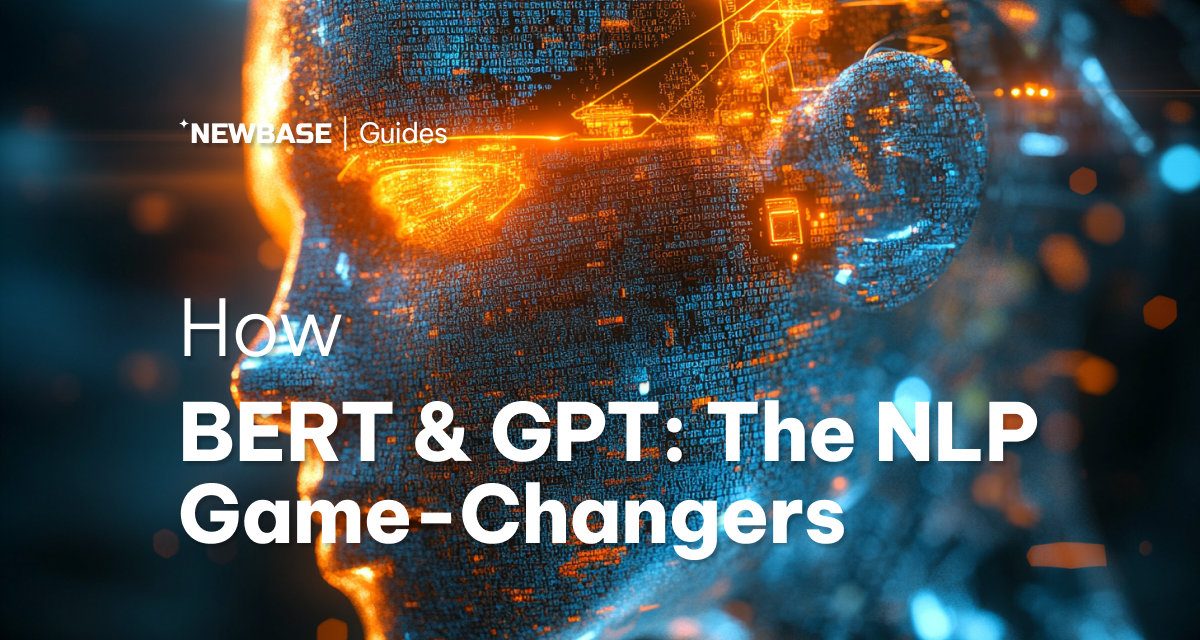
The field of Natural Language Processing (NLP) has undergone a dramatic transformation, thanks to the development of BERT (Bidirectional Encoder Representations from Transformers) and GPT (Generative Pre-Trained Transformer) models. These groundbreaking deep learning models have significantly enhanced the capabilities of NLP applications, enabling more accurate language understanding, generation, and contextual reasoning. This article explores the core concepts behind BERT and GPT models, how they differ, their key benefits, and the impact they have on NLP and AI-driven business applications.
The Evolution of Deep Learning for NLP
Traditional NLP models faced several limitations, particularly in handling long-term dependencies and understanding the context of words within sentences. Early models, like Recurrent Neural Networks (RNNs) and Long Short-Term Memory (LSTM) networks, processed sequences word-by-word, making it challenging to retain context over extended text inputs.
The introduction of Transformers in 2017 marked a major breakthrough in NLP. Transformers employ an attention mechanism that allows models to process entire sequences of text simultaneously, identifying relationships between words regardless of their distance in the text. This architecture enabled significant improvements in computational efficiency, parallelization, and contextual understanding. From this development emerged two landmark models: BERT and GPT.
What Makes BERT Special?
BERT, introduced by Google in 2018, redefined NLP by introducing bidirectional context learning. This bidirectional approach allows BERT to understand words in the context of words that come both before and after them. Unlike earlier models that only considered the left-to-right context, BERT’s ability to process context from both directions simultaneously results in a deeper understanding of word relationships.
Key Features of BERT:
- Bidirectional Contextual Learning: BERT’s primary innovation is its bidirectional attention, which enables it to predict masked words in a sentence based on context from both sides. For instance, in the sentence, “The [MASK] was filled with water,” BERT can use clues from both the left (“The”) and right (“was filled with water”) to determine that “glass” is a likely fit for the masked position.
- Pretraining with Masked Language Model (MLM) and Next Sentence Prediction (NSP): BERT’s training approach involves randomly masking words in sentences and training the model to predict them. Additionally, it uses NSP to understand the relationship between two sentences, which aids in tasks like question-answering and reading comprehension.
- Versatility in NLP Tasks: BERT’s pretraining makes it adaptable to a wide variety of downstream NLP tasks, such as text classification, sentiment analysis, and named entity recognition. Fine-tuning requires relatively small datasets, saving both time and computational resources.
