What Is AI Interpretability? Understanding the Key to Transparent and Trustworthy AI
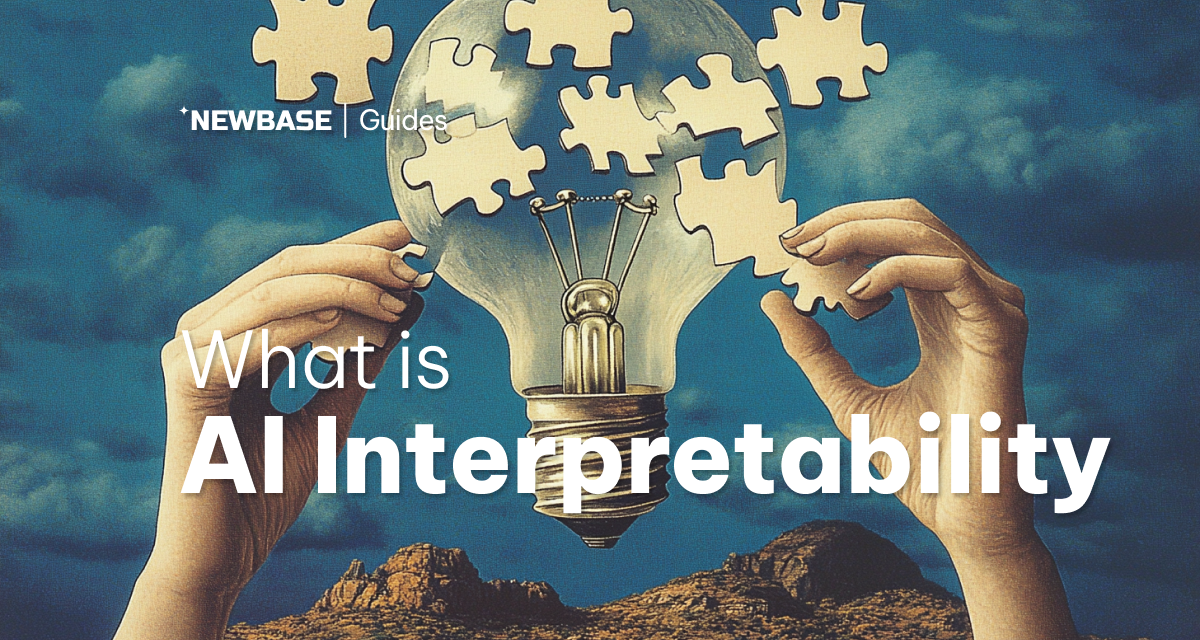
AI interpretability refers to the ability to understand, explain, and articulate how artificial intelligence (AI) models make decisions. As AI systems become more complex, interpretability plays a critical role in ensuring trust, fairness, and accountability in AI-driven processes. By making AI’s decision-making processes more transparent, interpretability enables developers, users, and regulators to better understand how predictions are generated.
AI models use complex webs of data inputs, algorithms, and logic to deliver insights. The more sophisticated the model, the harder it can be for humans to decipher its decision-making process—even for those who built it. An interpretable model allows its decisions to be easily understood by users, making it more reliable and trustworthy.
The Growing Importance of AI Interpretability
The use of AI is growing across various domains, including smart devices, fraud detection, customer support chatbots, and generative AI tools like ChatGPT. As AI models become more advanced, the importance of interpretability increases. Industries such as healthcare, finance, and criminal justice, where AI models influence life-altering decisions, require interpretable AI systems to ensure transparency, fairness, and accountability.
In these high-stakes environments, public trust is paramount. When AI models are understandable, stakeholders are more likely to trust their outputs, which promotes broader adoption of AI technologies. The consequences of non-interpretability are stark, as seen in cases where opaque AI systems produced biased hiring practices or erroneous legal judgments.
White-Box vs. Black-Box Models
- White-Box Models: These models have transparent inputs, logic, and decision paths that users can easily follow. For instance, decision trees and linear regression models are inherently interpretable. However, white-box models tend to be less accurate than more complex alternatives, as their simplicity can limit predictive power.
- Black-Box Models: These are highly complex models like deep learning neural networks. Their internal processes are opaque, and users typically cannot see how specific decisions are made. While black-box models are more accurate and versatile, they raise concerns about bias, fairness, and accountability. Making black-box models interpretable is a key focus of modern AI research, with growing emphasis on explainability tools to bridge this gap.
AI Interpretability vs. AI Explainability
While often used interchangeably, interpretability and explainability have distinct meanings.
