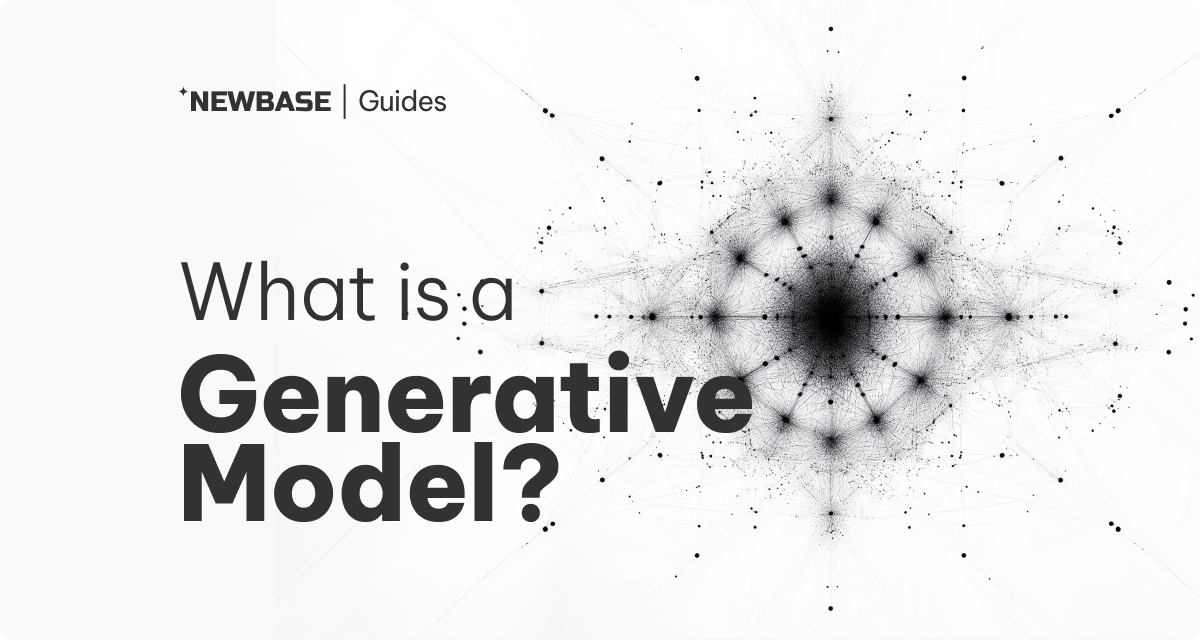
Generative models, the vanguards of artificial intelligence, have taken the world by storm. These ingenious models aren’t mere number crunchers; they are the architects of entirely new data, mimicking the patterns and distributions gleaned from their training sets to produce novel content. This sets them apart from their analytical counterparts, forever changing the landscape of AI.
The Genesis of Generative Models: From Automation to Innovation
The evolution of generative models wasn’t an overnight feat. It stemmed from the gradual digitization and automation that revolutionized the financial sector. Early fintech solutions, a collaboration between APIs, mobile applications, and web services, streamlined transactions and enhanced user experience. However, the true catalyst for generative models arrived in the form of a data deluge. Financial institutions began amassing vast troves of data, presenting both a challenge and an opportunity. Generative AI emerged as the key to unlocking the hidden potential within this data, paving the way for a new era of intelligent fintech solutions.
