Agentic AI: 4 Reasons Why It’s the Next Big Thing in AI Research
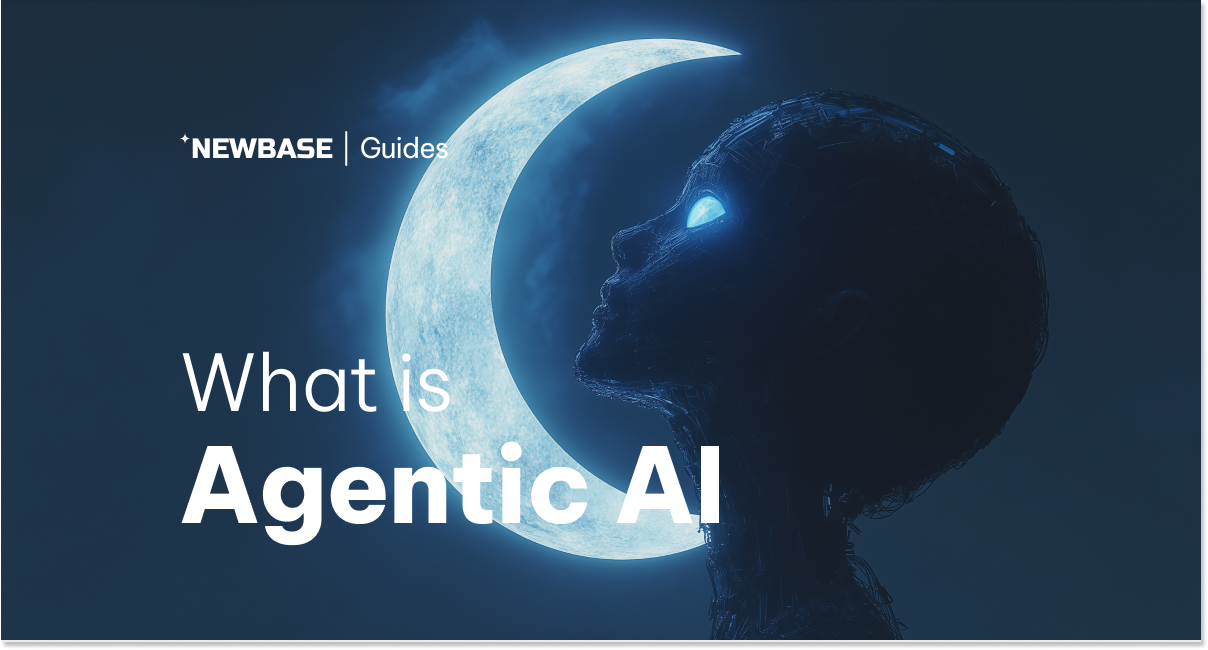
Agentic AI has recently become a major focus in artificial intelligence (AI) circles, and for good reason. While generative AI (gen AI) has dominated the spotlight over the past few years, agentic AI introduces a paradigm shift, blending the versatility of large language models (LLMs) with the precision of traditional programming. This transformative technology has the potential to redefine the way we interact with and deploy AI systems.
What is Agentic AI?
Agentic AI refers to AI systems capable of autonomously performing tasks on behalf of users or other systems by designing workflows and utilizing tools. These systems possess “agency”—the ability to make decisions, solve problems, and interact dynamically with external environments beyond their training data. Unlike static AI models, agentic AI systems adapt and learn from user behavior, improving their efficiency and scope over time. They can handle complex, multistep workflows that traditional AI models struggle with, making them indispensable for process automation and advanced problem-solving.
For example, a general-purpose LLM like ChatGPT might recommend an ice cream maker based on training data. However, an agentic AI system can take this further: it could access real-time e-commerce data, compare prices, and even complete the purchase on your behalf. By seamlessly integrating LLMs with actionable capabilities, agentic AI transforms abstract AI responses into real-world actions.