- Interpretability focuses on how an AI model works internally. It allows users to understand the model’s architecture and how it processes features to generate predictions. It requires detailed disclosure of the model’s inner workings.
- Explainability deals with how and why a model arrived at a specific prediction. It aims to provide justifications for a model’s output, often after the prediction has been made. Explainable AI (XAI) uses methods to present model predictions in human-readable formats, enabling non-technical users to understand them.
Why Is AI Interpretability Important?
AI interpretability is crucial for several reasons:
- Trust: Transparency fosters user trust. When stakeholders understand a model’s reasoning, they are more likely to trust its outputs.
- Bias and Fairness: Models can perpetuate biases in training data, leading to unfair outcomes. Interpretability allows developers to identify and correct biased decision-making.
- Debugging: Debugging an AI model without interpretability is challenging. Interpretable models allow developers to identify the causes of incorrect predictions and make adjustments.
- Regulatory Compliance: Regulations like the EU’s AI Act and GDPR require transparency in AI decision-making. Interpretability helps companies comply with these laws.
- Knowledge Transfer: Interpretable models facilitate knowledge sharing, enabling developers and researchers to use insights from one model to inform the development of new models.
Types of Interpretability
Stanford researcher Nigam Shah identifies three main types of interpretability:
- Engineers’ Interpretability: This type focuses on understanding the internal structure and workings of a model, which is useful for debugging and optimization.
- Causal Interpretability: This focuses on explaining why the model produced a specific output by identifying which features most influenced the prediction.
- Trust-Inducing Interpretability: This focuses on making the model’s decision-making process clear to end-users, often through natural language explanations or visualizations.
Factors Affecting Interpretability
Several key factors influence AI interpretability:
- Intrinsic vs. Post-Hoc Interpretability: Intrinsically interpretable models, like decision trees, are simple and easy to understand. Post-hoc interpretability, however, applies to black-box models after they are trained, often using tools like SHAP or LIME.
- Local vs. Global Interpretability: Local interpretability explains individual predictions, while global interpretability reveals the overall decision patterns of a model.
- Model-Specific vs. Model-Agnostic Methods: Model-specific methods work for only certain types of models, while model-agnostic methods, like LIME, can be applied to any model.
Methods for Enhancing Interpretability
To make complex models more interpretable, several techniques are employed:
- Local Interpretable Model-Agnostic Explanations (LIME): LIME explains a specific prediction by creating simpler, interpretable models for that single instance.
- Shapley Additive Explanations (SHAP): SHAP uses cooperative game theory to measure each feature’s contribution to a prediction.
- Partial Dependence Plots (PDPs): PDPs visualize the relationship between a feature and the model’s output, providing insight into how changing that feature affects predictions.
- Individual Conditional Expectation (ICE) Plots: ICE plots highlight how a single instance’s prediction changes as a feature’s value shifts.
- Counterfactual Explanations: These explanations show what changes to input features would have led to a different outcome, offering users actionable insights.
Use Cases of AI Interpretability
AI interpretability is essential across industries:
- Healthcare: AI-driven diagnosis and treatment recommendations require transparency so medical professionals can understand and trust the recommendations.
- Finance: AI is used for fraud detection, credit scoring, and financial planning. Interpretability helps ensure fair treatment and regulatory compliance.
- Criminal Justice: AI models influence decisions like parole recommendations. Interpretability is crucial to prevent unjust outcomes and build trust.
- Human Resources: AI used for resume screening and hiring must be free from bias. Interpretability helps to identify and remove unfair practices.
- Insurance: Interpretability explains how AI models determine insurance premiums, making the process fairer and more transparent for customers.
Challenges of AI Interpretability
While crucial, interpretability is not without challenges:
- Performance-Interpretability Trade-off: Simpler, interpretable models may have lower predictive accuracy than black-box models.
- Lack of Standardization: There is no universal approach to interpreting AI models, leading to inconsistencies in explanations.
- Subjectivity: What’s interpretable to one user may be confusing to another, making it difficult to create universally interpretable models.
- Privacy and Security: Making models more transparent may expose sensitive information, making them vulnerable to adversarial attacks.
Conclusion
AI interpretability is essential for ensuring trust, accountability, and fairness in AI systems. It enables developers, users, and regulators to understand how models operate, which is especially important in high-stakes industries like healthcare, finance, and criminal justice. While challenges remain, advances in interpretability methods like LIME, SHAP, and PDPs are helping bridge the gap between performance and transparency. As AI becomes more integral to daily life, interpretability will remain a vital aspect of ethical and responsible AI development.