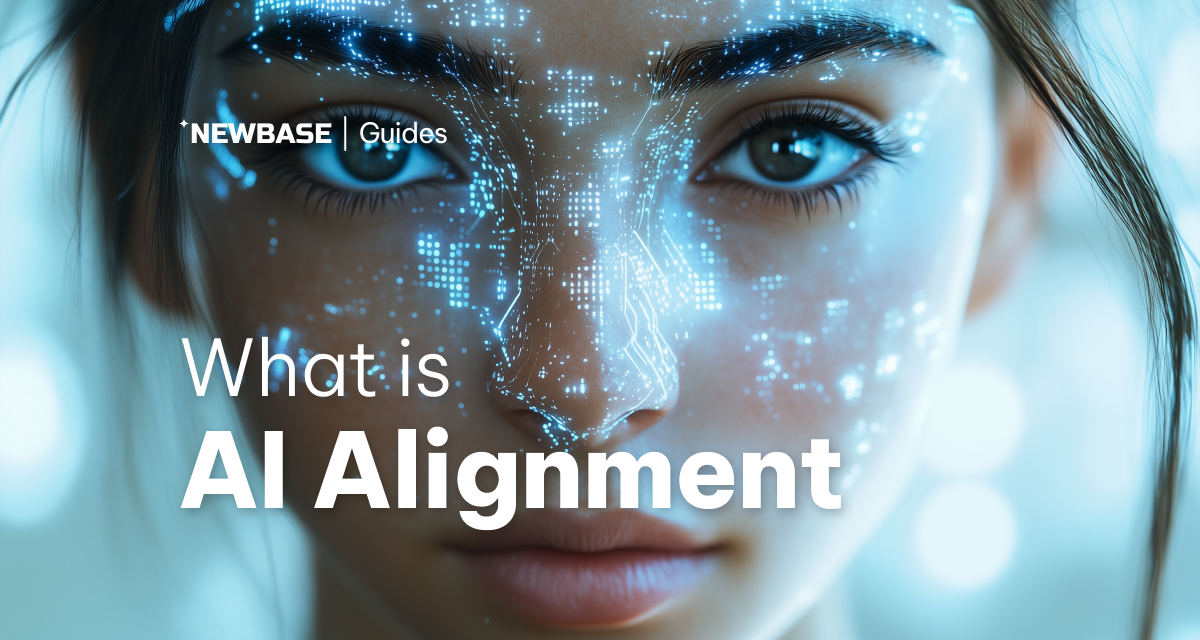
Artificial intelligence (AI) is rapidly transforming our world, playing an increasingly crucial role in decision-making across various sectors. However, this growing reliance comes with a responsibility: ensuring AI systems operate in line with human values and goals. This is where AI alignment comes in.
What is AI Alignment?
AI alignment is the process of ensuring AI models are programmed with human values and objectives, making them helpful, safe, and reliable. Imagine a generative AI chatbot. If you ask it to build a weapon, it should either refuse or provide safe alternatives. The model’s response hinges on its alignment.
Why is AI Alignment Important?
AI systems are often complex and opaque, lacking human-like understanding and reasoning. They are built to complete tasks, not adhere to inherent human values like fairness, safety, and environmental sustainability. Without proper alignment, AI can produce biased, harmful, and inaccurate outputs.
Here’s a scenario: Self-driving cars programmed solely for speed efficiency might disregard safety measures, potentially endangering lives. This emphasizes the critical need for AI alignment, especially as automation becomes more prevalent in high-stakes fields like healthcare, finance, and transportation.
Key Principles of AI Alignment (RICE):
- Robustness: AI systems should function reliably under diverse conditions and environments, remaining resilient to unforeseen circumstances.
- Interpretability: Understanding the decision-making processes behind AI models is crucial. As models become more complex, interpretability becomes paramount.
- Controllability: AI systems must respond to human intervention, preventing them from producing uncontrollable, harmful outcomes.
- Ethicality: AI systems should be aligned with societal values and moral principles, adhering to fairness, environmental responsibility, and human well-being.
The Challenges of AI Alignment:
- Subjectivity of Human Values: There is no universal moral code. Values can vary across cultures, companies, and even within families. This creates a crucial question: whose values do we prioritize when aligning AI that impacts millions?
