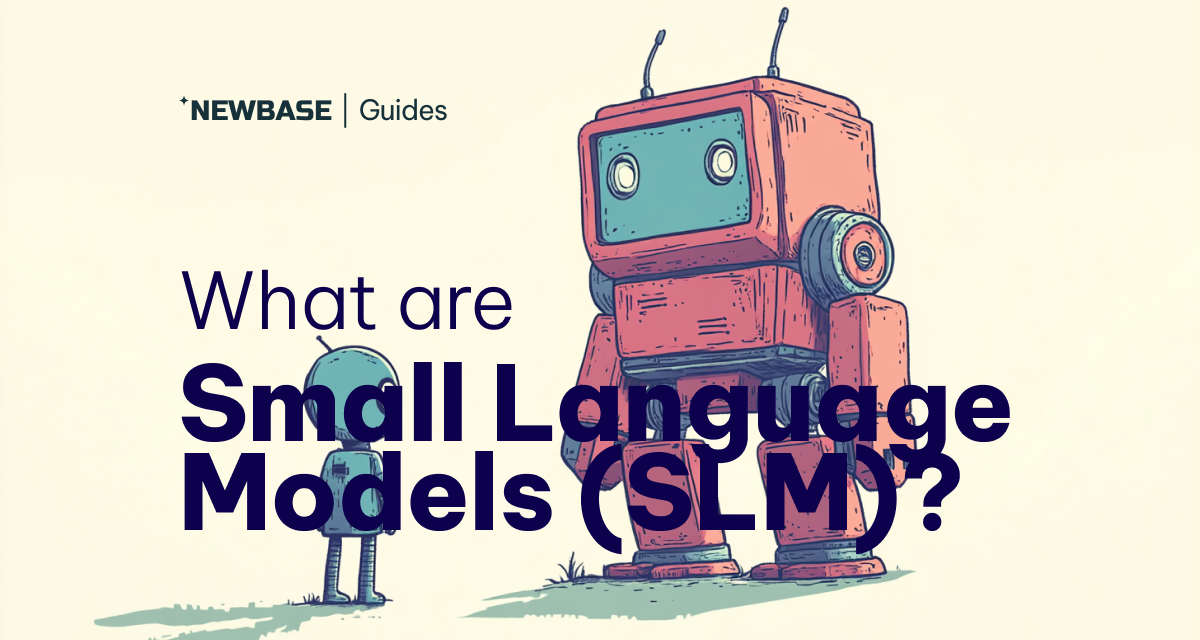
Small language models (SLMs) are a type of artificial intelligence (AI) designed to process, understand, and generate natural language content. Just like their name suggests, SLMs are more compact and limited in scope compared to large language models (LLMs).
Size Matters: Parameters and Performance
SLMs typically have a parameter range of a few million to a few billion. Parameters are internal variables in a model that are adjusted during training to influence its behavior. LLMs, on the other hand, boast hundreds of billions or even trillions of parameters. This difference in size translates to distinct advantages for SLMs:
- Efficiency: Their lean design makes SLMs less resource-intensive, enabling faster training and deployment.
- Accessibility: Researchers, developers, and anyone interested in exploring language models can experiment with SLMs without requiring access to expensive hardware like multiple GPUs.
- Performance: Efficiency doesn’t have to compromise effectiveness. Some SLMs demonstrate performance comparable or even superior to their larger counterparts. For instance, GPT-4o mini surpasses its predecessor, GPT-3.5 Turbo, in various LLM benchmarks encompassing language understanding, question answering, reasoning, and code generation.
How Small Language Models Work
The foundation for SLMs is often laid by LLMs. Both types of models leverage a neural network architecture called the transformer. Transformers are fundamental to natural language processing (NLP) and form the building blocks for models like the generative pre-trained transformer (GPT). Here’s a simplified breakdown of the transformer architecture:
- Encoders: These transform input sequences into numerical representations known as embeddings. Embeddings capture the meaning and position of individual tokens (words or phrases) within the input sequence.
- Self-Attention Mechanism: This allows transformers to focus on the most crucial tokens in the input sequence, regardless of their position.
- Decoders: Decoders utilize the self-attention mechanism and the encoders’ embeddings to generate the most statistically probable output sequence.
Making Small Models Even Smaller: Model Compression Techniques
Techniques are employed to create a more streamlined model from a larger one. This process, known as model compression, aims to reduce the model’s size while retaining as much accuracy as possible. Here are some common model compression methods:
- Pruning: This method removes unnecessary parameters from the neural network. These can include connections between neurons (weights set to 0), neurons themselves, or entire layers. However, pruned models often require fine-tuning after the process to compensate for any accuracy loss. It’s crucial to find the right balance, as over-pruning can degrade performance.
- Quantization: This converts high-precision data into lower-precision data. For example, model weights and activation values (numbers assigned to neurons) can be represented as 8-bit integers instead of 32-bit floating point numbers. Quantization lightens the computational load and accelerates processing. It can be integrated into model training (quantization-aware training) or done after training (post-training quantization).
- Low-Rank Factorization: This decomposes a large matrix of weights into a smaller, lower-rank matrix. This more compact approximation leads to fewer parameters, reduced computations, and simpler complex matrix operations. However, low-rank factorization can be computationally expensive and more challenging to implement. Like pruning, factorized networks require fine-tuning to recover accuracy.
- Knowledge Distillation: This involves transferring the knowledge of a pre-trained “teacher model” to a “student model.” The student model is trained to not only match the teacher model’s predictions but also mimic its underlying reasoning process. Essentially, the knowledge of a larger model is “distilled” into a smaller one. Knowledge distillation is a popular approach for many SLMs, typically using the offline distillation scheme where the teacher model’s weights are frozen during the process.
Examples of Small Language Models
- DistilBERT: A distilled version of BERT, designed for faster inference and reduced memory usage.
- Gemma: Google’s family of lightweight language models, derived from the same technology as Gemini.
- GPT-4o mini: A smaller, more affordable variant of OpenAI’s GPT-4, capable of handling various language tasks.
- Granite: IBM’s series of open-source LLMs, including smaller models optimized for specific tasks.
- Llama: Meta’s open-source language models, available in various sizes, including smaller variants.
- Ministral: A series of SLMs from Mistral AI, including smaller models that are efficient and performant.
- Phi: Microsoft’s suite of small language models, designed for a range of tasks and resource constraints.
Benefits of Small Language Models
- Efficiency: SLMs are less computationally intensive, making them suitable for resource-constrained environments.
- Cost-Effectiveness: Training and deploying SLMs require less computational power and resources compared to large models.
- Faster Inference: SLMs can process information and generate responses more quickly.
- Reduced Environmental Impact: Smaller models consume less energy and have a lower carbon footprint.
- Privacy and Security: SLMs can be deployed on edge devices or private servers, enhancing data privacy and security.
Limitations of Small Language Models
- Reduced Capabilities: SLMs may have limitations in handling complex tasks that require extensive knowledge and reasoning.
- Bias and Fairness: Smaller models might inherit biases from their training data, leading to potential biases in their outputs.
- Hallucinations: SLMs can sometimes generate incorrect or nonsensical outputs, especially when faced with ambiguous or out-of-domain queries.
Conclusion
Small language models offer a compelling alternative to their larger counterparts, providing a balance between performance and efficiency. As technology continues to advance, we can expect further improvements in the capabilities and performance of SLMs. By understanding their strengths and limitations, developers can effectively leverage SLMs to create innovative and practical applications.