RAG (Retrieval-Augmented Generation): Boosting AI Performance with External Knowledge
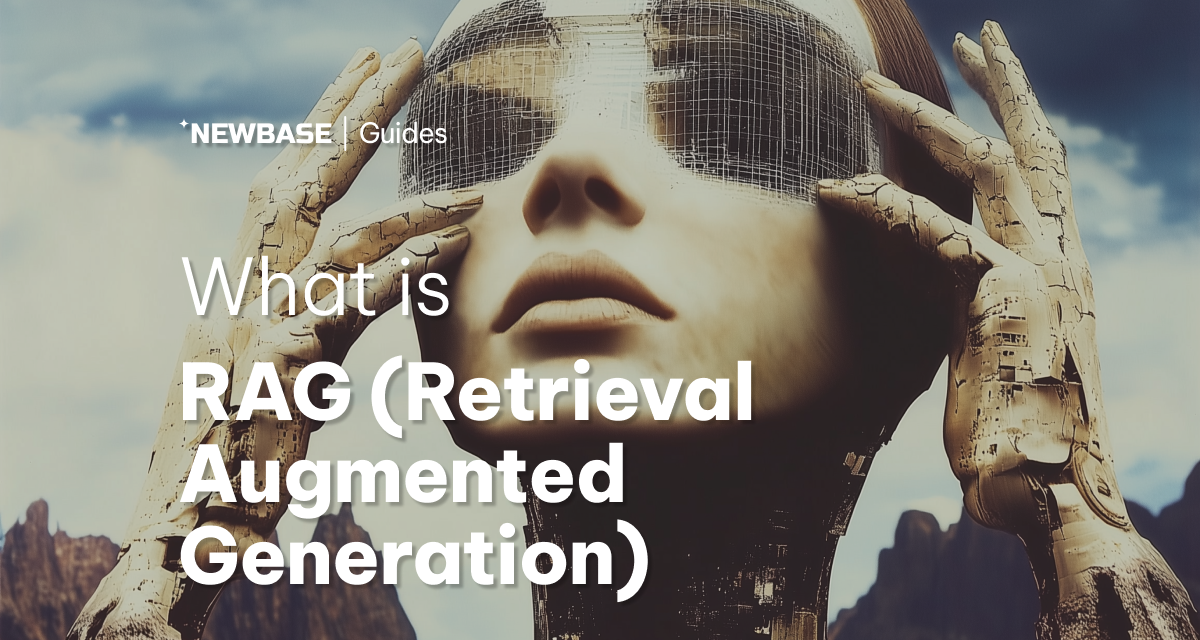
Large language models (LLMs) power many AI applications, but their reliance on static training data can limit their accuracy and effectiveness. Retrieval-Augmented Generation (RAG) addresses this by connecting LLMs with external knowledge bases, significantly improving the quality and domain-specific capabilities of AI systems.
Why Use RAG?
- Cost-Effective and Scalable: Avoids costly retraining for specific use cases. RAG leverages existing LLM knowledge and integrates relevant data from internal sources or real-time feeds.
- Access to Current Information: Combats the “knowledge cutoff” issue of LLMs. RAG ensures access to up-to-date information, enhancing response accuracy.
- Reduced Risk of AI Hallucinations: RAG grounds LLMs in factual data, minimizing the generation of incorrect or made-up information.
- Increased User Trust: RAG models can cite their sources, allowing users to verify outputs and gain confidence in the system’s reliability.
- Expanded Use Cases: Access to more data broadens the range of prompts an LLM can handle, leading to more versatile applications.
- Enhanced Developer Control: RAG simplifies model maintenance by allowing adjustments to external data sources rather than retraining the LLM itself.
- Greater Data Security: RAG preserves data security by connecting the LLM to external databases without incorporating that data into its core training.
RAG Applications
- Specialized Chatbots and Virtual Assistants: Equip customer support chatbots with deep product and policy knowledge.
- Research: Generate client-specific reports or facilitate research by accessing internal documents and search engines.
- Content Generation: Create reliable content with citations to authoritative sources, enhancing user trust and output accuracy.
- Market Analysis and Product Development: Analyze social media trends, competitor activity, and customer feedback to inform business decisions.
- Knowledge Engines: Empower employees with internal information, streamlining onboarding processes and providing on-demand guidance.
- Recommendation Services: Generate more accurate recommendations by analyzing user behavior and comparing it with current offerings.
How Does RAG Work?
- User Prompt: A user submits a question or request.
- Information Retrieval: The RAG system searches a knowledge base for relevant data based on the user prompt.
- Data Integration: Retrieved information is combined with the user query to create an enriched prompt.
- LLM Generation: The enhanced prompt is fed to the LLM, which generates a response informed by both the user input and retrieved data.
- User Output: The user receives the final response.
Key Components of a RAG System
- Knowledge Base: External data repository (documents, PDFs, websites) that feeds the system.
- Retriever: An AI model that searches the knowledge base for relevant data based on the user prompt.
- Integration Layer: Coordinates the overall functioning of the RAG system, processing retrieved data and user queries.
- Generator: The LLM that creates the final output based on the enriched prompt.
Additional components might include:
- Ranker: Ranks retrieved data based on relevance to the user prompt.
- Output Handler: Formats the generated response for the user.
Building a Strong Knowledge Base
- Data Preparation: Knowledge bases can contain unstructured data, requiring transformation into numerical representations (vectors) for efficient searching.
- Chunking: Documents are broken down into smaller chunks to ensure retrieved information aligns with the LLM’s context window.
- Continuous Updates: Regularly updating the knowledge base is crucial to maintain the system’s accuracy and relevance.
RAG vs. Fine-Tuning
While both methods aim to improve LLM performance, they differ in approach:
- RAG: Allows an LLM to query external data sources at runtime.
- Fine-tuning: Trains an LLM on a new dataset specific to the desired domain.
RAG and fine-tuning can be complementary. Fine-tuning helps an LLM understand the domain, while RAG provides access to relevant real-time data to create high-quality outputs.
By leveraging external knowledge, RAG empowers LLMs to deliver more accurate, relevant, and trustworthy results, unlocking the full potential of AI for an array of applications.