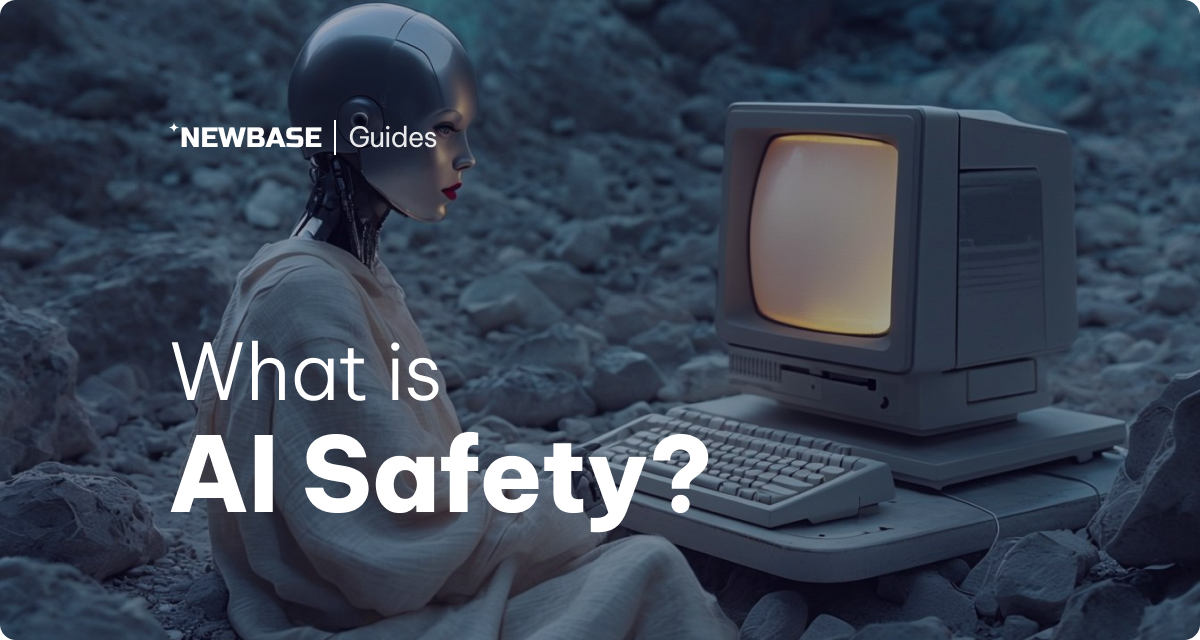
What Is AI Safety?
The increasing prevalence and impact of Artificial Intelligence (AI) necessitates careful consideration of how these technologies are designed and used. AI safety refers to a set of practices and principles that ensure AI benefits humanity and minimizes potential harm. Building safe AI systems is crucial for businesses and society as a whole.
Why is AI Safety Important?
AI systems are becoming increasingly sophisticated and integrated into critical areas of our lives, impacting infrastructure, finance, national security, and more. While AI offers immense potential, concerns about its negative impacts are growing.
- Public Concerns: A 2023 survey found over half of Americans are more concerned than excited about AI use, while another found 83% worry about accidental AI catastrophes.
- Negative Consequences: A 2024 report showed 44% of respondents experienced issues like inaccuracy or cybersecurity problems with their AI use.
- Existential Risks: Advanced AI with capabilities surpassing human intelligence (Artificial General Intelligence – AGI or Artificial Superintelligence – ASI) could pose existential threats if not aligned with human values.
From a business perspective, AI safety is essential for:
- Building trust with consumers
- Guarding against legal liabilities
- Avoiding poor decision-making
Organizations that prioritize responsible AI use can minimize negative consequences for themselves and their customers.
Trust, Transparency, and Governance in AI
Building trust in AI is paramount. This includes addressing issues like bias, explainability, and risk management. Resources like the IBM AI Academy offer guidance on adopting AI in an ethical and responsible manner.
Types of AI Risks
AI risks can be categorized into several areas, each requiring specific safety measures:
- Bias and Fairness: AI systems can perpetuate societal biases if trained on incomplete or biased data. This can lead to unfair outcomes like discriminatory loan approvals or hiring practices.
- Privacy: AI systems raise privacy concerns by potentially accessing, exposing, or misusing personal data. Breaches of sensitive data can place creators or users at risk.
- Loss of Control: Autonomous AI systems, especially those with advanced decision-making capabilities, pose the risk of unpredictable or harmful actions. Stopping them might be difficult without human intervention.
- Existential Risks: Highly advanced AI systems, like AGI or ASI, might endanger humanity or disrupt global systems if mismanaged. Existential threats could arise from an AI “arms race” or malicious manipulation for mass control.
- Malicious Misuse: Bad actors can exploit AI for cyberattacks, misinformation campaigns, illegal surveillance, or even physical harm. These threats exist at individual and societal levels.
- Cybersecurity: AI systems are vulnerable to security issues like adversarial attacks, where malicious actors manipulate data inputs to produce incorrect outputs.
AI Safety vs. AI Security
While related, AI safety and AI security are distinct:
- AI Safety: Addresses inherent issues and unintended consequences of AI.
- AI Security: Focuses on protecting AI systems from external threats like cyberattacks and data breaches.
