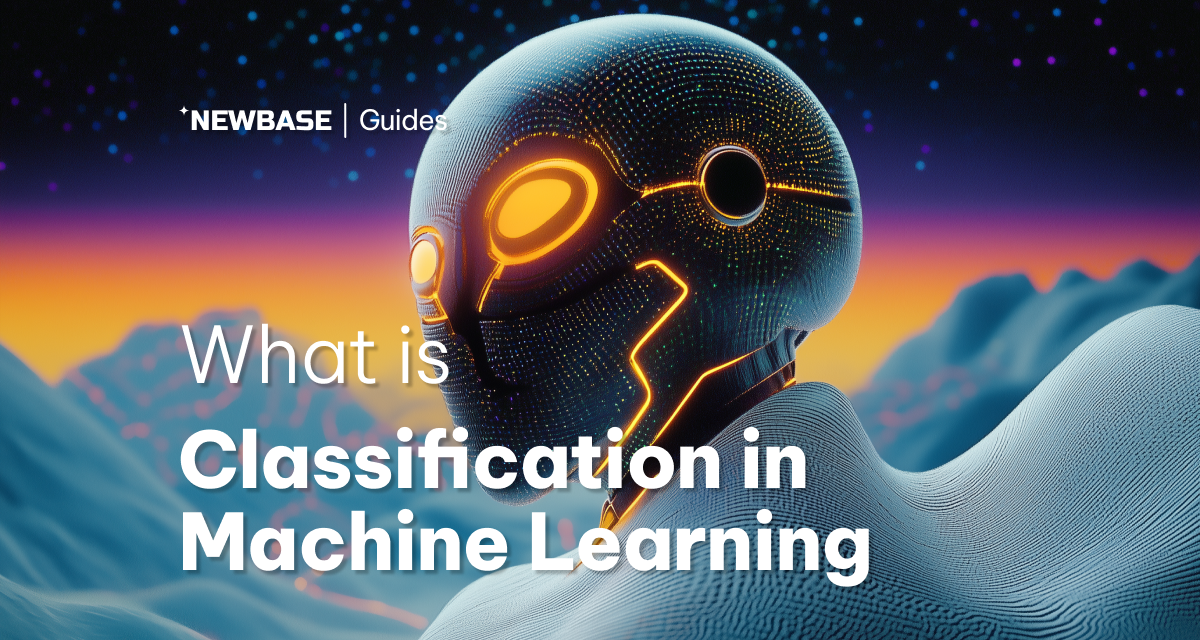
Classification, a cornerstone of machine learning, is a supervised learning technique that involves assigning data points to predefined categories or classes. It’s akin to sorting objects into boxes based on their characteristics.
Key Components of Classification:
- Features: These are the attributes or characteristics of a data point that are used to make predictions.
- Labels: The predefined categories or classes that data points are assigned to.
- Model: The mathematical function that learns the relationship between features and labels.
- Training Data: A dataset of labeled examples used to train the model.
- Testing Data: A separate dataset used to evaluate the model’s performance.
The Classification Process
- Data Preparation:
- Data Cleaning: Handling missing values, outliers, and inconsistencies.
- Feature Engineering: Creating new features or transforming existing ones to improve model performance.
- Data Splitting: Dividing the dataset into training and testing sets.
- Model Training:
- Model Evaluation:
- Performance Metrics: Using metrics like accuracy, precision, recall, F1-score, and confusion matrices to assess the model’s performance.
- Model Selection: Choosing the best-performing model based on the evaluation metrics.
Types of Classification Problems
- Binary Classification: Classifying data into two categories (e.g., spam or not spam, positive or negative).
- Multi-class Classification: Classifying data into more than two categories (e.g., different types of fruits).
- Multi-label Classification: Assigning multiple labels to a single data point (e.g., a news article can be classified as “politics,” “technology,” and “environment”).
Popular Classification Algorithms
- Logistic Regression: A statistical model used to predict the probability of a binary outcome.
- Decision Trees: A tree-like model of decisions and their possible consequences, used to classify data.
- Random Forest: An ensemble method that combines multiple decision trees to improve accuracy.
- Support Vector Machines (SVM): A powerful algorithm that finds the optimal hyperplane to separate data points.
- Naive Bayes: A probabilistic classifier based on Bayes’ theorem.
- K-Nearest Neighbors (KNN): A simple algorithm that classifies data points based on their nearest neighbors.
Advanced Techniques
- Ensemble Methods: Combining multiple models to improve performance (e.g., bagging, boosting).
- Deep Learning: Using neural networks with multiple layers to learn complex patterns.
- Transfer Learning: Leveraging pre-trained models on large datasets to improve performance on smaller datasets.
Challenges and Considerations
- Imbalanced Datasets: Handling datasets with unequal class distributions.
- Overfitting: Preventing the model from memorizing the training data too closely.
- Underfitting: Ensuring the model is complex enough to capture the underlying patterns.
- Feature Engineering: Selecting and transforming relevant features.
- Model Selection: Choosing the right algorithm for the specific problem.
- Hyperparameter Tuning: Optimizing the model’s hyperparameters.
Real-World Applications
- Image Recognition: Classifying images of objects, scenes, and faces.
- Natural Language Processing (NLP): Sentiment analysis, text classification, and machine translation.
- Medical Diagnosis: Predicting diseases based on medical records.
- Fraud Detection: Identifying fraudulent transactions.
- Recommendation Systems: Suggesting products or content to users.
By understanding the principles and techniques of classification, you can harness the power of machine learning to solve a wide range of real-world problems.
Related articles
No comments yet...