What is AI Bias? Understanding AI Bias and How We Can Make AI Fairer
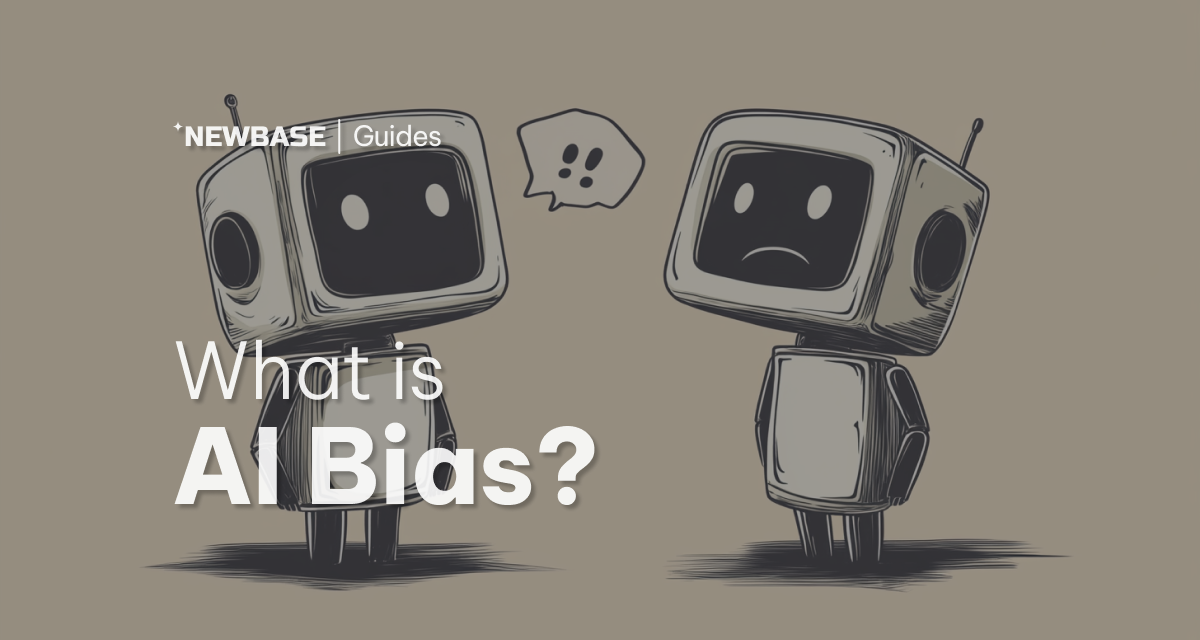
AI bias, also referred to as machine learning bias or algorithm bias, is the manifestation of systematic errors in artificial intelligence systems due to biases inherent in their design, training data, or implementation. These biases can result in skewed outputs, perpetuating harmful stereotypes, and causing unfair or inaccurate outcomes across various sectors. By embedding human prejudices, societal inequities, or unbalanced datasets into AI models, AI bias poses significant challenges to achieving equitable and effective AI systems.
The Impact of AI Bias
Unchecked AI bias has far-reaching implications for organizations and society. It undermines the reliability and accuracy of AI systems, diminishing their potential benefits and eroding trust among users. For businesses, this can translate into poor decision-making, reputational damage, and lost opportunities. For individuals, especially those from marginalized communities, biased AI systems can exacerbate existing inequalities, limiting access to resources, opportunities, and fair treatment.
Real-World Examples of AI Bias
- Healthcare:
Predictive AI algorithms in healthcare often underperform for minority groups due to underrepresentation in training data. For instance, computer-aided diagnostic systems have been shown to produce less accurate results for African-American patients compared to white patients, potentially leading to misdiagnoses or inadequate care. - Recruitment:
AI-driven resume scanning tools, while efficient, can inadvertently reinforce gender or racial biases. For example, job postings containing words like “rockstar” or “ninja” may disproportionately attract male applicants, skewing the candidate pool and disadvantaging women and non-binary individuals. - Image Generation:
Studies have revealed biases in AI-generated imagery. For instance, when asked to create images of professionals like CEOs or doctors, models like Stable Diffusion predominantly generated depictions of white males, reinforcing outdated stereotypes about race and gender in leadership and specialized professions. - Predictive Policing:
AI tools used in law enforcement often rely on historical arrest data. This perpetuates existing patterns of racial profiling, leading to disproportionate targeting of minority communities and raising ethical and legal concerns.