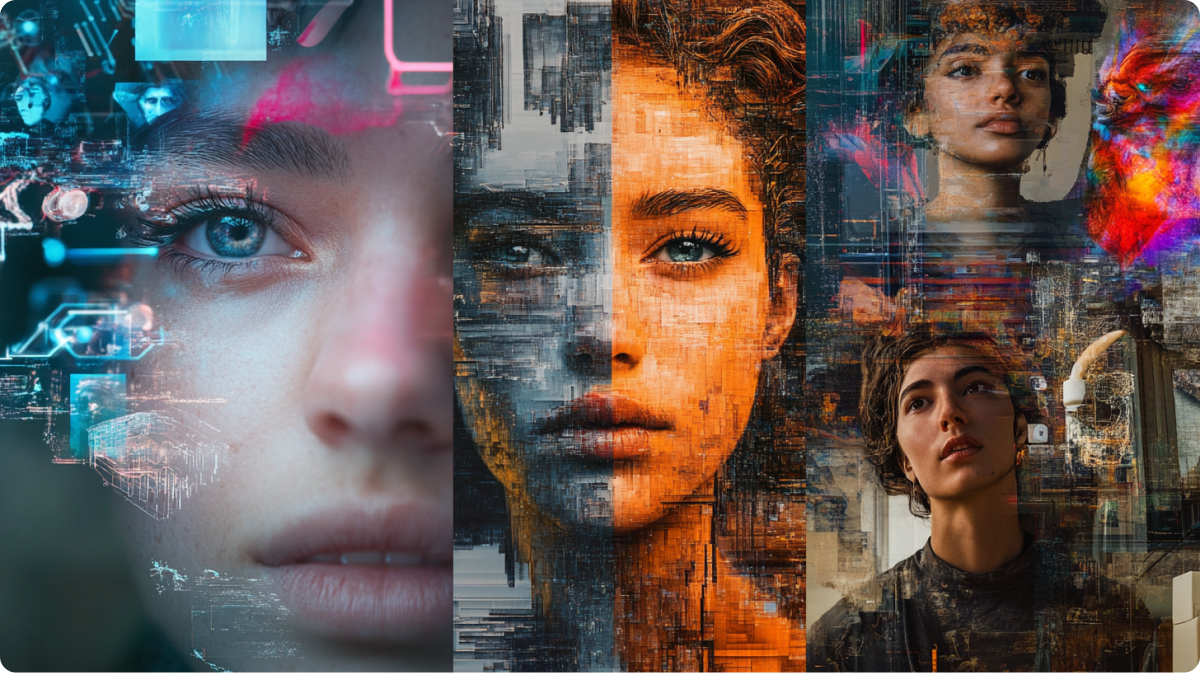
The Power of Creation: How Generative Models are Transforming Industries
Generative models offer a plethora of benefits that extend far beyond financial services:
- Data-Driven Decision Making: By analyzing massive datasets, generative models can identify patterns and predict future trends, empowering businesses to make informed decisions in areas like risk management, market analysis, and investment strategies.
- Enhanced Security: Fraudulent activities pose a constant threat. Generative AI’s anomaly detection capabilities can analyze transactions in real-time, identifying suspicious patterns and preventing fraud attempts before they occur.
- Personalized Customer Experiences: AI-powered chatbots equipped with natural language processing (NLP) can provide 24/7 customer support, offering personalized recommendations and addressing inquiries with unmatched efficiency.
- Automated Processes: Generative models can automate repetitive tasks such as data entry, invoicing, and payment processing, freeing up human resources for higher-value activities.
- Algorithmic Trading and Portfolio Management: Generative models can analyze vast amounts of financial data, including news, market sentiment, and historical trends, to develop data-driven trading strategies and manage investment portfolios with greater precision.
A Glimpse Inside the Machine: Unveiling the Different Types of Generative Models
The realm of generative models is a diverse one, with each type boasting unique strengths:
- Autoregressive Models: These models excel at predicting the next element in a sequence, analyzing the preceding elements to determine the most likely continuation. This makes them ideal for tasks like text generation, code completion, and time-series forecasting.
- Diffusion Models: Imagine a sculptor meticulously chipping away at a block of marble to reveal a hidden masterpiece. Diffusion models operate similarly, gradually adding noise to input data before meticulously refining it into novel, yet similar, data. This process makes them particularly adept at image generation and inpainting (adding or removing content within an image).
- Generative Adversarial Networks (GANs): GANs are a captivating innovation, pitting two models against each other in a competitive dance. A generative model strives to create data that a discriminative model mistakes for real data. This adversarial training fosters the creation of highly realistic outputs, particularly in the domain of image generation.
- Variational Autoencoders (VAEs): VAEs function by compressing input data into a latent space, a compressed representation that captures the essence of the data. This compressed data is then decoded back into its original form, albeit with some variations. While not the most dominant player in image generation, VAEs excel in tasks like anomaly detection, data imputation (filling in missing data points), and semi-supervised learning (classifying data with limited labels).
