What is ChatDev? Understanding ChatDev: A Multiagent Framework for Collaborative AI-Driven Software Development
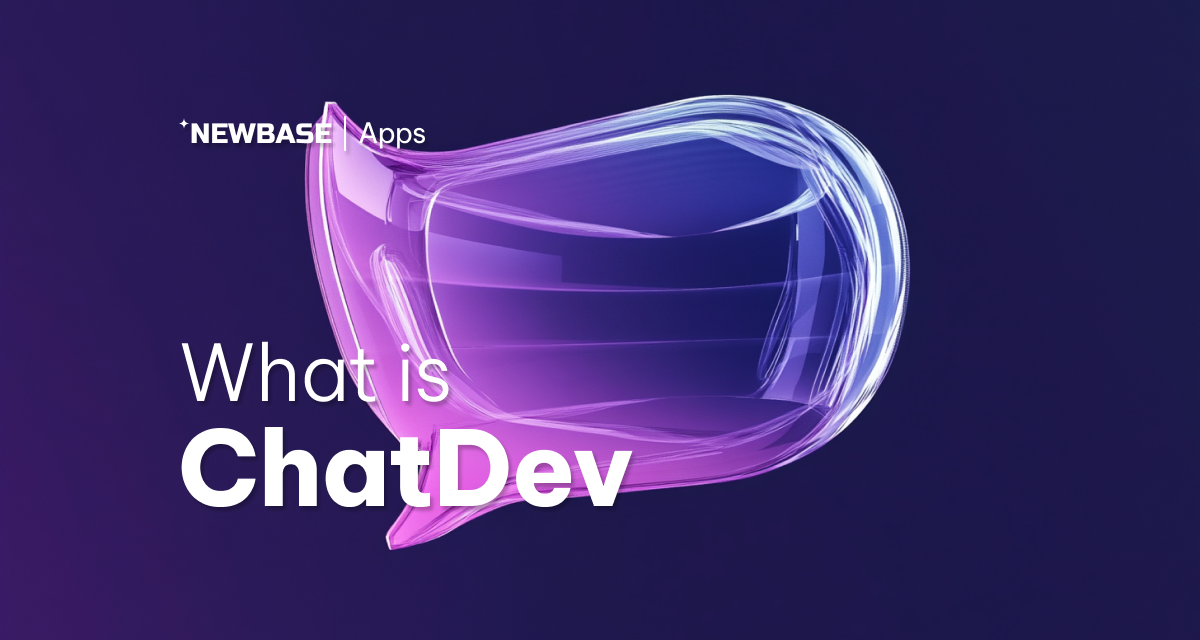
ChatDev is an open-source, agent-based framework designed to facilitate multiagent collaboration through a team of specialized intelligent agents powered by large language models (LLMs). The system operates like a virtual software company, where each agent is assigned specific roles, working collaboratively to complete complex tasks within the software development lifecycle. This collaborative process empowers agents to autonomously generate software applications by performing tasks traditionally associated with the stages of software development, such as design, coding, testing, and documentation. The framework combines the power of LLMs with sophisticated orchestration techniques, allowing for seamless cooperation and efficient task completion.
At its core, ChatDev aims to offer a customizable and scalable LLM orchestration framework, providing a real-world setting to study and explore collective intelligence within multiagent systems (MAS). It provides an ideal environment for understanding how multiple agents, each specialized in different tasks, can work together effectively to outperform individual agents in solving complex problems.
Collective Intelligence and Multiagent Systems (MAS)
The concept of collective intelligence refers to the ability of a group of agents or entities to work together to solve problems more effectively than any single agent could on its own. Collective intelligence is a cornerstone of artificial intelligence (AI) research, particularly in the design of multiagent systems (MAS). These systems comprise multiple interconnected agents that collaborate and communicate to tackle tasks that are too complex for a single agent to address.
In the case of ChatDev, collective intelligence is applied within the context of the software development lifecycle. Each agent specializes in a specific aspect of the development process (e.g., software design, coding, testing), and by working together, these agents can complete the entire development process autonomously. The application of collective intelligence in MAS requires effective communication and collaboration between agents, as well as the coordination of their actions to ensure a cohesive solution is achieved.
Cooperative Multiagent Interactions in ChatDev
One of the challenges in designing a functional multiagent system is ensuring that agents collaborate effectively on a global task. In MAS, the coordination of agents involves understanding how the actions of one agent can impact the actions of others. This coordination is crucial in ensuring that agents do not conflict with each other but instead work together to solve a problem. In ChatDev, the agents’ interactions are governed by a series of well-defined workflows and communication protocols.
To streamline the software development process, ChatDev employs a “multi-collaborative” design pattern. This pattern involves breaking down a complex task (such as developing software) into smaller, more manageable subtasks, which are then delegated to specialized agents. For example, during the coding phase, a code reviewer agent may collaborate with a GUI (graphical user interface) programmer agent, with the two agents working together to produce the necessary code.
