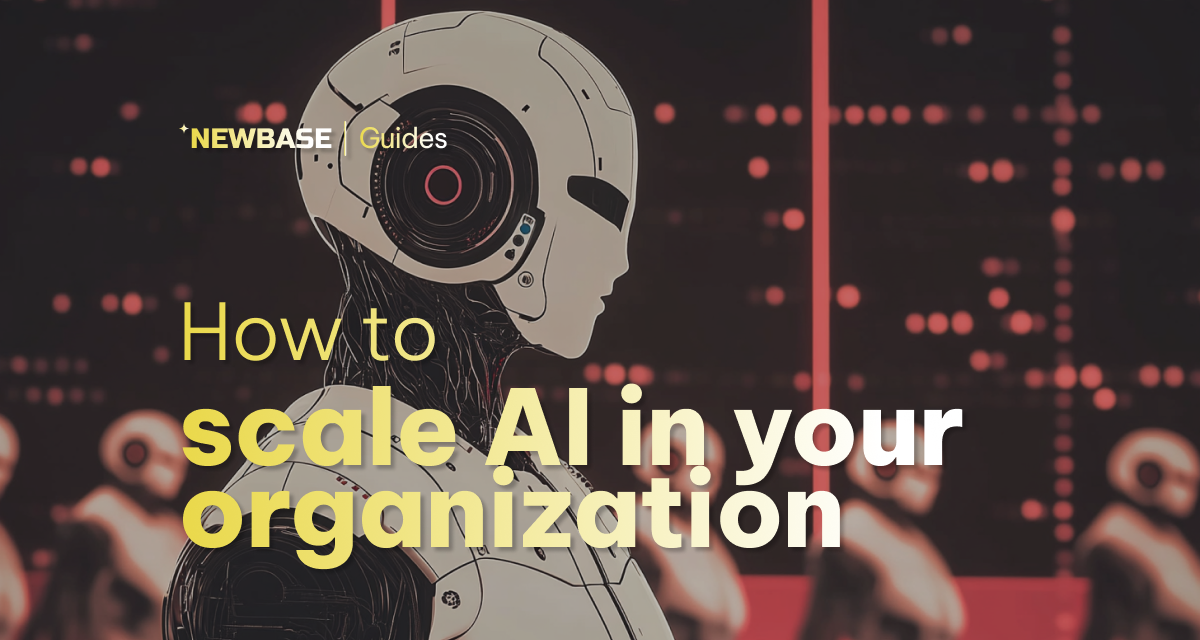
Adopting artificial intelligence (AI) at scale has become a transformative trend across various industries, including manufacturing, healthcare, retail, and financial services. This shift marks the transition from isolated pilot projects to comprehensive digital transformation initiatives, where AI becomes a core component of operations. However, scaling AI is a multifaceted endeavor that requires more than just deploying a few models. It demands strategic planning, robust infrastructure, collaboration across departments, and a proactive approach to overcoming challenges.
The Strategic Value of Scaling AI
When deeply integrated into an organization’s operations, AI delivers transformative value. Key applications include:
- Modernizing Data Management: AI can streamline data collection, integration, and analysis, turning raw data into actionable insights.
- Automating Workflows: AI-driven automation enhances productivity by reducing manual interventions in repetitive tasks.
- Transforming Customer Experiences: Tools such as AI-powered chatbots and recommendation engines provide personalized and efficient customer interactions.
- Driving Innovation with Generative AI: Generative AI can produce original content, modernize codebases, and accelerate creative processes, significantly boosting productivity and innovation.
Scaling AI, however, presents unique challenges. As AI initiatives expand, complexities such as performance degradation, limited visibility into model behavior, and growing data volumes emerge. Addressing these issues requires a deliberate focus on governance, security, and cross-functional collaboration.
Critical Enablers for AI Scaling
Organizations aiming to scale AI must invest in key enablers that support operational and strategic goals:
- Feature Stores and Code Repositories: These centralized resources streamline AI model development by providing reusable assets and ensuring consistency.
- Machine Learning Operations (MLOps): MLOps establishes a robust framework for developing, deploying, and maintaining AI models efficiently, reducing time-to-market and ensuring reliability.
- Cloud-Based AI Platforms and APIs: By leveraging cloud services and APIs, organizations can democratize AI access, enabling more teams to benefit from advanced capabilities without requiring extensive technical expertise.
