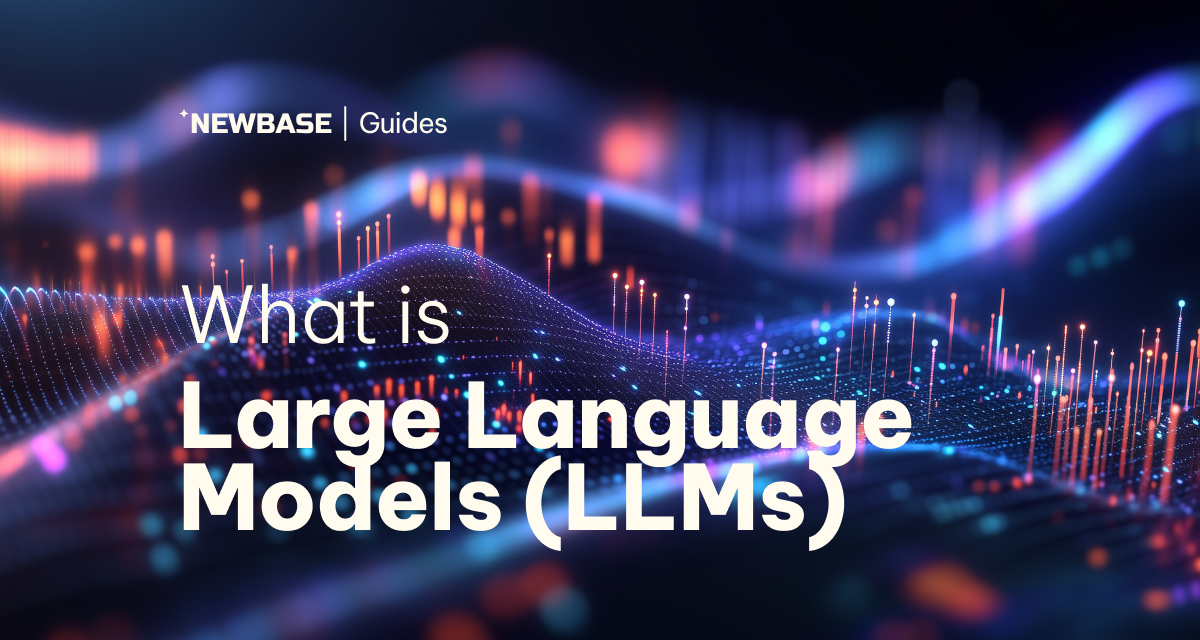
Large language models (LLMs) are a transformative class of foundation models in artificial intelligence (AI) that are trained on vast amounts of data. Their core purpose is to understand, process, and generate human-like text and other content types. This unique capability enables them to perform a wide range of language-based tasks, driving advances in natural language processing (NLP) and natural language understanding (NLU).
The Rise of LLMs
LLMs have surged in popularity, largely due to their role in bringing generative AI to mainstream attention. Their ability to power AI-driven applications like chatbots, content generation, and virtual assistants has made them a focal point for enterprises looking to harness the potential of AI. Organizations across sectors are integrating LLMs into their workflows, enabling automation and enhancing business processes.
While it may seem that LLMs appeared out of nowhere, their development has been ongoing for years. Companies like IBM, Google, OpenAI, and Meta have been at the forefront of these advancements. IBM, for instance, has implemented LLMs to improve NLU and NLP capabilities within its AI-driven platforms, including Watson products.
What Makes LLMs Unique?
At their core, LLMs differ from traditional, domain-specific models. Instead of building and training a separate model for each specific task, LLMs are trained on enormous datasets that allow them to support multiple use cases. This “train once, use many times” approach reduces costs, increases flexibility, and boosts performance across a broad range of tasks.
Unlike earlier models, LLMs employ advanced architectures, such as transformer models, which enable them to process and understand sequential data more effectively. This shift has unlocked new possibilities for AI applications, making LLMs the go-to choice for tasks requiring language comprehension and generation.
How Do Large Language Models Work?
LLMs leverage deep learning, a subfield of machine learning, to process and analyze vast textual datasets. The key to their success lies in the transformer architecture, which allows the model to “attend” to different parts of the input data as it makes predictions. This attention mechanism helps the model focus on relevant words and phrases, improving its comprehension and contextual understanding.
