Foundations of Trustworthy AI: Governed Data and AI, AI Ethics, and an Open, Diverse Ecosystem
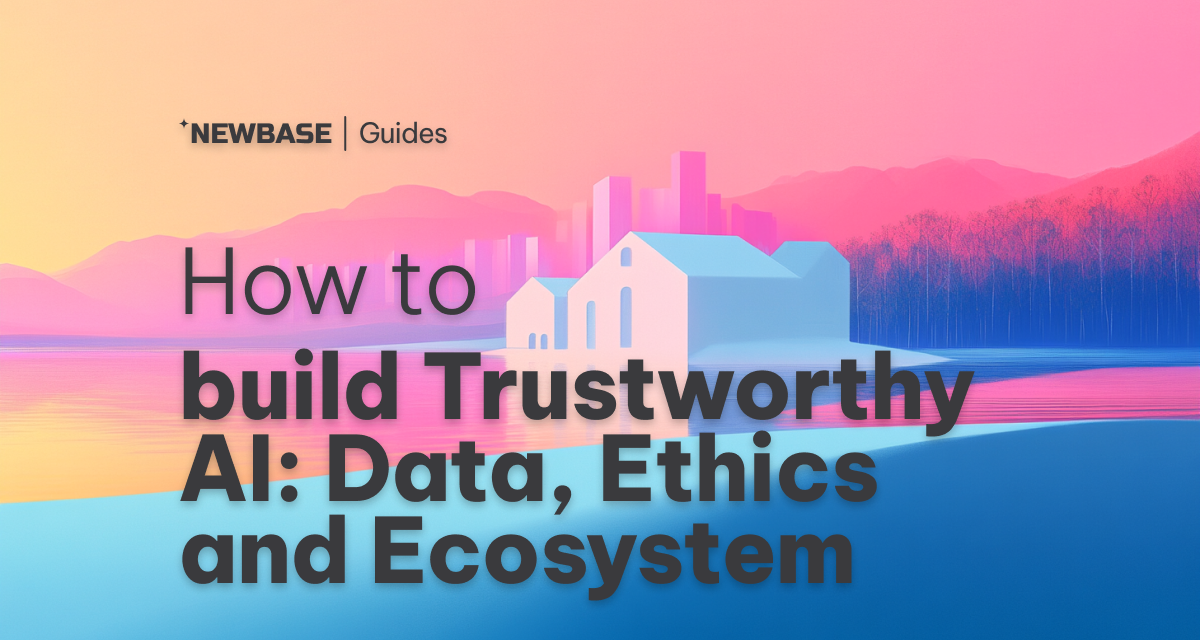
Artificial Intelligence (AI) is transforming industries, economies, and societies. However, as its influence grows, so does the need for transparency, accountability, and trust. Trustworthy AI is essential for widespread adoption, consumer confidence, and regulatory compliance. According to the Global AI Adoption Index 2021, 86% of global IT professionals agree that consumers are more likely to choose services from companies that offer transparency and an ethical framework for how data and AI models are built, managed, and used.
Building trust in AI requires a solid foundation rooted in governed data and AI, AI ethics, and an open, diverse ecosystem. These elements work together to ensure that AI systems are transparent, fair, robust, and ethical. Here’s a closer look at each of these pillars.
1. Governed Data and AI
Governed data and AI refers to the frameworks, tools, and processes that ensure AI solutions are built, deployed, and maintained in a manner that upholds trust. It focuses on establishing oversight to ensure AI operates as intended and complies with regulatory requirements. At its core, governed data and AI is structured around five key principles:
1.1. Transparency
Transparency is a cornerstone of trust. It involves the disclosure of how AI models work and the data used to train them. When users, stakeholders, and regulators can understand AI’s logic, they are more likely to trust its outputs. Transparency requires open access to information about the models, algorithms, and data used in AI decision-making processes. This also enables external audits and regulatory scrutiny, ensuring ethical standards are met.
1.2. Explainability
While transparency provides visibility into AI’s processes, explainability goes a step further by offering clear, human-understandable explanations of AI’s decisions. When AI systems influence critical decisions, such as hiring, lending, or medical diagnoses, it’s essential for users to understand how conclusions are reached. Explainability promotes accountability and enables users to challenge and verify AI-driven decisions, reducing the risk of “black box” AI.
