Understanding Model Collapse: Challenges and Prevention in Generative AI
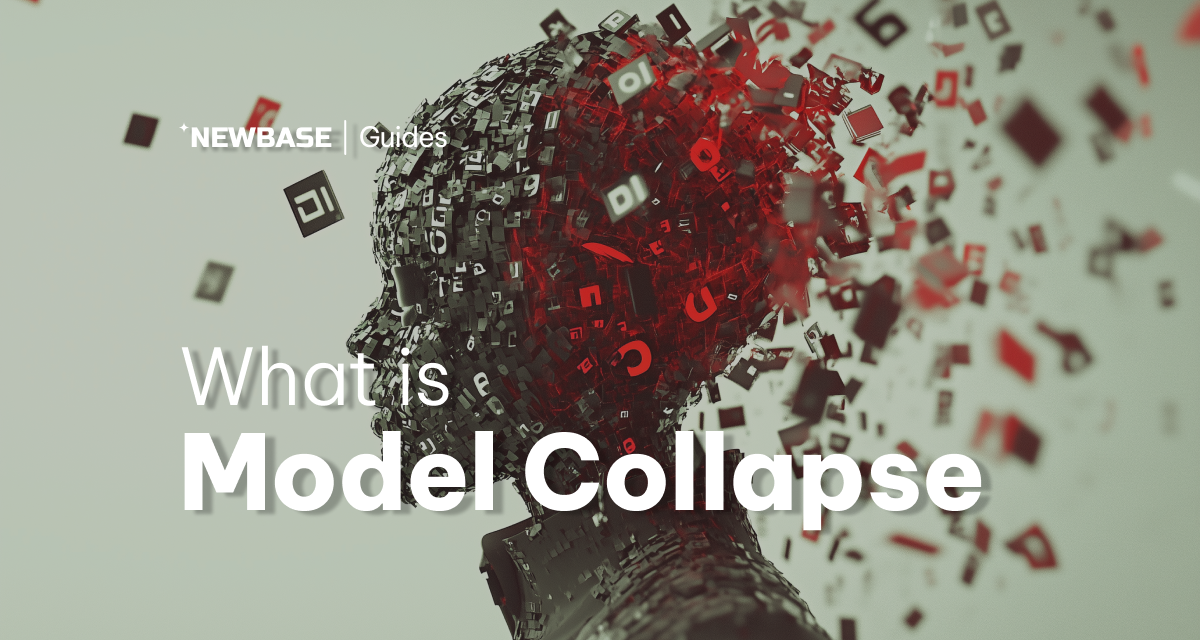
Model collapse refers to the declining performance of generative AI models that are trained on AI-generated content. This degradation stems from the compounding of errors across successive model generations, leading to a divergence from original data distributions and ultimately rendering models less accurate and reliable over time.
A common principle in artificial intelligence (AI) and computer science is that an AI model is only as effective as the quality of the data it is trained on. In recent years, researchers have discovered that generative models trained solely on synthetic, AI-generated data exhibit a phenomenon known as model collapse. These models, plagued by what researchers describe as “irreversible defects,” fail to maintain the diversity and accuracy of the original datasets. Errors introduced during the training of one model generation are propagated and compounded in subsequent iterations, resulting in a progressive decline in performance. This phenomenon can occur in various generative AI systems, such as large language models (LLMs), image-generation models, and others.
How Does Model Collapse Occur?
Generative AI models produce datasets with less variation than the original data distributions. This lack of diversity accelerates model collapse through two key stages:
Early Model Collapse:
- Initial loss of information occurs at the extremes (tails) of the data distribution.
- Models trained on AI-generated data begin to lose rare and low-probability features, which are critical for preserving the richness of the data.
Late Model Collapse:
- The data distribution becomes increasingly narrow and homogenous.
- Successive model iterations produce outputs that deviate significantly from the original data, ultimately becoming unrecognizable and less functional.
In experiments conducted by researchers like Ilia Shumailov and colleagues, models trained on synthetic data consistently demonstrated this pattern of collapse. Their findings underscore the importance of maintaining access to high-quality, human-generated datasets to mitigate this issue.
