errors in machine learning algorithms lead to unfair, discriminatory, or prejudiced outcomes. It reflects or even amplifies existing socioeconomic, racial, gender, or other societal biases. This phenomenon has emerged as one of the most pressing challenges in the development and deployment of Artificial Intelligence (AI) systems, particularly as AI plays an increasingly pivotal role in decision-making across sectors like healthcare, criminal justice, recruitment, and finance. The risks associated with algorithmic bias are multifaceted, ranging from perpetuating inequality to creating legal, financial, and reputational consequences for businesses.
At its core, algorithmic bias arises not from the algorithms themselves but from the data used to train them, as well as the design choices and evaluation processes involved in their development. The biases embedded within the data—whether historical, cultural, or social—can shape the model’s predictions and decisions, making them unfair or discriminatory. As AI continues to permeate high-stakes fields, understanding and mitigating algorithmic bias has become crucial for ensuring fairness, accountability, and trust in these technologies.
What Causes Algorithmic Bias?
Algorithmic bias is primarily driven by human involvement at various stages of the AI development process. It is not inherently an issue of flawed algorithms but a product of how data is collected, interpreted, and modeled. Here are the key causes of algorithmic bias:
- Biases in Training Data
Training data is the foundation upon which machine learning models are built. If the data used to train an AI system is flawed—whether due to underrepresentation, misrepresentation, or historical bias—the resulting model will likely inherit these flaws. For instance, if an AI system is trained on historical data where marginalized communities have been systematically underrepresented or misrepresented, the AI might make biased decisions that unfairly disadvantage these groups. - Biases in Algorithm Design
Developers’ subjective decisions during the design and development of algorithms can introduce biases. For example, if certain factors are unfairly weighted during the decision-making process, it can distort the model’s output. Bias can also arise if an algorithm is programmed with assumptions that unintentionally favor one group over another. These biases might be conscious, stemming from personal prejudices, or unconscious, emerging from unexamined norms and practices within a development team. - Biases in Proxy Data
Proxy data refers to data points that stand in for protected attributes like race, gender, or age, which should not be used in decision-making to prevent discrimination. However, proxies can be biased if they correlate with sensitive attributes, even if unintentionally. For example, using postal codes as a proxy for socioeconomic status can lead to racial bias if certain neighborhoods have predominantly Black or Hispanic populations and are unfairly disadvantaged in the model’s predictions. - Biases in Evaluation
Even when the algorithm itself is neutral, biases can creep into the system during evaluation. How the algorithm’s results are interpreted—by individuals or organizations—can influence decisions in ways that perpetuate biases. For example, human evaluators may interpret outputs in ways that align with preexisting biases, leading to outcomes that unfairly affect certain groups. - Flawed Data
Data that is incomplete, outdated, or poorly collected can introduce flaws into AI models. This can include data that lacks representation of certain groups or is based on outdated assumptions about populations. When AI systems make predictions based on flawed data, the results can reinforce stereotypes or discriminatory practices.
The Risks of Algorithmic Bias
When algorithmic bias is left unaddressed, it can lead to numerous harmful consequences. These range from social and ethical issues to legal, financial, and reputational risks for businesses. Below are some of the primary risks associated with algorithmic bias:
- Discrimination and Inequality
One of the most damaging effects of algorithmic bias is the reinforcement of discrimination and inequality. Biased algorithms perpetuate the systemic disadvantages faced by marginalized communities, which can exacerbate societal divides. For example, in predictive policing, algorithms that rely on historical arrest data can disproportionately target minority communities, perpetuating cycles of over-policing and racial inequality. - Legal and Reputational Damage
Organizations that use biased AI systems risk facing legal actions, financial penalties, and reputational damage. Many jurisdictions have antidiscrimination laws that prevent organizations from making biased decisions, and biased AI systems can inadvertently violate these laws. For example, if a hiring algorithm systematically discriminates against women or minorities, the company could face lawsuits for disparate impact, potentially leading to substantial financial liabilities and long-term damage to its public image. - Erosion of Trust
Trust is crucial to the adoption of AI technologies. Algorithmic bias can significantly erode trust, not only in AI systems themselves but also in the institutions that deploy them. If AI is seen as inherently biased or unfair, both users and stakeholders may lose confidence in its ability to make impartial decisions. This can be particularly damaging for businesses that rely on AI tools to maintain their competitiveness or for public institutions whose credibility is tied to fairness and equity.