1.3. Fairness
Bias in AI systems can perpetuate and even exacerbate societal inequalities. To achieve fairness, AI solutions must be designed to identify and reduce biases in datasets, algorithms, and decision-making processes. Fairness ensures equitable treatment of all individuals and groups, regardless of race, gender, or other protected characteristics. Continuous monitoring is crucial to maintain fairness, as biases can emerge over time or as new data is introduced.
1.4. Robustness
AI systems must be resilient against attacks and anomalies. As AI becomes more deeply embedded in daily life, it becomes a target for adversarial attacks. Robust AI can withstand threats, errors, and unexpected conditions while maintaining its integrity. This ensures that AI systems remain secure, stable, and functional in the face of evolving cyber threats and operational challenges.
1.5. Privacy
Data privacy is a non-negotiable aspect of trustworthy AI. AI systems process vast amounts of personal and sensitive information, and the protection of this data is paramount. Privacy-focused AI systems ensure that user data is anonymized, encrypted, and managed according to regulatory requirements. Beyond compliance, privacy principles strengthen user trust and enhance a company’s brand reputation.
2. AI Ethics
AI ethics serves as a moral compass for the development and use of AI technologies. As AI systems augment human decision-making and influence social behaviors, ethical considerations become essential. Ethical AI is guided by principles that prioritize human rights, fairness, and accountability.
2.1. Human-Centric Design
AI systems should be designed to augment human capabilities, not replace them. This approach ensures that AI acts as a complement to human decision-making, supporting and enhancing human judgment rather than overriding it. For example, in healthcare, AI can assist doctors in diagnosing diseases but should not make autonomous life-or-death decisions.
2.2. Data Ownership and Rights
Data is a critical component of AI development, but ethical AI maintains that data belongs to its human creators. Companies must respect data privacy rights and ensure users have control over their personal information. Ethical AI development prioritizes data protection and emphasizes user consent, giving people the right to know how their data is used.
2.3. Accountability and Responsibility
Accountability is a key tenet of ethical AI. Organizations must clearly define who is responsible for AI decisions and outcomes. If an AI-driven decision causes harm, it’s essential to determine responsibility—whether it lies with the developer, the organization, or the technology itself. Accountability frameworks ensure transparency in the decision-making process, mitigating risk and promoting public trust.
2.4. Transparency and Explainability
The ethical principles of transparency and explainability are closely tied to the technical principles mentioned earlier. Ethical AI should provide stakeholders with clear explanations of how AI makes decisions. This enables users, regulators, and society at large to understand and challenge AI’s conclusions. It’s especially crucial in high-stakes environments such as criminal justice, financial services, and healthcare.
3. Open and Diverse Ecosystem
While technology and ethics are critical, they must be supported by a culture of openness, diversity, and inclusion. A collaborative ecosystem fosters innovation and ensures AI systems are designed for all users, not just a select few.
3.1. Open Collaboration and Open-Source Innovation
An open ecosystem allows companies, developers, and researchers to work together, share knowledge, and accelerate the pace of AI innovation. Open-source AI frameworks encourage collaborative problem-solving and transparency. By allowing multiple stakeholders to contribute, open-source initiatives improve AI’s fairness, robustness, and security. Companies like IBM have adopted this approach by making AI models and datasets openly available, enabling diverse perspectives to shape AI development.
3.2. Diverse Teams
A diverse development team is essential for building inclusive AI solutions. Teams made up of people from varied cultural, racial, and gender backgrounds are better equipped to identify and mitigate biases in AI models. A diverse workforce brings unique perspectives, enhancing the cultural relevance and equity of AI solutions. Companies are encouraged to prioritize diversity in their hiring and team-building efforts to create AI that serves society as a whole.
3.3. Cross-Industry Partnerships
AI’s potential extends across industries, from healthcare to financial services. An open ecosystem facilitates cross-industry partnerships, enabling organizations to leverage shared expertise and co-develop AI solutions. Such partnerships not only foster innovation but also ensure that AI systems are robust, fair, and aligned with diverse stakeholder needs.
Conclusion
Trustworthy AI is built on a strong foundation of governed data and AI, ethical principles, and an open, diverse ecosystem. Together, these pillars establish transparency, fairness, robustness, and privacy—the core elements of trust. By prioritizing these principles, companies can build AI systems that inspire trust, reduce risk, and promote responsible innovation.
Organizations that embrace trustworthiness in AI position themselves as leaders in an era of rapid technological change. Companies that prioritize transparency, accountability, and diversity are better equipped to meet regulatory standards, reduce reputational risk, and build stronger customer relationships. By adopting a culture of openness and inclusivity, businesses can ensure that AI serves humanity ethically, equitably, and effectively.