Real-World Implications of Model Collapse
As AI-generated content becomes more prevalent, the risks associated with model collapse extend to various applications, with significant consequences for businesses, users, and knowledge systems.
Poor Decision-Making:
Inaccurate outputs resulting from model collapse can have costly repercussions for organizations that rely on AI for decision-making. For instance:
- Healthcare: Diagnostic models trained on degraded data might fail to identify rare diseases, compromising patient outcomes.
- Customer Service: AI-powered chatbots might provide irrelevant or misleading responses, frustrating users and damaging brand reputation.
User Disengagement:
When AI models discard outlying data points, users seeking unique or less common outputs may feel alienated. Examples include:
- Recommendation Systems: A consumer with niche preferences, such as a preference for lime green shoes, may consistently receive recommendations for mainstream products, driving them to competitors.
- Content Platforms: AI-generated content that prioritizes popular trends over niche or diverse topics could fail to meet user expectations.
Knowledge Decline:
AI systems undergoing model collapse risk perpetuating narrower outputs, potentially erasing long-tail ideas and limiting human knowledge. For example:
- Scientific Research: AI-powered tools that rely on degraded data might exclude valuable yet less-cited studies, hindering innovation and discovery.
- Cultural Diversity: AI models trained on homogenized data could overlook unique linguistic, cultural, or artistic expressions.
Impacts Across Different Generative AI Models
Model collapse manifests differently across various types of generative AI systems:
Large Language Models (LLMs):
- Symptoms include irrelevant, nonsensical, or repetitive text outputs.
- In one study, successive generations of an LLM trained on AI-generated data shifted from meaningful responses to incoherent outputs, such as producing content about unrelated topics like “jack rabbits with different-colored tails.”
Image-Generating Models:
- Outputs become less diverse, precise, and realistic over time.
- Experiments with Variational Autoencoders (VAEs) and Generative Adversarial Networks (GANs) revealed diminishing quality and homogeneity in visual outputs, such as indistinguishable handwritten digits or overly similar human faces.
Gaussian Mixture Models (GMMs):
- Models tasked with clustering data exhibited significant performance degradation after successive iterations. By the 2000th iteration, the output displayed minimal variance, highlighting the compounded impact of training on synthetic data.
Model Collapse vs. Other Model Degradation Phenomena
Model collapse is part of a broader category of issues affecting machine learning models but remains distinct from related phenomena:
Catastrophic Forgetting:
- Occurs when a single model forgets previously learned information while acquiring new knowledge.
- Unlike model collapse, this happens within a single model rather than across generations.
Model Collapse:
- Specific to GANs, this occurs when the generator produces outputs lacking variance, resulting in repetitive and unvaried data.
- In contrast, model collapse spans across various generative AI architectures.
Model Drift:
- Refers to performance degradation due to changes in input data or relationships over time.
- Model collapse is distinct as it stems from training on AI-generated data, rather than external changes.
Performative Prediction:
- Occurs when a model’s outputs influence real-world outcomes, creating feedback loops that reinforce its predictions.
- Unlike model collapse, this phenomenon is typically observed in supervised learning models.
How Can Model Collapse Be Prevented?
To counteract model collapse, researchers and developers are exploring various strategies:
Retaining Non-AI Data Sources:
- Ensuring access to high-quality, human-generated data helps preserve the diversity and accuracy of training datasets.
Determining Data Provenance:
- Tracking the origins of data ensures a clear distinction between human-generated and synthetic content.
- Initiatives like The Data Provenance Initiative audit datasets to maintain transparency and reliability.
Leveraging Data Accumulation:
- Combining real data with synthetic data across multiple generations can mitigate performance degradation.
Using Better Synthetic Data:
- Improving the quality of synthetic data through advanced algorithms enhances its reliability for training purposes.
Implementing AI Governance Tools:
- Tools for monitoring bias, drift, and anomalies can detect early signs of model collapse, allowing for timely intervention.
Conclusion
Model collapse represents a critical challenge in the development of generative AI systems. As errors compound through successive generations of AI-generated data, the risk of degraded performance threatens the reliability and utility of these models. By prioritizing high-quality training data, enhancing synthetic data generation, and employing robust governance tools, researchers and developers can address this phenomenon and ensure the continued evolution of generative AI.