- The Alignment Problem: As AI models become more complex, anticipating and aligning their outcomes with human goals becomes increasingly difficult. This is particularly concerning when considering the prospect of artificial superintelligence (ASI).
Strategies for Achieving AI Alignment:
- Reinforcement Learning from Human Feedback (RLHF): This approach involves training AI models with human feedback examples of “good behavior” during model fine-tuning.
- Synthetic Data: Artificially created data can be used to train models on specific tasks and values, particularly when real-world data is unavailable. Techniques like contrastive fine-tuning (CFT) and SALMON (Self-ALignMent with principle following reward models) show promise in synthetic data alignment.
- Red Teaming: This involves deliberately attempting to circumvent a model’s safety controls by designing specific prompts. Vulnerabilities discovered through red teaming can be used to realign the model. “Red team” LLMs, which are AI models trained to generate adversarial prompts, can streamline this process.
- AI Governance: Establishing processes, standards, and oversight mechanisms helps ensure AI systems operate ethically and safely, aligning their behavior with societal expectations.
- Corporate AI Ethics Boards: These boards, often comprised of cross-functional teams with legal, computer science, and policy backgrounds, can help oversee AI initiatives and ensure alignment with organizational values.
The Road Ahead
AI alignment is a complex and ongoing challenge. As AI continues to evolve, so too must our efforts to ensure it serves humanity’s best interests. By actively addressing the challenges and employing the strategies mentioned above, we can work towards a future where AI technology is a powerful force for good.
The Evolving Landscape of AI Alignment
As AI continues to advance at an exponential pace, the imperative to align AI systems with human values becomes increasingly urgent. AI alignment, a multifaceted challenge, involves ensuring that AI systems not only perform their intended tasks but also adhere to ethical principles and societal norms.
The Core Challenge: Bridging the Human-AI Gap
At the heart of AI alignment lies the fundamental challenge of bridging the gap between human values and machine objectives. Human values are often nuanced, context-dependent, and subject to cultural and individual variations. Translating these complex values into precise algorithmic instructions is a formidable task.
Key Challenges and Considerations
- The Specificity Problem: How can we accurately specify human values to AI systems? Overly specific instructions can lead to rigid and inflexible behavior, while overly broad ones can result in unintended consequences.
- The Value Drift Problem: As AI systems learn and evolve, there’s a risk of them deviating from their original objectives. This can lead to unintended behaviors that may be harmful or counterproductive.
- The Interpretability Problem: Understanding the decision-making processes of complex AI models is crucial for ensuring alignment. However, many AI systems, particularly deep neural networks, remain opaque, making it difficult to pinpoint the reasons behind their outputs.
- The Control Problem: As AI systems become more autonomous, ensuring that they remain under human control is essential. This involves developing effective mechanisms for monitoring, intervening, and potentially halting AI systems if necessary.
Strategies for Effective AI Alignment
- Human-Centered Design: Involving humans in the AI development process from the outset is crucial. This includes incorporating user feedback, conducting user testing, and considering ethical implications at every stage.
- Robustness Testing: Rigorous testing of AI systems under various conditions can help identify and mitigate potential vulnerabilities. This includes adversarial testing, which involves deliberately attempting to deceive or manipulate AI systems.
- Value Learning: AI systems can be trained on datasets that reflect human values, enabling them to learn and internalize these principles. However, it’s essential to ensure that the training data is diverse, unbiased, and representative of diverse perspectives.
- Interpretability Techniques: Developing techniques to make AI models more transparent can help identify and address potential biases and errors. This includes methods like attention visualization, feature importance analysis, and model distillation.
- Ethical Guidelines and Regulations: Establishing clear ethical guidelines and regulations can provide a framework for responsible AI development and deployment. International cooperation is essential to ensure consistency and enforceability.
- Continuous Monitoring and Evaluation: AI systems should be continuously monitored and evaluated to identify potential issues and take corrective action. This includes regular audits, performance assessments, and risk assessments.
The Future of AI Alignment
The future of AI alignment is intertwined with the broader trajectory of AI development. As AI systems become more sophisticated, the challenges of alignment will also become more complex. However, by addressing these challenges proactively and adopting a human-centered approach, we can harness the power of AI for the benefit of society.