What Are Foundation Models? Key Characteristics, Applications, and Challenges
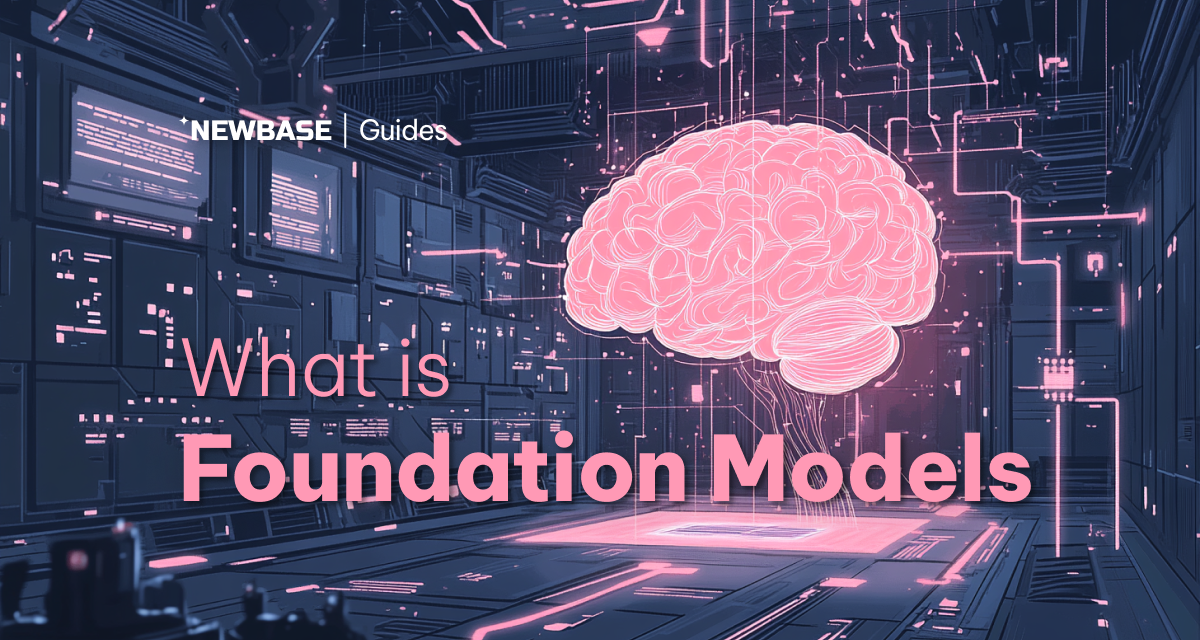
Foundation models are a groundbreaking category of artificial intelligence (AI) models characterized by their immense scale and versatility. These models are trained on vast datasets and designed to perform a broad range of tasks, making them the foundational building blocks for crafting specialized AI applications. Their development represents a paradigm shift in AI, enabling innovations across multiple domains like computer vision, natural language processing (NLP), and robotics.
Key Characteristics of Foundation Models
- Scale and Generality Foundation models stand out for their massive size and flexibility. They are trained on diverse datasets that encompass a wide array of domains, allowing them to adapt to varied tasks without needing extensive retraining. This is in stark contrast to traditional machine learning models, which are typically trained on smaller, domain-specific datasets to accomplish narrow tasks like image classification or predictive analytics.
- Transfer Learning Foundation models leverage transfer learning, a technique that allows them to apply knowledge gained during initial training to new, related tasks. For instance, a model trained for language understanding can be fine-tuned for sentiment analysis, legal document summarization, or medical text interpretation, with minimal additional training.
- Adaptability These models can be adapted through fine-tuning or prompting, making them highly versatile tools for industries requiring customized AI solutions.