Here’s a simplified explanation of how LLMs are trained and operate:
- Tokenization: Text is broken down into smaller chunks, called tokens, which can be words, subwords, or characters. Tokenization helps the model process and analyze text more efficiently.
- Embedding: Tokens are converted into numerical representations, known as embeddings, that capture semantic relationships between words.
- Training: Using billions of pages of text data, the model learns the relationships between words, grammatical structures, and concepts. Training is self-supervised, meaning the model predicts the next word in a sentence based on the preceding context.
- Attention Mechanism: Transformers’ attention mechanisms identify which words in a sentence are most relevant to the prediction task, leading to more accurate and context-aware responses.
- Prediction: Once trained, the model can generate responses by predicting the most likely next token, which builds coherent and contextually relevant sentences.
Popular LLMs in Use Today
Several LLMs are available for both public and enterprise use. Notable examples include:
- GPT-3 and GPT-4: Developed by OpenAI, these models support chatbots like ChatGPT and are widely used for content generation, coding assistance, and more.
- Google’s BERT, RoBERTa, and PaLM: These models are known for their efficiency in sentiment analysis, content classification, and contextual search.
- Meta’s LLaMA Models: Meta’s LLaMA series focuses on open-source development, making LLMs more accessible to the AI research community.
- IBM’s Granite Models: Available on Watsonx.ai, IBM’s LLMs are designed for enterprise applications, supporting AI assistants, automation, and enhanced NLP.
Use Cases for LLMs
The applications for LLMs are vast and growing. Here are some key use cases where LLMs are driving value:
- Text Generation: LLMs can create written content like blog posts, email drafts, and marketing copy. Businesses use them to generate mid-to-long-form text quickly and efficiently.
- Content Summarization: Long articles, reports, or legal documents can be condensed into shorter summaries, saving time and improving productivity.
- AI Assistants and Chatbots: LLM-powered chatbots provide human-like responses to customer inquiries, enhancing customer service and self-service support.
- Code Generation and Debugging: Developers use LLMs to generate, review, and debug code, often “translating” between programming languages.
- Sentiment Analysis: By analyzing text data, LLMs identify the sentiment behind customer feedback, supporting brand reputation management.
- Language Translation: LLMs break down language barriers by offering highly accurate translations across multiple languages.
These use cases span industries from healthcare and finance to customer service and marketing. The ability of LLMs to automate, streamline, and optimize business processes makes them essential to digital transformation.
Addressing Model Hallucinations and Bias
Despite their remarkable capabilities, LLMs aren’t perfect. They can sometimes generate factually incorrect or biased responses, a phenomenon known as “hallucination.” Enterprises deploying LLMs must take steps to mitigate these risks through fine-tuning, reinforcement learning with human feedback (RLHF), and robust governance practices.
- Fine-Tuning: Adjusting model parameters to better align responses with factual data and enterprise requirements.
- Prompt Engineering: Crafting precise prompts to guide the model’s responses in a desired direction.
- Reinforcement Learning with Human Feedback (RLHF): Humans provide feedback on responses to reduce inaccuracies and biases.
LLM Governance and Compliance
For enterprises to adopt LLMs at scale, governance and compliance are critical. Governance involves ensuring that AI systems operate transparently, securely, and ethically. To achieve this, organizations must:
- Trace Data and Models: Maintain clear records of training data, model changes, and system decisions.
- Ensure Accountability: Make sure AI systems’ decisions are auditable and can be traced back to a specific source.
- Adhere to AI Ethics: Implement ethical guidelines to prevent bias, misinformation, and misuse of AI-generated content.
IBM’s Watsonx.ai emphasizes governance and traceability, ensuring enterprises have access to trustworthy AI that’s both transparent and secure. This governance structure supports the responsible use of AI, mitigating risks that could damage an organization’s reputation.
The Future of LLMs
LLMs are at the forefront of AI’s evolution, with new applications emerging daily. From supporting customer service chatbots to enabling content creation at scale, these models are poised to redefine business processes and human-machine interaction. As LLMs continue to improve, expect them to become even more integral to sectors like healthcare, legal services, and education.
To remain competitive, businesses must prioritize LLM adoption and governance. The ability to leverage LLMs effectively will set industry leaders apart from laggards in the era of AI-driven transformation. By addressing challenges related to hallucinations, biases, and ethical AI, enterprises can unlock the full potential of LLMs to drive efficiency, innovation, and growth.