The excitement around agentic AI isn’t mere hype. Here are four reasons why it is poised to be the next big thing in AI research:
1. Combining Flexibility and Precision
LLMs excel at natural language processing (NLP), enabling users to interact with AI through intuitive commands. This flexibility allows LLMs to interpret nuanced instructions, generate creative content, and adapt to context-specific requirements. However, their generative nature can sometimes lack the precision required for deterministic tasks.
Traditional programming, on the other hand, is structured and reliable, making it ideal for tasks demanding high performance, repeatability, and strict logic. By combining these strengths, agentic AI creates a hybrid system that is both adaptive and precise.
An agentic AI platform might include:
- Simple Reflex Agents: Perform straightforward tasks consistently.
- Rule-Based Agents: Use memory to tackle more complex, multistep problems.
- Learning Agents: Continuously improve by ingesting new data and refining their decision-making processes.
For example, in an enterprise setting, an agentic AI system could integrate natural language interfaces for seamless user interactions while employing deterministic algorithms for critical functions such as security, compliance, and financial transactions. This synthesis makes agentic AI systems highly versatile, capable of addressing both dynamic and structured requirements.
2. Extended Reach
One of the limitations of LLMs is their reliance on static datasets. They cannot autonomously update their knowledge or access real-time data. Agentic AI overcomes this limitation by incorporating agents that can actively gather information from external sources, query databases, and interact with APIs.
Real-World Applications:
- Data Collection: Agents can fetch real-time data, such as stock prices, weather updates, or IoT sensor readings, and feed it into an LLM for contextual analysis.
- Monitoring and Feedback: Agents can monitor social media trends, business operations, or supply chain performance, providing timely insights.
- Adaptability: Feedback loops enable agents to refine their processes over time, ensuring continuous optimization.
For instance, in the retail sector, an agentic AI system could track inventory levels, analyze sales patterns, and autonomously reorder stock. By continuously integrating fresh data, these systems maintain relevance and deliver actionable insights.
3. Autonomous Operation
Agentic AI systems are designed for autonomy, enabling them to operate independently without constant human oversight. This capability allows them to handle long-term goals, manage complex workflows, and adapt to evolving conditions.
Industry Examples:
- Marketing: An agentic AI could manage an entire marketing campaign—from audience targeting and content creation to performance tracking and strategy adjustment—without human intervention.
- Healthcare: Agents can monitor patient data, suggest treatment adjustments based on test results, and provide clinicians with actionable insights.
- Cybersecurity: AI agents can autonomously detect and respond to threats, such as phishing attempts or malware, in real time.
- Supply Chain Management: By analyzing demand forecasts and production schedules, agentic AI can autonomously optimize inventory levels and logistics.
This autonomy reduces the need for manual intervention in repetitive tasks, freeing up human workers to focus on strategic, high-value activities.
4. Intuitive User Experience
One of the most compelling features of agentic AI is its ability to simplify complex systems through natural language interfaces. Instead of navigating through complicated software interfaces, users can interact with agentic AI systems using plain language, making advanced functionalities accessible to non-technical users.
Imagine This:
A project manager asks an agentic AI to generate a presentation showing team performance metrics for the past six months. Instead of manually extracting and analyzing data from multiple sources, the AI:
- Gathers relevant data from various tools.
- Analyzes trends and highlights key insights.
- Creates polished slides with charts and graphs—all within seconds.
This level of convenience not only boosts productivity but also democratizes access to powerful AI tools, enabling organizations to derive value more efficiently.
The Road Ahead for Agentic AI
As organizations strive to maximize the potential of AI, the limitations of standalone LLMs are becoming increasingly evident. While generative AI has captured public imagination, its practical applications often fall short in enterprise contexts. Agentic AI bridges this gap by integrating LLMs with actionable capabilities, providing a scalable framework for real-world use cases.
Agentic AI represents the future of AI research, where systems are not just intelligent but also capable of meaningful action. By combining flexibility, precision, extended reach, autonomy, and intuitive usability, it paves the way for transformative innovations across industries. As investments and research in this area grow, agentic AI is set to redefine what’s possible in AI-driven automation and decision-making.