The Origin of Foundation Models
The term “foundation models” was coined by researchers at Stanford University’s Center for Research on Foundation Models and Institute for Human-Centered Artificial Intelligence in 2021. In their seminal paper, they described these models as a “paradigm shift,” emphasizing their role as an “incomplete but essential basis” for task-specific adaptations. The name highlights their role as a stable, secure architectural bedrock for future AI applications.
Adapting Foundation Models
Developing a foundation model from scratch is resource-intensive, requiring significant computational power, time, and expertise. As a result, organizations often adapt pre-existing models to suit their unique needs. This adaptation typically follows one of two methods:
- Fine-Tuning involves training a pretrained model on a smaller, domain-specific dataset using supervised learning. This process updates the model’s parameters to optimize its performance for specific tasks. While highly effective, fine-tuning can be time-consuming and may require creating labeled datasets, which is a labor-intensive process. Additionally, altering the model’s parameters might affect its performance on other tasks.
- Prompting is a more lightweight approach that involves giving the model task-specific instructions or examples within the input prompt. This method leverages the model’s “in-context learning” capability to understand and execute tasks without altering its underlying parameters. Although prompting doesn’t require retraining, it may take several iterations to craft effective prompts that yield optimal results.
Real-World Applications of Foundation Models
The general-purpose nature of foundation models allows them to drive innovation in diverse industries:
- Computer Vision Foundation models are instrumental in image generation, classification, and object recognition. Text-to-image models like DALL-E, Imagen, and Stable Diffusion have revolutionized creative industries by enabling AI-generated visuals based on textual descriptions.
- Natural Language Processing (NLP) Large Language Models (LLMs), a subset of foundation models, excel in tasks like text summarization, translation, transcription, and video captioning. Popular NLP foundation models include:
- BERT: Google’s 2018 model that introduced bidirectional contextual understanding.
- GPT Series: OpenAI’s models powering applications like ChatGPT and Microsoft Copilot.
- Claude: Developed by Anthropic, renowned for advanced reasoning and multilingual processing.
- PaLM 2: Google’s next-gen multilingual model with enhanced reasoning capabilities.
- Healthcare Foundation models enable breakthroughs in medical research and patient care by summarizing patient data, answering medical queries, and accelerating drug discovery. For example, Med-PaLM 2 by Google can synthesize insights from medical images and text to provide actionable recommendations.
- Robotics Robotics systems benefit from the adaptability of foundation models to navigate new environments and tasks. Multimodal models like PaLM-E combine language and visual processing capabilities with robotics training data to enhance autonomy and generalization.
- Software Code Generation AI models like Codey and IBM’s Granite Code series assist developers by generating, debugging, and explaining code in multiple programming languages, significantly accelerating software development cycles.
Benefits of Foundation Models
Organizations leveraging foundation models gain several advantages:
- Accelerated Time-to-Value: Pretrained models eliminate the need for extensive development, enabling faster deployment.
- Reduced Data Requirements: Companies can adapt foundation models without needing vast datasets for training.
- High Baseline Accuracy: These models are pretrained and validated, offering a reliable starting point.
- Cost Efficiency: Adapting existing models reduces the expense associated with building AI systems from scratch.
Challenges and Risks
Despite their promise, foundation models face significant challenges:
- Bias: Training data may contain biases that can propagate into the model’s outputs.
- Computational Costs: Even pre-existing models require substantial resources for fine-tuning and deployment.
- Data Privacy: Using sensitive or proprietary data poses risks of intellectual property infringement and data exposure.
- Environmental Impact: Training and operating these large-scale models consume considerable energy, contributing to carbon emissions.
- Hallucinations: Foundation models can generate inaccurate or nonsensical outputs, necessitating thorough validation.
The Future of Foundation Models
As foundational building blocks for modern AI, these models are transforming industries by enabling automation, enhancing decision-making, and unlocking new possibilities. Their continued evolution will likely address current limitations, making them even more robust, efficient, and reliable. By investing in responsible AI practices, organizations can harness the full potential of foundation models to drive innovation and growth in a rapidly changing world.