Real-World Examples of Algorithmic Bias
Algorithmic bias has been observed in a range of real-world applications, many of which have significant social implications:
- Bias in Criminal Justice
The COMPAS tool, used in the U.S. court system to assess the likelihood of recidivism, was found to disproportionately classify Black defendants as high-risk compared to white defendants. This is a striking example of how biased historical data—such as arrest records—can lead to unjust outcomes that exacerbate racial disparities within the justice system. - Bias in Predictive Policing
Predictive policing tools have been shown to target neighborhoods with higher populations of people of color. These systems often rely on historical crime data, which can reflect existing racial biases. For instance, an algorithm trained on crime reports may inaccurately predict more criminal activity in areas where Black individuals are more frequently arrested, reinforcing a cycle of over-policing in these communities. - Bias in Healthcare
AI algorithms used in healthcare, such as those for diagnosing diseases or predicting patient outcomes, have been found to perform less accurately for minority groups. This is often due to a lack of representation of these groups in training datasets. In one case, AI systems used to prioritize patients for organ transplants were less likely to recommend Black patients, leading to disparities in healthcare access. - Bias in Recruitment
Amazon faced significant backlash when its AI-based recruitment tool was found to be biased against female candidates. The tool was trained on resumes from past hires, who were predominantly male, and it learned to favor resumes with male-associated keywords, disadvantaging women candidates for technical roles. - Bias in Facial Recognition Systems Studies have shown that commercial facial recognition systems struggle to accurately identify individuals with darker skin tones, especially women of color. This bias arises from training data that over-represents light-skinned individuals, leading to less accurate results for underrepresented groups.
How to Avoid Algorithmic Bias
Mitigating algorithmic bias requires a combination of technical, ethical, and governance strategies. Here are some approaches that organizations can take to reduce the risk of bias in AI systems:
- Diverse and Representative Data
To ensure AI models reflect the communities they serve, it’s essential to use diverse and representative data during the training phase. This includes ensuring that data from all relevant demographics—across race, gender, age, and other factors—are included and accurately represented. A more inclusive dataset helps prevent the model from developing biased patterns that disadvantage any particular group. - Bias Detection and Mitigation
Ongoing monitoring and testing are necessary to detect and mitigate biases during the AI lifecycle. Techniques such as impact assessments, algorithmic audits, and fairness testing can help identify areas where bias might emerge. Additionally, “human-in-the-loop” systems, where human reviewers assess AI recommendations before decisions are made, can provide another layer of protection. - Transparency and Interpretability
Transparent AI systems are easier to understand and trust. Providing clear documentation about how algorithms are trained, what data is used, and how decisions are made can improve transparency. This level of explainability helps stakeholders understand why a particular decision was made and whether it was based on sound, unbiased reasoning. - Inclusive Design and Development
A diverse team of developers, data scientists, and engineers is essential to ensure that AI systems are developed with a broad range of perspectives. This diversity helps identify potential biases that might otherwise go unnoticed and fosters a more inclusive approach to AI development. - Algorithmic Bias Regulation
Governments and regulatory bodies are increasingly recognizing the need for policies to mitigate algorithmic bias. The European Union’s AI Act, for example, introduces specific guidelines for managing high-risk AI systems and preventing discriminatory outcomes. The U.S. has also implemented frameworks such as the AI Bill of Rights to provide protections against algorithmic discrimination.
Conclusion
Algorithmic bias poses significant risks to the fairness, accountability, and trustworthiness of AI systems. As AI becomes increasingly integrated into decision-making processes across diverse sectors, addressing bias is critical to ensuring that these technologies serve everyone equitably. By understanding the causes and risks of algorithmic bias and implementing effective governance strategies, organizations can reduce the harmful impacts of bias and create AI systems that reflect society’s diverse values and needs. The continued development of responsible AI systems, combined with strong ethical frameworks and regulatory oversight, will help ensure that AI technologies contribute to a fairer, more just world.