- Hybrid Cloud Infrastructure: A hybrid approach facilitates secure and flexible AI deployment across diverse IT environments, ensuring scalability and compliance with organizational policies.
Steps to Successfully Scale AI
Scaling AI is a complex process that involves strategic planning, robust execution, and continuous optimization. Below are the key steps to ensure success:
1. Collaborate with Data Science Experts
Partner with skilled data scientists and machine learning professionals to design algorithms aligned with your business objectives. Employ tools such as large language models (LLMs) and specialized APIs to customize solutions for specific use cases, driving innovation and operational efficiency.
2. Identify and Prepare Data Sources
AI models thrive on high-quality data. Identify relevant data sources—both internal (e.g., customer records, operational logs) and external (e.g., market trends, research reports). Invest in data cleaning and preparation to ensure accuracy, consistency, and relevance.
3. Engage Stakeholders Across Departments
AI initiatives should involve stakeholders from various functions, such as marketing, finance, customer service, and IT. Cross-departmental collaboration ensures that AI solutions address diverse challenges and align with overarching business goals.
4. Implement Robust Data Lifecycle Management
Establish secure, standardized data structures to manage data throughout its lifecycle. Regular updates and maintenance keep data relevant for training, validating, and refining AI models.
5. Optimize MLOps for Scalability
Adopt MLOps platforms that integrate seamlessly with your IT infrastructure and cloud ecosystem. These platforms should provide capabilities for monitoring, version control, and automated deployment, ensuring scalability and reliability.
6. Form Cross-Functional AI Teams
Create multidisciplinary teams that include data scientists, IT engineers, and business leaders. This structure fosters collaboration, enhances understanding of business requirements, and promotes innovation.
7. Prioritize High-Impact Projects
Focus on projects with significant potential for success, such as automating customer service processes or modernizing legacy systems. Early successes build organizational confidence and pave the way for more ambitious AI initiatives.
8. Embed Governance and Compliance
Incorporate AI governance and compliance mechanisms from the start. Use tools with built-in governance features to ensure ethical practices, regulatory adherence, and transparency in AI operations.
9. Leverage Advanced Tools and Platforms
Provide cloud-based environments that enable experimentation, development, and scaling of AI models. Such platforms foster collaboration among data scientists, engineers, and business users while streamlining governance and compliance efforts.
10. Monitor and Optimize AI Models
Track AI performance across key metrics, such as speed, accuracy, and cost efficiency. Use real-time monitoring and KPI tracking to identify issues early and optimize performance over time.
Overcoming Challenges in Scaling AI
Scaling AI often involves navigating complex challenges that require proactive strategies and resource investment:
1. Data Management and Security
AI relies on diverse and voluminous data sources, ranging from structured databases to unstructured content like images and videos. Organizations must invest in scalable data storage solutions, such as cloud-based data lakehouses, and implement robust data security measures to protect sensitive information.
2. Cross-Functional Collaboration
Scaling AI is inherently collaborative. Business experts, IT professionals, and data scientists must work together to ensure that AI outputs align with strategic objectives. Techniques like Retrieval Augmented Generation (RAG) can enhance AI outcomes by leveraging organizational knowledge without modifying underlying models.
3. Selecting the Right Tools
AI scaling requires tools that cater to the needs of data scientists, IT teams, and business users. Integrated platforms such as MLOps streamline development, deployment, and monitoring, enabling efficient and scalable operations.
4. Addressing Talent Shortages
The demand for AI expertise often exceeds supply. Organizations can mitigate this challenge by leveraging pre-trained models, cloud-based APIs, and automated MLOps solutions, reducing reliance on specialized talent.
5. Defining an Appropriate Scope
Start with well-defined, manageable projects to build momentum. Early wins create a foundation of trust and expertise, enabling the organization to expand AI initiatives incrementally.
6. Allocating Time and Resources
Scaling AI requires significant investment in time and effort, particularly in data preparation, integration, and monitoring. Automation tools, open-source libraries, and strategic partnerships can accelerate these processes.
The Path to AI-Driven Transformation
Scaling AI is not merely a technological endeavor; it’s a holistic transformation that reshapes how an organization operates, innovates, and competes. By addressing enablers and challenges, fostering collaboration, and maintaining a clear strategic vision, organizations can unlock AI’s full potential. This journey, while demanding, ultimately positions AI as a cornerstone of sustainable growth and competitive advantage.