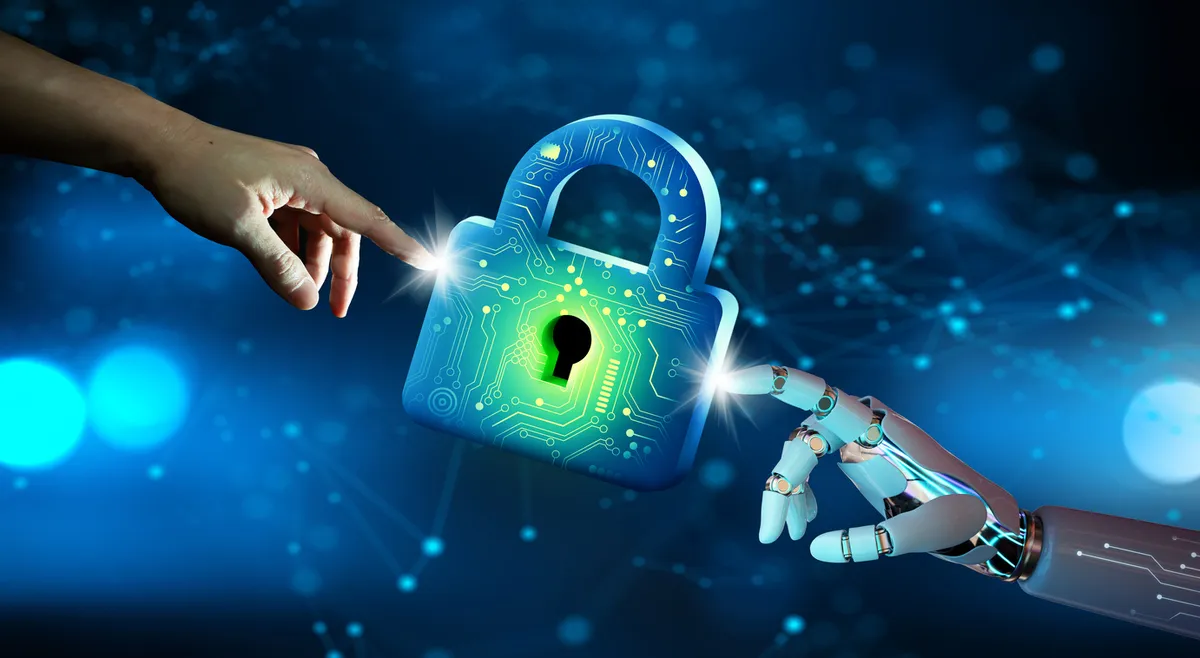
AI safety aims to align AI with human values and minimize negative impacts, using “AI alignment” to encode human goals into models. AI security safeguards the confidentiality and integrity of models, including using AI and Machine Learning (ML) to anticipate and address potential security threats.
AI Safety Measures
AI leaders and businesses are implementing various practices for responsible AI development and use:
- Algorithmic Bias Detection and Mitigation: Techniques like diverse data collection, algorithmic fairness assessments, and debiasing methods help identify and address potential biases within AI systems.
- Robustness Testing and Validation: Rigorous testing processes help AI systems withstand hazards and identify technical risks. Techniques include adversarial testing, stress testing, and formal verification.
- Explainable AI (XAI): Many AI models, especially large language models (LLMs), are “black boxes” with opaque decision-making processes. XAI aims to make these processes more transparent and interpretable.
- Ethical AI Frameworks: Organizations can adopt ethical AI frameworks that guide AI development and use. These frameworks typically include principles like transparency, fairness, accountability, and privacy.
- Human Oversight: While automation offers advantages, maintaining human control over AI systems is crucial for safety. This involves human operators monitoring performance, intervening when necessary, and making final decisions in critical situations.
- Security Protocols: Implementing strong security measures like encryption, access control, and anomaly detection helps protect AI systems from misuse or unauthorized access.
- Industry-wide Collaboration: AI safety is a complex and evolving field requiring collaboration among researchers, industry leaders, and policymakers. Participation in industry consortia, research initiatives, and standardization efforts facilitates knowledge sharing and best practices.
Who is Responsible for AI Safety?
AI safety is a collective responsibility shared by various stakeholders:
- Researchers and Developers: They lay the foundation by designing, building, and testing AI systems. Their focus includes aligning AI goals with human values, creating transparent and explainable models, and conducting rigorous testing to ensure intended behavior.
- Technology Companies: Leading AI companies like IBM, OpenAI, Google, and Microsoft play a crucial role in AI safety. They invest in dedicated safety teams, establish ethical guidelines, and adhere to responsible AI principles. Some companies create frameworks and protocols for risk mitigation, including bias detection tools and human oversight mechanisms.
- Governments and Regulators: Governments worldwide are creating AI safety rules and regulations. International organizations like the United Nations, World Economic Forum, and OECD lead initiatives focusing on AI ethics and safety. In the US, the National Institute of Standards and Technology (NIST) addresses AI safety issues, while the EU AI Act sets safety standards and guidelines.
- Nonprofits and Advocacy Groups: NGOs, think tanks, and other organizations advocate for AI safety, raise awareness about risks, set ethical guidelines, and promote transparency and responsible research. Examples include the Center for AI Safety, Center for Human-Compatible AI, and the Future of Life Institute.
Conclusion
AI safety is a complex and evolving field that requires ongoing attention and collaboration. By understanding the risks and implementing appropriate safety measures, we can harness the power of AI while mitigating potential harm. It’s crucial to prioritize responsible AI development and use to ensure a future where AI benefits humanity.