Foundations of Trustworthy AI: How to Conduct Trustworthy AI Assessment and Mitigation
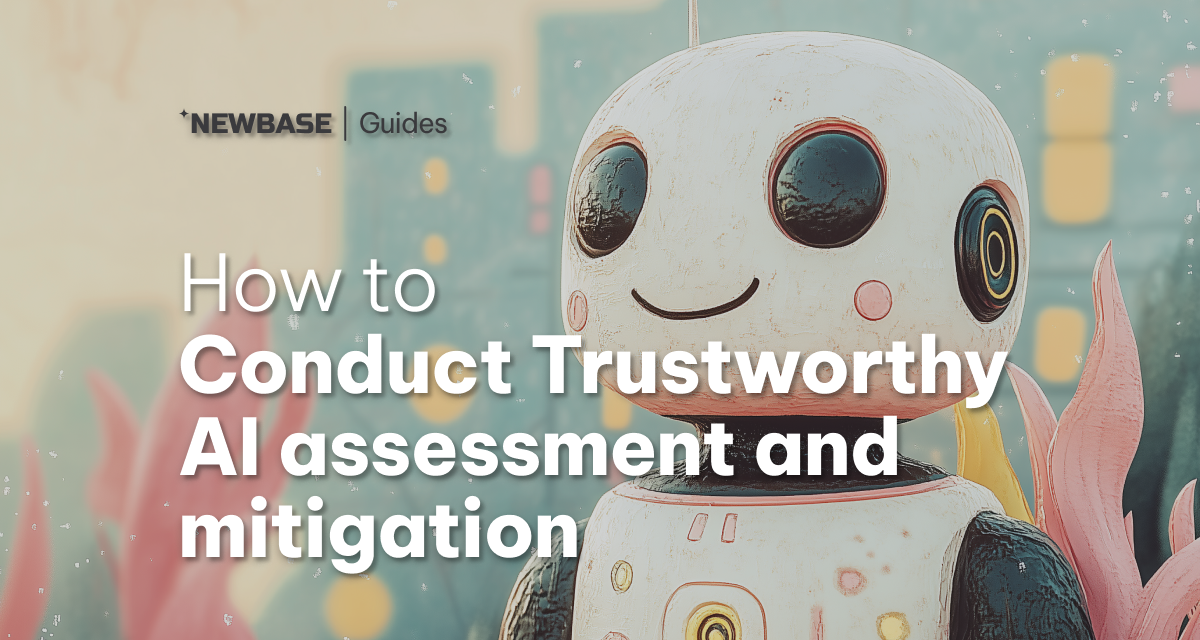
Artificial intelligence (AI) has evolved from theoretical concepts to a transformative force driving essential business processes. As AI applications increase in complexity and impact, the need for trustworthy AI becomes paramount. Ensuring trust in AI requires a robust framework to assess and mitigate risks related to privacy, robustness, fairness, explainability, and transparency — collectively known as the five pillars of trustworthy AI. This article delves into these five pillars, the process of assessment, and strategies for effective mitigation.
The Five Pillars of Trustworthy AI
Privacy
Privacy ensures that sensitive information is safeguarded against both accidental leaks and deliberate breaches. With AI, the challenge extends to protecting data used in training models. For instance, when training a mortgage approval AI system on historical data, it is crucial to prevent sensitive information, like an applicant’s income, from being inferred through indirect methods. Ensuring privacy requires data encryption, anonymization, and the implementation of secure access controls.
Robustness
Robustness refers to an AI system’s ability to maintain accurate performance in varying conditions, whether those changes occur naturally or are induced by malicious actors. A robust mortgage approval AI should continue making sound decisions even amid disruptive events, such as a financial crisis. Methods to improve robustness include adversarial testing, stress testing, and data augmentation to ensure stability under different operating conditions.
Fairness
Fairness guarantees that AI systems do not exhibit systematic bias in favor of or against specific groups, often defined by attributes like gender, race, or nationality. For instance, a fair mortgage approval AI should not exhibit bias in how it assesses applications from individuals of different demographic groups. Fairness can be promoted by using balanced datasets, conducting fairness audits, and using mitigation techniques to adjust biased model predictions.
