What is AI Data Management? How to Optimize Your AI Data Strategy
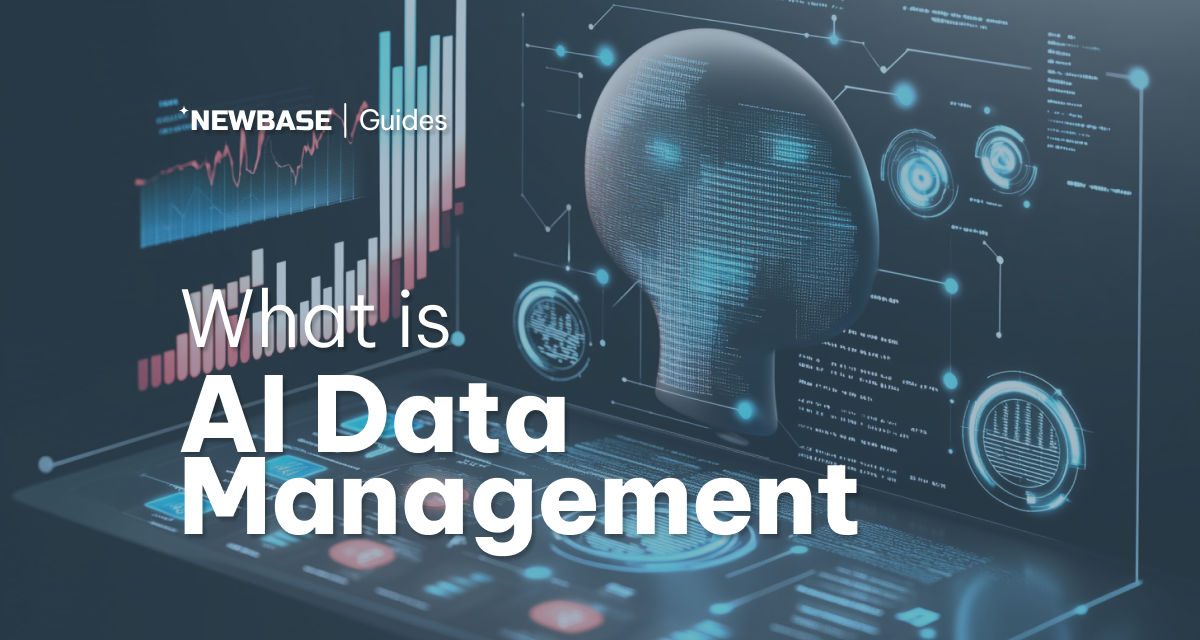
In today’s fast-paced digital world, organizations are dealing with massive amounts of data. Managing this data efficiently has become crucial for businesses to stay competitive. AI data management refers to the practice of using artificial intelligence (AI) and machine learning (ML) to optimize the data management lifecycle. This includes data collection, cleaning, analysis, security, and overall management processes.
Traditional, rule-based AI and advanced generative AI models both play vital roles in modern data management. By leveraging AI, companies can transform how they handle data, ensuring better access, reliability, and usability. This shift allows businesses to make more informed decisions and drive strategic initiatives.
Why is AI Data Management Important?
Modern enterprises generate and manage vast amounts of data, including financial transactions, product inventory, employee records, and customer preferences. However, challenges arise when data is fragmented, inaccurate, or inaccessible. According to IBM’s Data Differentiator report, 82% of enterprises face data silos that hinder workflows, and 68% of organizational data goes unanalyzed, meaning businesses fail to realize its full potential.
AI data management addresses these issues by automating critical processes like integrating data sources, cleaning datasets, and ensuring data accessibility. Additionally, AI data management helps organizations create high-quality data pipelines, which are essential for training and deploying AI models and machine learning algorithms.
Key AI Data Management Tools
Many traditional data management tools now integrate AI capabilities, such as:
- Data storage solutions: AI enhances storage efficiency and optimization.
- Data integration tools: AI enables seamless connection of disparate data sources.
- Master data management (MDM) tools: AI identifies and merges duplicate records.
- Governance solutions: AI ensures compliance with data privacy and protection standards.
These tools leverage both traditional AI algorithms and generative AI models, such as Microsoft Copilot, Meta’s LLaMA, and IBM Granite™. By embedding AI, these solutions can process natural language queries, create data summaries, and offer user-friendly, human-like interactions.
AI Data Management Use Cases
AI can support nearly every aspect of the data management lifecycle. Here are some of the most common applications:
1. Data Discovery
Organizations handle data from multiple sources, stored in different formats across public and private clouds, on-premises storage, and personal devices. Without central visibility, it’s difficult to know what data exists or how to control it. Undiscovered “shadow data” also poses security risks—one-third of data breaches involve shadow data, with an average breach cost of USD 5.27 million.