Types of AI Bias
Understanding the different types of AI bias is crucial to addressing and mitigating its effects. Here are some common forms:
- Algorithm Bias:
Occurs when the questions or problems posed to the AI are incomplete or misinformed, leading to skewed or misleading outputs. - Cognitive Bias:
Human developers inadvertently introduce personal biases into the AI system, affecting its training data or operational behavior. - Confirmation Bias:
AI models may overly rely on pre-existing patterns in data, reinforcing existing stereotypes or failing to recognize new trends. - Exclusion Bias:
Important data may be omitted from training datasets, often due to oversight, leading to incomplete or inaccurate outputs. - Measurement Bias:
Arises from using datasets that do not comprehensively represent the target population. For example, training a model on data exclusively from college graduates would skew its performance when applied to the general population. - Out-Group Homogeneity Bias:
Developers may create algorithms that struggle to differentiate among individuals outside the majority group represented in the data, leading to errors and misclassifications. - Prejudice Bias:
Stereotypes and societal assumptions embedded in the training data result in outputs that reinforce harmful prejudices. - Sample/Selection Bias:
Insufficient or non-representative training data leads to AI systems that fail to generalize effectively. - Stereotyping Bias:
AI systems inadvertently perpetuate harmful stereotypes, such as associating certain professions with specific genders or ethnicities. - Recall Bias:
Inconsistent labeling during data annotation can result in uneven training and skewed model outputs.
Addressing AI Bias
Effective strategies are essential for minimizing bias and promoting fairness in AI systems. Below are some approaches:
1. Implementing AI Governance
AI governance involves establishing frameworks, policies, and practices to guide the responsible development and deployment of AI. Key components include:
- Assessing fairness, equity, and inclusion in AI models.
- Utilizing techniques like counterfactual fairness to ensure equitable outcomes across diverse groups.
2. Ensuring Transparency
Transparent practices help stakeholders understand how AI systems are built and function. This includes documenting the datasets, algorithms, and decision-making processes to identify potential biases early on.
3. Building Diverse Teams
Inclusive AI development teams with varied perspectives can better recognize and address potential biases. Diversity in race, gender, educational background, and expertise ensures a holistic approach to problem-solving.
4. Human-in-the-Loop Systems
Incorporating human oversight at critical decision-making points allows AI outputs to be reviewed and corrected, reducing the risk of biased outcomes.
5. Robust Data Practices
- Selecting balanced training data that reflects the diversity of the target population.
- Regularly auditing datasets to identify and rectify gaps or imbalances.
6. Continuous Monitoring
AI systems require ongoing evaluation to detect and address biases as new data becomes available or as societal norms evolve. Independent assessments by third-party organizations can provide an additional layer of accountability.
7. Technological Interventions
Emerging tools and techniques, such as bias detection software and fairness optimization algorithms, help identify and mitigate bias during model training and deployment.
Principles for Ethical AI Development
Organizations can follow these guiding principles to build ethical and unbiased AI systems:
- Proactive Design:
Address potential biases during the conceptual phase to prevent costly corrections later. - Ethical Decision-Making:
Ensure AI applications align with societal values, prioritizing fairness and inclusivity. - Stakeholder Engagement:
Involve diverse stakeholders, including representatives from impacted communities, to inform AI development and deployment. - Regulatory Compliance:
Adhere to industry standards and legal frameworks designed to promote ethical AI use.
Conclusion
AI bias is a critical challenge in the development and deployment of artificial intelligence systems. Left unaddressed, it can perpetuate societal inequalities, harm marginalized groups, and erode trust in AI technologies. By understanding the sources and types of bias and implementing comprehensive strategies to mitigate them, organizations can build AI systems that are equitable, reliable, and beneficial for all. As AI continues to shape our world, prioritizing fairness and inclusivity will be essential to unlocking its full potential.