The core mechanism that enables this cooperative communication between agents is called ChatChain, a dual-agent communication design. ChatChain structures the communication into a series of phases, where two agents with distinct roles interact to complete specific tasks. This system of communication allows the agents to break down tasks into manageable steps, ensuring that each agent focuses on their area of expertise while still contributing to the overall solution.
ChatDev’s Agentic Workflow: ChatChain
ChatDev’s architecture relies on ChatChain, which divides the software development process into three main phases: design, coding, and testing. Within these phases, ChatChain further segments tasks into more granular subtasks such as demand analysis, language selection, code writing, static testing, dynamic testing, and documentation.
Each phase of ChatChain is governed by two agents: an instructor agent and an assistant agent. The instructor agent is responsible for guiding the subtask toward completion by providing instructions and setting goals, while the assistant agent follows these instructions and responds with solutions. The agents engage in multi-turn dialogues, iterating on their solutions until consensus is reached or the task is completed.
This dual-agent design simplifies the communication process by creating a clear structure that avoids the complexities often associated with larger multiagent topologies. By keeping the communication between two agents in each subtask, ChatDev ensures that the agents stay focused on the task at hand, reducing distractions and inefficiencies. Once a subtask is completed, the solution is passed to the next phase, creating a seamless transition between the stages of development.
For instance, during the coding phase, one agent might be tasked with writing the initial code, while another agent reviews the code for functionality and consistency. This system allows for the continuous refinement of the software as it progresses through the stages of development.
Inception Prompting and Role-Playing
One of the key features that enables effective multiagent collaboration in ChatDev is inception prompting. This technique helps agents stay focused on their roles and ensures that they remain aligned with the task requirements. Inception prompting is a form of auto-prompting that encourages agents to engage in role-playing. Each agent is prompted to behave in a way that reflects their specific role within the system, guiding them to provide appropriate responses.
For example, if an assistant agent struggles to comply with instructions due to ambiguity or a lack of information, the agent may ask for clarification from the instructor before proceeding. This iterative prompting process helps prevent misunderstandings and ensures that agents remain on track.
Additionally, ChatDev employs a communicative dehallucination mechanism to address the issue of LLM “hallucinations,” or inaccurate and nonsensical outputs. This mechanism ensures that agents request more detailed information from the instructor before delivering a response, thereby reducing the likelihood of errors. By guiding agents through a deliberate process of information exchange, ChatDev can maintain high-quality output, even when agents encounter complex or vague tasks.
ChatDev’s Visualizer and Debugging Tools
To facilitate understanding of the interactions between agents and to help developers troubleshoot the multiagent system, ChatDev provides a Visualizer. The Visualizer is a Python-based web application that allows users to view real-time logs, replay logs, and the ChatChain workflow. The Visualizer offers three distinct views: the Log Visualizer, which shows real-time agent dialogues and system changes; the Replay Visualizer, which replays past agent interactions; and the ChatChain Visualizer, which allows users to upload and examine the agentic workflow for any software project.
By using the Visualizer, developers can gain insights into the interactions between agents, identify bottlenecks, and improve the overall efficiency of the system. This transparent view of the development process is a powerful tool for studying and refining the collaborative capabilities of agents.
Scaling ChatDev with MacNet
As multiagent collaboration in ChatDev grows, scalability becomes a key consideration. MacNet (Multiagent Collaboration Network) is a framework within ChatDev designed to scale multiagent systems. Drawing inspiration from the neural scaling law, which suggests that increasing the number of neurons in a neural network leads to emergent abilities, MacNet applies a similar principle to multiagent collaboration. By increasing the number of agents in the system, ChatDev can handle increasingly complex tasks more effectively.
MacNet organizes agents using acyclic graphs and enhances their reasoning abilities through topological ordering. As more agents are added to the network, the system becomes capable of solving more complex problems and delivering higher-quality solutions. This collaborative scaling law demonstrates that increasing the number of agents in the system results in a logistic improvement in the quality of solutions.
ChatDev’s Performance Compared to Other Frameworks
When compared to other multiagent frameworks like GPT-Engineer and MetaGPT, ChatDev has been shown to outperform these systems in key areas such as quality and cooperative communication. While GPT-Engineer relies on a single-agent orchestration model, ChatDev divides tasks among multiple specialized agents, allowing for more efficient problem-solving and higher-quality outputs. This multiagent approach provides a more versatile and adaptable system, capable of handling complex software development tasks with greater precision.
MetaGPT, another multiagent framework, shares similarities with ChatDev in its role-playing agent structure, but ChatDev distinguishes itself with more advanced communication techniques and the ability to handle a broader range of tasks.
Conclusion
ChatDev represents a cutting-edge advancement in the field of multiagent systems, combining large language models with specialized agents to tackle complex software development tasks. By breaking down the software development process into discrete phases and leveraging the power of multiagent collaboration, ChatDev enables agents to autonomously generate software while maintaining high standards of quality and efficiency. Through its innovative design and orchestration techniques, ChatDev provides valuable insights into collective intelligence and serves as a model for the future of AI-driven software development.