Examples of AI in Data Discovery:
- AI-powered data discovery tools: Automatically scan devices and repositories, indexing new data in real-time.
- Automated data classification tools: Use machine learning to tag new data, such as identifying nine-digit numbers as U.S. Social Security numbers.
- LLM-powered data extraction: Extract structured information from unstructured sources like resumes, extracting contact details and work history.
2. Data Quality
Bad data leads to flawed decision-making. If data is inaccurate or incomplete, it undermines business initiatives and AI model performance. AI tools improve data quality by identifying and correcting errors automatically.
Examples of AI in Data Cleaning:
- AI-enabled data preparation tools: Identify and fix errors, irregular formats, and invalid entries.
- Synthetic data generators: Fill gaps in datasets with synthetic data points based on existing patterns.
- AI-driven MDM tools: Merge duplicate customer records with matching names, addresses, and contact details.
- Data observability tools: Generate data lineage records to track how data changes over time.
3. Data Accessibility
Data silos prevent businesses from making full use of their data. AI facilitates data integration, allowing users to access unified datasets in a seamless manner.
Examples of AI in Data Accessibility:
- AI-enabled data integration tools: Automatically detect relationships between datasets and merge them.
- Metadata management tools: Automatically create data catalogs and tag datasets.
- LLM-powered interfaces: Allow users to query datasets using natural language instead of SQL.
- AI-driven query engines: Improve query performance by optimizing database execution.
4. Data Security
Data breaches cost organizations an average of USD 4.88 million, making security a top priority. AI can enhance data security by monitoring for threats and automating security measures.
Examples of AI in Data Security:
- AI-driven data loss prevention tools: Identify sensitive information and apply security controls automatically.
- Anomaly detection tools: Detect unusual activity, such as abnormal data transfers.
- AI-enabled role-based access control (RBAC): Use LLMs to generate security roles and access rules.
- Fraud detection tools: Spot suspicious transactions using AI pattern analysis.
Benefits of AI Data Management
AI can revolutionize data management by making processes more efficient, accurate, and secure. Key benefits include:
- Increased Efficiency: AI automates manual, time-consuming processes like data discovery, cleaning, and cataloging.
- Improved Accuracy: AI can identify and correct errors in large datasets faster than human users.
- Enhanced Data Accessibility: Unified data repositories enable teams to access the right data at the right time.
- Stronger Security: AI-driven security tools reduce the risk of data breaches and ensure compliance with industry regulations.
- Better AI Model Training: Clean, high-quality data is essential for training machine learning models and generative AI systems.
The Role of AI in Business Data Strategies
According to AvePoint’s AI and Information Management Report, 64% of organizations manage at least one petabyte of data—equal to 9 quadrillion bits of information. A significant portion of this data is unstructured (e.g., text, images, video), making it difficult to manage manually. AI and ML tools can automate the discovery, integration, and cleaning of this data, enabling businesses to:
- Support predictive analytics: Forecast future trends in consumer spending using historical data.
- Democratize data access: Enable employees without data science backgrounds to access, analyze, and use data.
- Enhance AI model training: Use business-specific data to train AI models, enhancing their performance for industry-specific applications.
Fueling AI Initiatives with High-Quality Data
AI models require high-quality data to perform well. The IBM Institute for Business Value reports that 59% of CEOs believe future competitive advantage hinges on generative AI adoption. Organizations that develop AI models need clean, accessible, and relevant data pipelines.
AI data management helps organizations streamline these pipelines, ensuring their data is fit for AI training. By leveraging AI-enabled data management, businesses can:
- Prepare Data for AI: AI automates data prep, cleaning, and annotation.
- Reduce Costs: Automated processes reduce the need for manual intervention.
- Build Industry-Specific Models: Tailor AI models to specific business needs using industry-specific data.
Complying with Data Privacy and Security Regulations
Enterprises must comply with regulations like GDPR and the Payment Card Industry Data Security Standard (PCI-DSS). For 57% of CEOs, data security is a barrier to AI adoption, and 45% see data privacy as another obstacle. AI data management can help organizations comply with privacy laws by:
- Enforcing Governance: Automatically applying role-based access controls.
- Generating Synthetic Data: Use synthetic data to remove sensitive personal information.
- Applying Real-Time Protections: Prevent unauthorized access and flag potential breaches.
Conclusion
AI data management transforms how organizations handle, secure, and utilize data. By automating critical tasks like discovery, cleaning, and security, AI enables organizations to unlock the full potential of their data assets. With clean, accessible, and secure data pipelines, businesses can train more effective AI models, comply with regulations, and make data-driven decisions with confidence.