Explainability
Explainability enables stakeholders to understand how an AI system makes decisions. For a mortgage approval AI, explainability allows loan officers, applicants, and regulators to comprehend how specific inputs (e.g., credit score, income, and debt-to-income ratio) affect loan decisions. Techniques like SHAP (SHapley Additive exPlanations) and LIME (Local Interpretable Model-agnostic Explanations) are used to make AI decision-making more transparent and understandable.
Transparency
Transparency involves clear documentation and disclosure of AI system design, decisions, and performance assessments. Stakeholders, including regulators, employees, and customers, should have access to “AI FactSheets” that document the model’s purpose, development process, risks, and performance metrics. Transparency builds trust and accountability, as it provides visibility into how AI models operate and how they are governed.
Conducting Trustworthy AI Assessments
Why Assess Trustworthiness?
Similar to inspecting a house for safety and functionality, AI must be assessed to ensure its safety, reliability, and ethical use. Various stakeholders — such as regulators, developers, customers, and internal teams — have different motivations for conducting assessments. For instance, regulators may assess AI to ensure compliance, while developers assess it to improve performance. These assessments occur at various points in the AI lifecycle: during development, before deployment, during production, and after adverse events.
What Should Be Assessed?
Both the AI model and the data it uses must be tested for compliance with the five pillars of trustworthiness. Key steps in this process include:
- Defining Performance Indicators: Each pillar has unique performance indicators. For instance, privacy may be measured by data re-identification risk, robustness by model stability under adversarial attacks, fairness by disparate impact ratios, explainability by interpretability scores, and transparency by the completeness of AI FactSheets.
- Generating Test Data: To assess AI under realistic and extreme conditions, both historical and synthetic test data are required. Withholding a portion of training data for testing, generating stress test scenarios, and using adversarial inputs can reveal vulnerabilities and areas for improvement.
When to Assess AI?
AI assessments should be conducted throughout the entire AI lifecycle, from initial development to continuous monitoring in production. This ensures early identification of issues and supports continuous improvement. Specific assessment phases include:
- Development Stage: Identify potential risks during design and development.
- Validation Stage: Verify compliance with regulatory standards before deployment.
- Production Stage: Continuously monitor performance to detect drifts or anomalies.
- Post-Incident Stage: Assess after adverse events to identify root causes and corrective actions.
Mitigation of Trustworthiness Issues
If assessments reveal issues with privacy, robustness, fairness, explainability, or transparency, organizations must take immediate action to address them. Mitigation strategies are categorized into three key approaches:
Pre-Processing Techniques
These techniques aim to address issues before model training begins. For example, if bias is detected in training data, pre-processing methods like re-sampling, re-weighting, or adversarial de-biasing can be applied to improve fairness. Similarly, to ensure privacy, data anonymization or differential privacy methods can be used.
In-Processing Techniques
These methods intervene during model training. For example, fairness constraints can be applied to the optimization function, ensuring that the model’s predictive power is balanced across demographic groups. Robustness can be improved by incorporating adversarial training, which teaches the model to recognize and handle adversarial inputs.
Post-Processing Techniques
Post-processing methods are applied after model training. These methods adjust the model’s predictions to address issues like fairness and explainability. For instance, fairness-aware re-ranking can be used to adjust final predictions to ensure equal outcomes. To enhance explainability, model-agnostic approaches like SHAP and LIME can be used to provide clear explanations for model predictions.
Mitigation Tools and Technologies
Organizations can leverage various tools and platforms to streamline assessment and mitigation processes. IBM’s Cloud Pak for Data, for example, offers a comprehensive suite of AI governance capabilities, including model risk management, automated testing, fairness audits, and bias mitigation algorithms. IBM Research also provides advanced mitigation algorithms through early access programs, allowing companies to stay ahead of the curve in trustworthy AI innovation.
Conclusion
Trustworthy AI is the foundation of responsible AI adoption. By focusing on privacy, robustness, fairness, explainability, and transparency, organizations can establish trust in their AI systems, ensuring responsible innovation. Conducting comprehensive assessments at every stage of the AI lifecycle and using effective mitigation strategies allows businesses to manage risks, maintain compliance, and foster trust with customers, regulators, and stakeholders. With tools like IBM’s Cloud Pak for Data and advanced mitigation algorithms from IBM Research, enterprises can build AI systems that are not only powerful but also ethical, fair, and accountable.