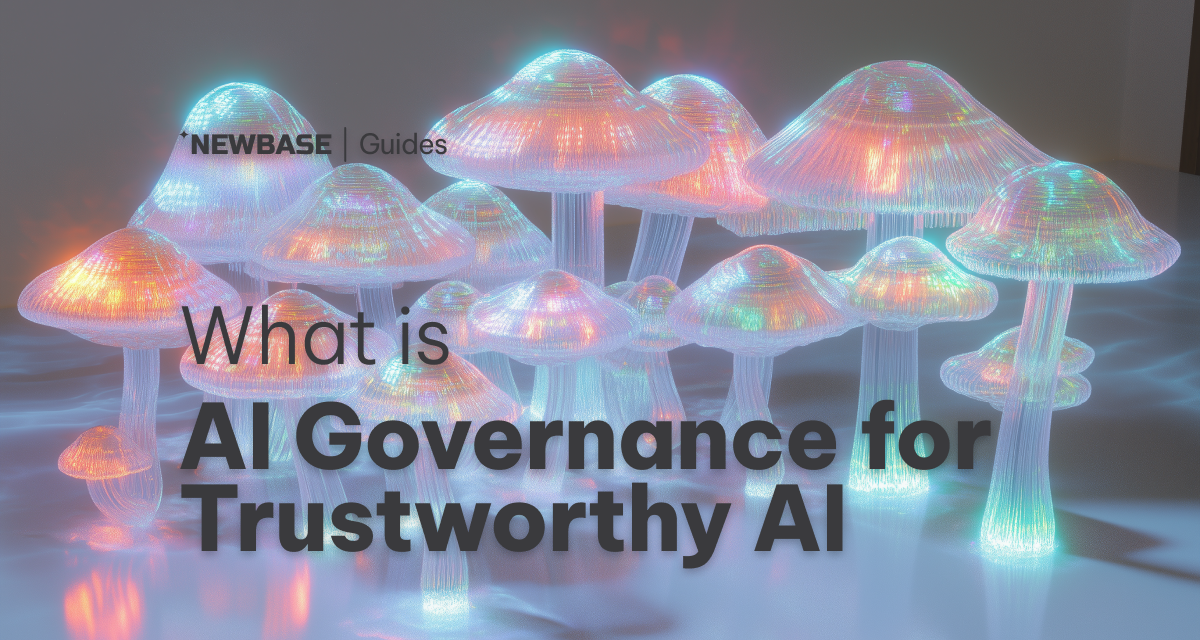
As organizations scale their use of artificial intelligence (AI), the importance of responsible and governed AI deployment becomes increasingly clear. Multiple forces drive the demand for AI governance, including brand reputation, regulatory compliance, AI complexity, and social justice. While any one of these factors alone would justify the need for governance, their convergence highlights AI governance as a critical capability for enterprises. Without a comprehensive approach to AI governance, AI initiatives risk failure due to unchecked risks and unpredictable outcomes.
What is AI Governance?
AI governance is the process of defining policies and establishing accountability to guide the creation and deployment of AI systems within an organization. Effective AI governance provides transparency into how AI systems are built and deployed, a key requirement for regulatory compliance. This transparency is achieved through the capture and management of metadata on AI models, which tracks their construction, deployment, and decision-making logic.
When executed correctly, AI governance does not hinder innovation; rather, it empowers organizations to operate with agility and confidence. By embedding governance into every stage of the AI lifecycle, businesses can trust AI-driven outcomes, accelerate time-to-market, and improve operational efficiency. From data profiling and policy enforcement to model development and risk management, AI governance ensures that organizations can achieve trustworthy and reliable AI outcomes.
Core Components of AI Governance
Achieving effective AI governance requires two fundamental elements:
- Organizational Structure: A well-defined structure provides governance leaders with the information they need to establish policies and accountability for AI development and deployment.
- Enhanced AI Lifecycle: This involves collecting and managing information across the AI lifecycle, enforcing governance policies, and making the information accessible to stakeholders in a clear and customizable manner.