- Flow-Based Models: These models excel at understanding the intricacies of data distribution. They achieve this by transforming simple distributions into increasingly complex ones through a series of reversible mathematical operations. This in-depth understanding of data distribution allows them to generate new data samples that closely resemble the original dataset.
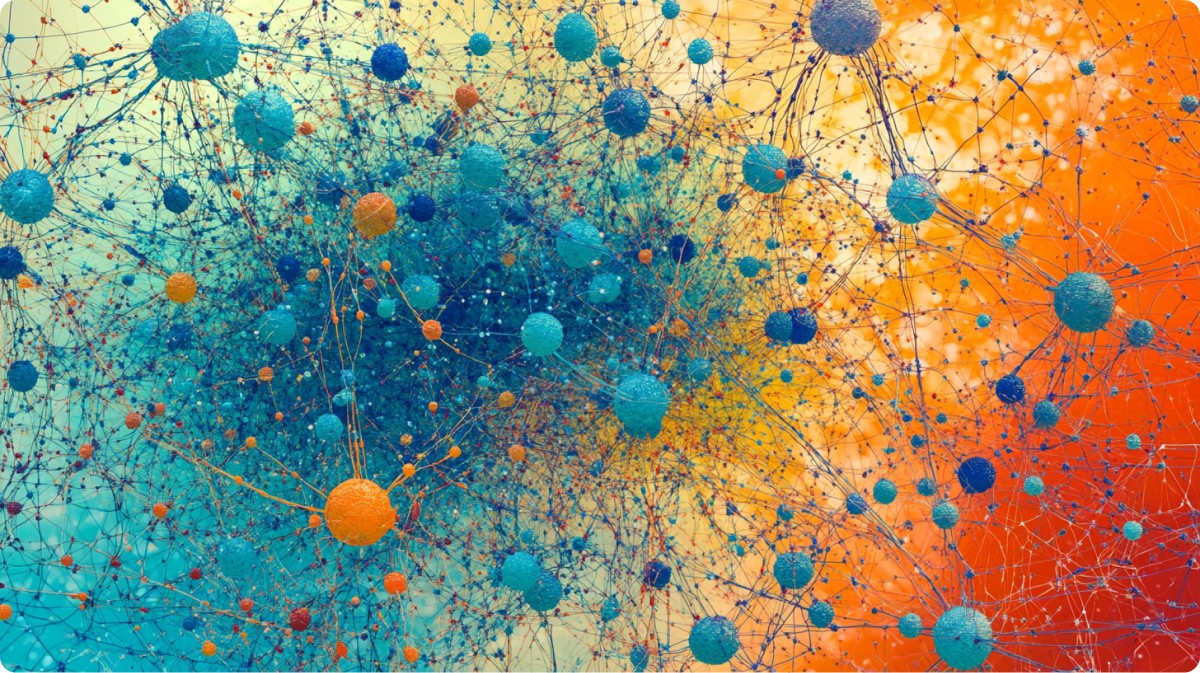
The Hallucination Conundrum: When Imagination Runs Wild
Despite their brilliance, generative models aren’t infallible. They are susceptible to a phenomenon known as hallucination, where they produce misleading or incorrect information. This often occurs when they identify patterns in their training data that don’t exist in the real world. To mitigate this, best practices include providing clear prompts, ensuring high-quality training data, and employing techniques like human verification and fine-tuning.
The Road Ahead: A Future Filled with Generative Potential
As AI technology continues to evolve, we can expect even more sophisticated generative models capable of generating content that pushes the boundaries of creativity and realism. Generative models have the potential to revolutionize various sectors, from scientific discovery and drug development to entertainment and artistic expression.
However, it’s crucial to approach this technology with a sense of responsibility. Ethical considerations, such as the potential misuse of AI for malicious purposes or the creation of deepfakes, must be addressed. By fostering transparency, accountability, and ethical guidelines, we can harness the power of generative models for the betterment of society.
In conclusion, generative models represent a significant leap forward in the field of AI. Their ability to create novel and realistic content has the potential to reshape industries and inspire new forms of creativity. As technology continues to advance, we can anticipate even more exciting developments in the world of generative AI.