Use Cases for BERT:
- Text Classification: BERT’s contextual understanding enhances spam filtering, sentiment analysis, and document categorization.
- Question-Answering Systems: BERT can identify and extract answers from larger text passages.
- Natural Language Inference (NLI): BERT enables models to classify the relationship between premise statements and hypotheses (e.g., determining if a statement is true, false, or undetermined).
What Sets GPT Apart?
While BERT focuses on bidirectional understanding, GPT models excel in text generation. The original GPT model, introduced by OpenAI, was succeeded by GPT-2, GPT-3, and the more advanced GPT-4. GPT-3, with 175 billion parameters, was one of the most powerful NLP models of its time. GPT-4, however, marked a significant leap forward, featuring enhanced reasoning, creativity, and contextual understanding capabilities, as well as support for both text and image inputs. Unlike BERT, which uses a bidirectional approach, GPT models employ a unidirectional strategy, predicting the next word in a sequence based on prior context. This evolution in GPT models has enabled more sophisticated and nuanced AI interactions across a wide range of applications.
Key Features of GPT:
- Unidirectional Attention: GPT generates language by predicting the next word in a sequence, similar to how humans might write. This design is optimized for generative language tasks such as text completion and content creation.
- Massive Pretraining on Diverse Data: GPT-3’s training on 175 billion parameters from a vast range of online sources enables it to perform tasks with minimal fine-tuning. Few-shot, one-shot, and zero-shot learning capabilities mean it can generate content with little to no prior exposure to task-specific data. Building on this foundation, GPT-4 features an even larger and more sophisticated architecture, with improved reasoning, creativity, and understanding of nuanced instructions. It supports multimodal input (allowing image and text inputs) and exhibits better performance in tasks requiring complex problem-solving and multilingual comprehension, making it more adaptable across a wider range of applications.
- Contextual Language Generation: GPT’s strength lies in its ability to generate coherent, context-aware text for tasks like essay writing, code generation, and chatbots.
Use Cases for GPT:
- Content Generation: GPT-3 can write essays, product descriptions, and creative writing with minimal human input.
- Conversational AI: Many AI chatbots and virtual assistants use GPT’s language generation capabilities for natural and human-like conversations.
- Code Generation: GPT-3 can generate programming scripts and code snippets based on plain-language descriptions.
Key Differences Between BERT and GPT
Feature | BERT | GPT |
---|---|---|
Training | Bidirectional | Unidirectional (left-to-right) |
Task Focus | Language understanding | Language generation |
Use Case | Classification, NLI, QA | Text generation, chatbots |
Pretraining | Masked language model | Next-word prediction |
Parameter Count | BERT-Base: 110M | GPT-3: 175B |
BERT’s bidirectional design makes it ideal for understanding and classification tasks, while GPT’s unidirectional approach allows for coherent text generation.
How Do BERT and GPT Models Benefit NLP?
The emergence of BERT and GPT models has had a transformative impact on NLP. These models offer several key benefits:
- Improved Contextual Understanding: BERT’s bidirectional training enables nuanced language understanding, helping models disambiguate words with multiple meanings (e.g., “bank” as a financial institution vs. a riverbank).
- Advanced Text Generation: GPT’s unidirectional, predictive training allows for natural, human-like text generation for chatbots, AI companions, and creative writing.
- Reduced Data Requirements: Transfer learning allows for fine-tuning on smaller datasets, making BERT and GPT more accessible to companies without massive labeled datasets.
- Higher Efficiency and Scalability: Transformers’ parallel processing architecture facilitates faster model training and inference, enabling larger and more complex models.
The Future of NLP with BERT and GPT
The ongoing development of BERT, GPT, and similar models like RoBERTa and T5 reflects the rapid pace of NLP advancements. The models are already being used in diverse applications like AI-driven search engines, personalized recommendations, and conversational AI. However, challenges remain, such as biases in AI-generated text and the need for more explainable AI.
Several emerging trends point to the future of NLP:
- Hybrid Models: Combining the bidirectional learning of BERT with the generative capabilities of GPT could lead to more robust AI systems.
- Smaller, Efficient Models: While GPT-3’s 175B parameters are impressive, research is focused on creating smaller, more efficient models that deliver similar performance with less computational overhead.
- Advanced Multilingual NLP: Cross-lingual models are expected to offer seamless NLP capabilities across multiple languages.
Conclusion
BERT and GPT have redefined the landscape of NLP, introducing new capabilities in language understanding and generation. BERT’s bidirectional context awareness and GPT’s generative prowess have found applications in everything from chatbots to search engines. As research continues, hybrid models and efficiency improvements will further enhance the transformative power of NLP, enabling smarter AI-driven applications for business and society at large. As we look to the future, the possibilities for NLP innovation are boundless.