Benefits of AI Governance
Enterprises that implement strong AI governance can realize several key benefits:
- Visibility and Documentation: Automated metadata capture throughout the AI lifecycle provides comprehensive documentation of development activities.
- Improved Outcomes and Efficiency: Analyzing metadata can reveal best practices, driving continuous improvement.
- Policy Consistency: Consistent policy enforcement ensures AI models meet regulatory, ethical, and business standards.
- Collaboration and Communication: Governance fosters collaboration among data scientists, AI engineers, developers, and other key stakeholders.
- Scalability: With a centralized view of AI development, organizations can build and scale AI initiatives with confidence and control.
The AI Governance Maturity Model
Organizations often progress through five levels of AI governance maturity as they advance their AI capabilities. Each level reflects an increasing degree of structure, oversight, and automation.
Level 0: No AI Lifecycle Governance
At this level, individual AI development teams operate in isolation, using their own tools and processes. There are no formalized, enterprise-wide policies or frameworks. This approach offers flexibility but creates significant risks if AI models are deployed to production. Without a framework, it becomes impossible to identify, measure, or mitigate AI-related risks. Companies at this level struggle to scale AI operations effectively.
Level 1: AI Policies in Place
Organizations at this stage have established formal AI policies at either a line-of-business or enterprise level. Policies guide AI development and deployment, and a common definition of required model information is established. However, without mechanisms for policy enforcement, individual AI teams often work in silos. This can lead to inconsistencies in AI implementation and heightened risk.
Level 2: Common Metrics for Governance
At level 2, organizations introduce a standardized set of metrics to measure AI model performance. A monitoring framework is implemented to track and compare model metrics across teams. This approach reduces risk, enhances transparency, and allows companies to make more informed policy decisions. Central model validation teams often enforce compliance with enterprise policies, driving measurable improvements in AI productivity.
Level 3: Enterprise Data and AI Catalog
This stage involves the creation of a unified enterprise data and AI catalog. By leveraging the metadata from level 2, organizations establish a comprehensive view of data, models, lifecycle metrics, and code pipelines. Full data lineage and traceability become possible, supporting compliance audits and risk assessments. This level offers a comprehensive view of AI risks and opportunities, helping organizations clearly articulate their AI strategy’s success.
Level 4: Automated Validation and Monitoring
Automation plays a central role at this level. Data capture, model validation, and monitoring processes are automated, reducing the burden on data scientists and lifecycle participants. This reduces operational overhead and minimizes the risk of human error. Automated validation also allows model validation teams to make decisions more quickly and efficiently. Companies at this level experience exponential productivity gains as they accelerate AI deployment cycles.
Level 5: Fully Automated AI Lifecycle Governance
The final stage represents the pinnacle of AI governance maturity. Automated governance mechanisms ensure that enterprise policies are consistently enforced across all AI models. Automated documentation of the AI lifecycle ensures transparency for regulators, auditors, and internal stakeholders. Risk assessment processes become more precise, and companies can focus manual intervention on high-risk areas. Organizations at this level achieve significant efficiency gains and maintain full confidence in their AI-driven strategies.
Industry Best Practices for AI Governance
To achieve and sustain a mature level of AI governance, organizations should adopt several best practices:
- Establish an AI Ethics Council: This council provides oversight on issues like privacy, fairness, and ethical AI use.
- Implement Automated AI FactSheets: Track and document every stage of AI model development to support compliance, accountability, and transparency.
- Foster Cross-Functional Collaboration: Enable collaboration among stakeholders, including legal, compliance, IT, and business units.
- Enhance Governance through AI Platforms: Leverage platforms like IBM’s Cloud Pak for Data to manage AI lifecycles and improve visibility.
Conclusion
AI governance is not just a regulatory requirement; it is a strategic enabler of trustworthy and efficient AI systems. Organizations that prioritize AI governance can scale AI with confidence, reduce risks, and achieve stronger business outcomes. By progressing through the AI governance maturity model, companies can move from isolated AI projects to a fully governed, enterprise-wide AI strategy. Organizations that act decisively on AI governance today will lead the future of responsible AI innovation.